Improving Attention-Based Handwritten Mathematical Expression Recognition with Scale Augmentation and Drop Attention
2020 17th International Conference on Frontiers in Handwriting Recognition (ICFHR)(2020)
摘要
Handwritten mathematical expression recognition (HMER) is an important research direction in handwriting recognition. The performance of HMER suffers from the two-dimensional structure of mathematical expressions (MEs). To address this issue, in this paper, we propose a high-performance HMER model with scale augmentation and drop attention. Specifically, tackling ME with unstable scale in both horizontal and vertical directions, scale augmentation improves the performance of the model on MEs of various scales. An attention-based encoder-decoder network is used for extracting features and generating predictions. In addition, drop attention is proposed to further improve performance when the attention distribution of the decoder is not precise. Compared with previous methods, our method achieves state-of-the-art performance on two public datasets of CROHME 2014 and CROHME 2016.
更多查看译文
关键词
handwritten mathematical expression recognition,data augmentation,encoder-decoder network,attention mechanism
AI 理解论文
溯源树
样例
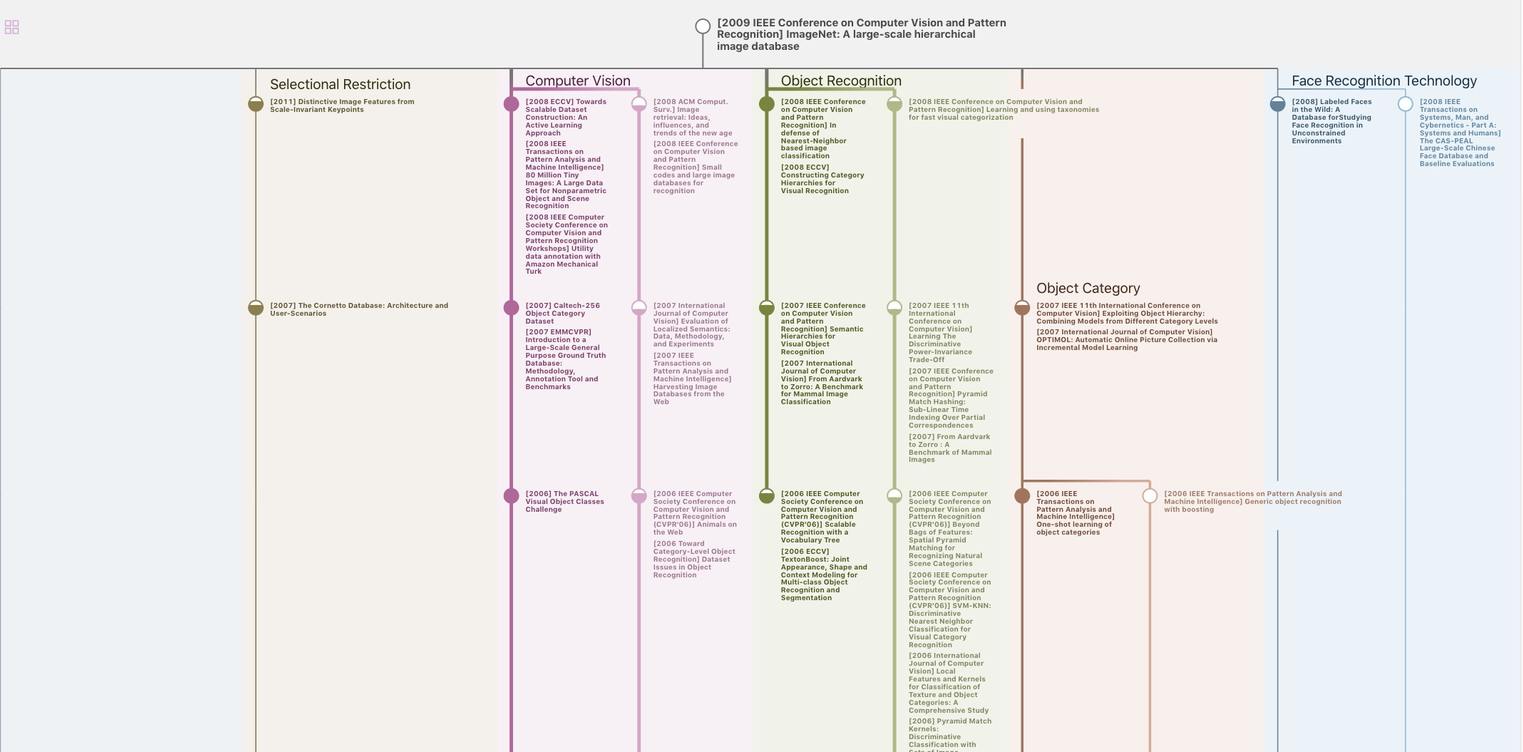
生成溯源树,研究论文发展脉络
Chat Paper
正在生成论文摘要