Navigating the Trade-Off between Multi-Task Learning and Learning to Multitask in Deep Neural Networks
arxiv(2020)
摘要
The terms multi-task learning and multitasking are easily confused. Multi-task learning refers to a paradigm in machine learning in which a network is trained on various related tasks to facilitate the acquisition of tasks. In contrast, multitasking is used to indicate, especially in the cognitive science literature, the ability to execute multiple tasks simultaneously. While multi-task learning exploits the discovery of common structure between tasks in the form of shared representations, multitasking is promoted by separating representations between tasks to avoid processing interference. Here, we build on previous work involving shallow networks and simple task settings suggesting that there is a trade-off between multi-task learning and multitasking, mediated by the use of shared versus separated representations. We show that the same tension arises in deep networks and discuss a meta-learning algorithm for an agent to manage this trade-off in an unfamiliar environment. We display through different experiments that the agent is able to successfully optimize its training strategy as a function of the environment.
更多查看译文
关键词
multitask,deep neural networks,learning,neural networks,trade-off,multi-task
AI 理解论文
溯源树
样例
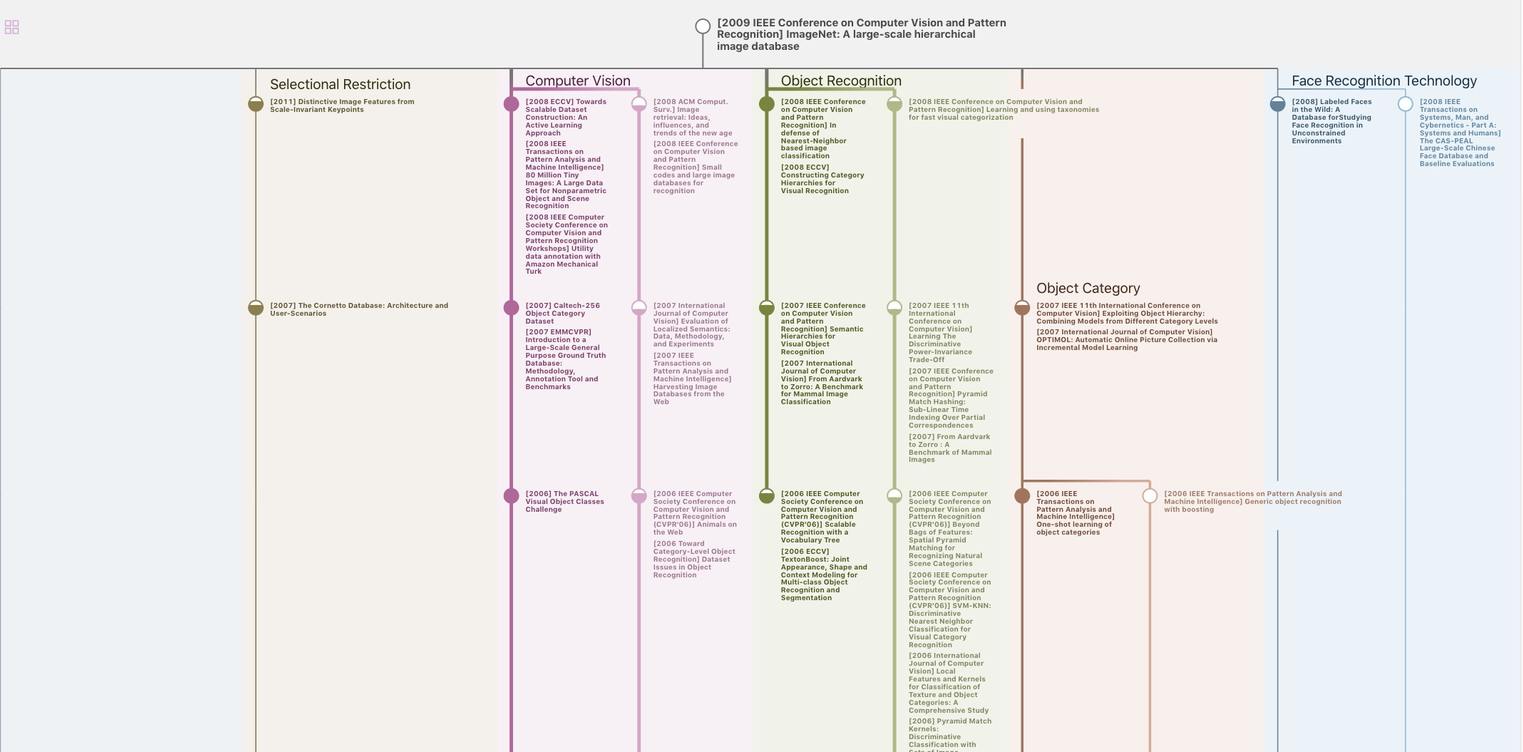
生成溯源树,研究论文发展脉络
Chat Paper
正在生成论文摘要