Uncertainty-Aware Weakly Supervised Action Detection from Untrimmed Videos
European Conference on Computer Vision(2020)
摘要
Despite the recent advances in video classification, progress in spatio-temporal action recognition has lagged behind. A major contributing factor has been the prohibitive cost of annotating videos frame-by-frame. In this paper, we present a spatio-temporal action recognition model that is trained with only video-level labels, which are significantly easier to annotate. Our method leverages per-frame person detectors which have been trained on large image datasets within a Multiple Instance Learning framework. We show how we can apply our method in cases where the standard Multiple Instance Learning assumption, that each bag contains at least one instance with the specified label, is invalid using a novel probabilistic variant of MIL where we estimate the uncertainty of each prediction. Furthermore, we report the first weakly-supervised results on the AVA dataset and state-of-the-art results among weakly-supervised methods on UCF101-24.
更多查看译文
关键词
Spatio-temporal action recognition, Weak supervision, Video understanding, Mulitple Instance Learning
AI 理解论文
溯源树
样例
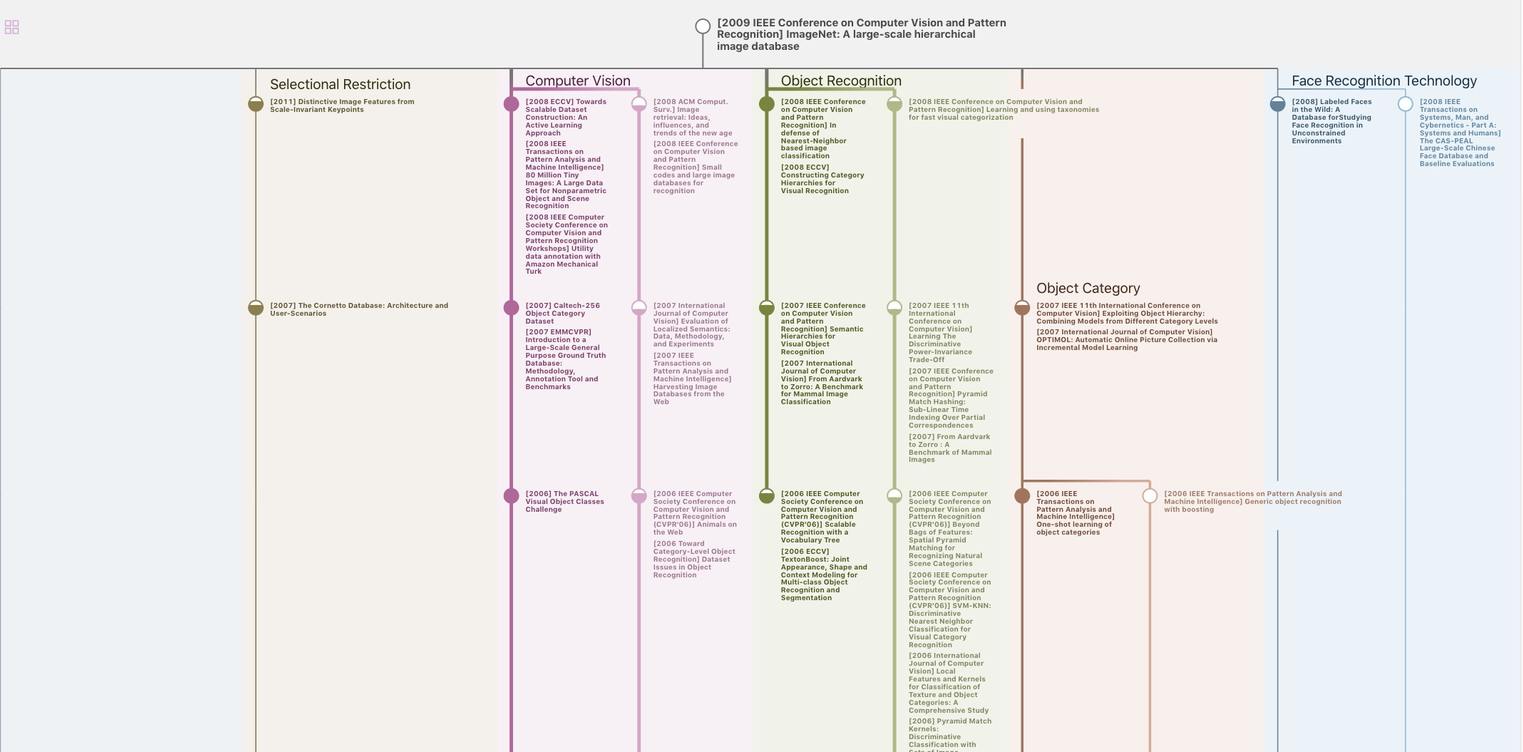
生成溯源树,研究论文发展脉络
Chat Paper
正在生成论文摘要