Reinforcement Learning-Enabled Decision-Making Strategies for a Vehicle-Cyber-Physical-System in Connected Environment
CoRR(2020)
摘要
As a typical vehicle-cyber-physical-system (V-CPS), connected automated vehicles attracted more and more attention in recent years. This paper focuses on discussing the decision-making (DM) strategy for autonomous vehicles in a connected environment. First, the highway DM problem is formulated, wherein the vehicles can exchange information via wireless networking. Then, two classical reinforcement learning (RL) algorithms, Q-learning and Dyna, are leveraged to derive the DM strategies in a predefined driving scenario. Finally, the control performance of the derived DM policies in safety and efficiency is analyzed. Furthermore, the inherent differences of the RL algorithms are embodied and discussed in DM strategies.
更多查看译文
关键词
reinforcement,connected environment,decision-making decision-making,learning-enabled,vehicle-cyber-physical-system
AI 理解论文
溯源树
样例
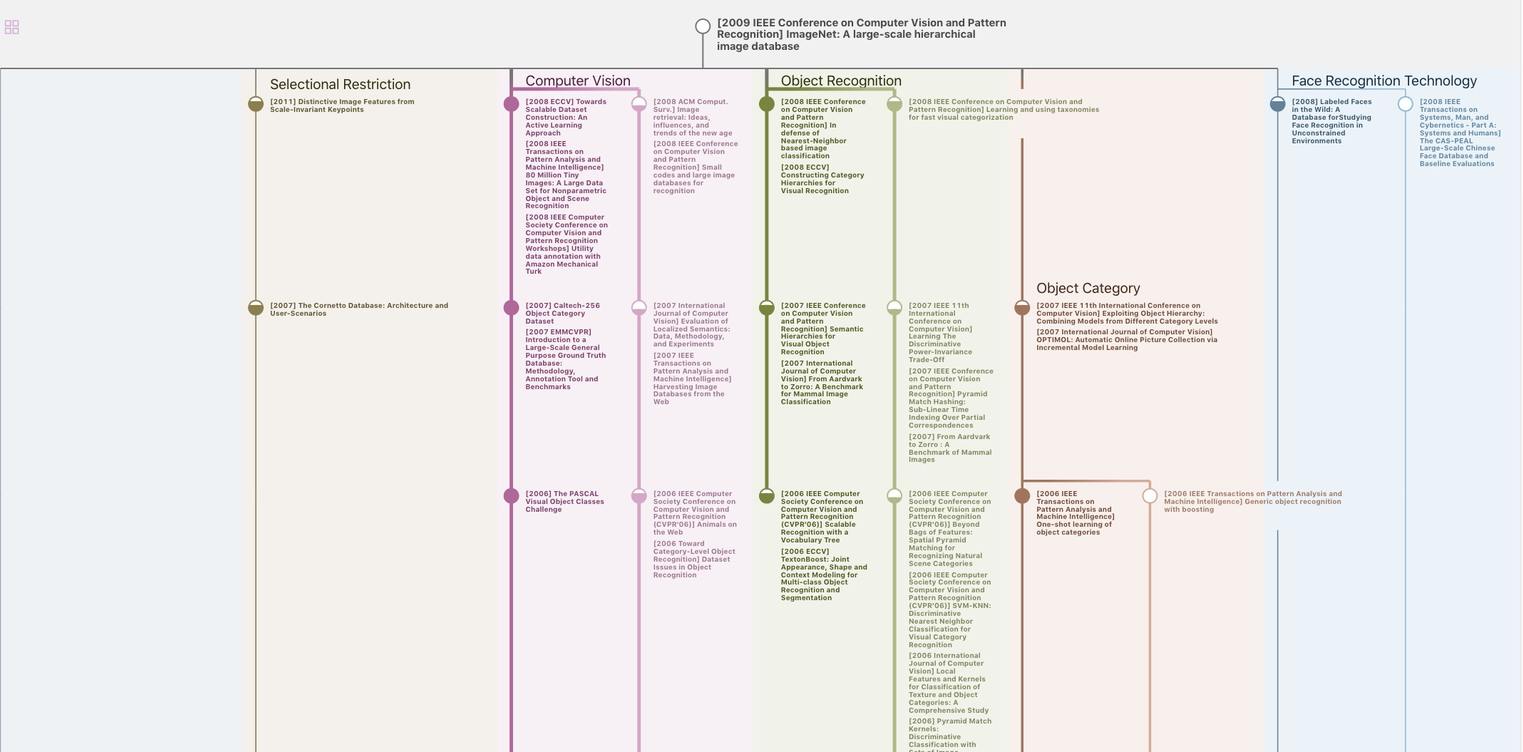
生成溯源树,研究论文发展脉络
Chat Paper
正在生成论文摘要