Combining Hyperspectral Imaging and Feature Wavelength Extraction Methods for the Rapid Discrimination of Red Meat
Journal of Applied Spectroscopy(2020)
摘要
hyperspectral imaging system (400–800 nm) combined with multivariate analyses was investigated to discriminate between beef, pork, and mutton species based on the feature wavelengths of intact and minced samples. The performances of classification models constructed by combining linear discriminant analysis (LDA), partial least squares discriminant analysis (PLS–DA), or a support vector machine (SVM) with a variable selection method, such as a successive projection algorithm (SPA), regression coefficient analysis (RCA), or random frog (RF), were compared. The results clearly showed that the linear classifier was preferred to the nonlinear classifier in the identification of red meat species. Furthermore, instead of selecting different sets of feature wavelengths for different types meat samples, only a set of optimum wavelengths including five wavebands (567, 579, 595, 624, and 732 nm) were identified as universal feature wavelengths by a comprehensive comparison of three schemes, namely, variable fusion, a merging, and cross modeling. A simplified LDA model was then established based on these important wavelengths, yielding classification accuracies of 94.20 and 98.36% in the validation set for the intact meat and minced samples, respectively. The overall results showed that the integration of hyperspectral imaging and multivariate analyses has great potential for rapid and nondestructive differentiation of common red meat species.
更多查看译文
关键词
hyperspectral imaging, red meat, variable selection, feature fusion
AI 理解论文
溯源树
样例
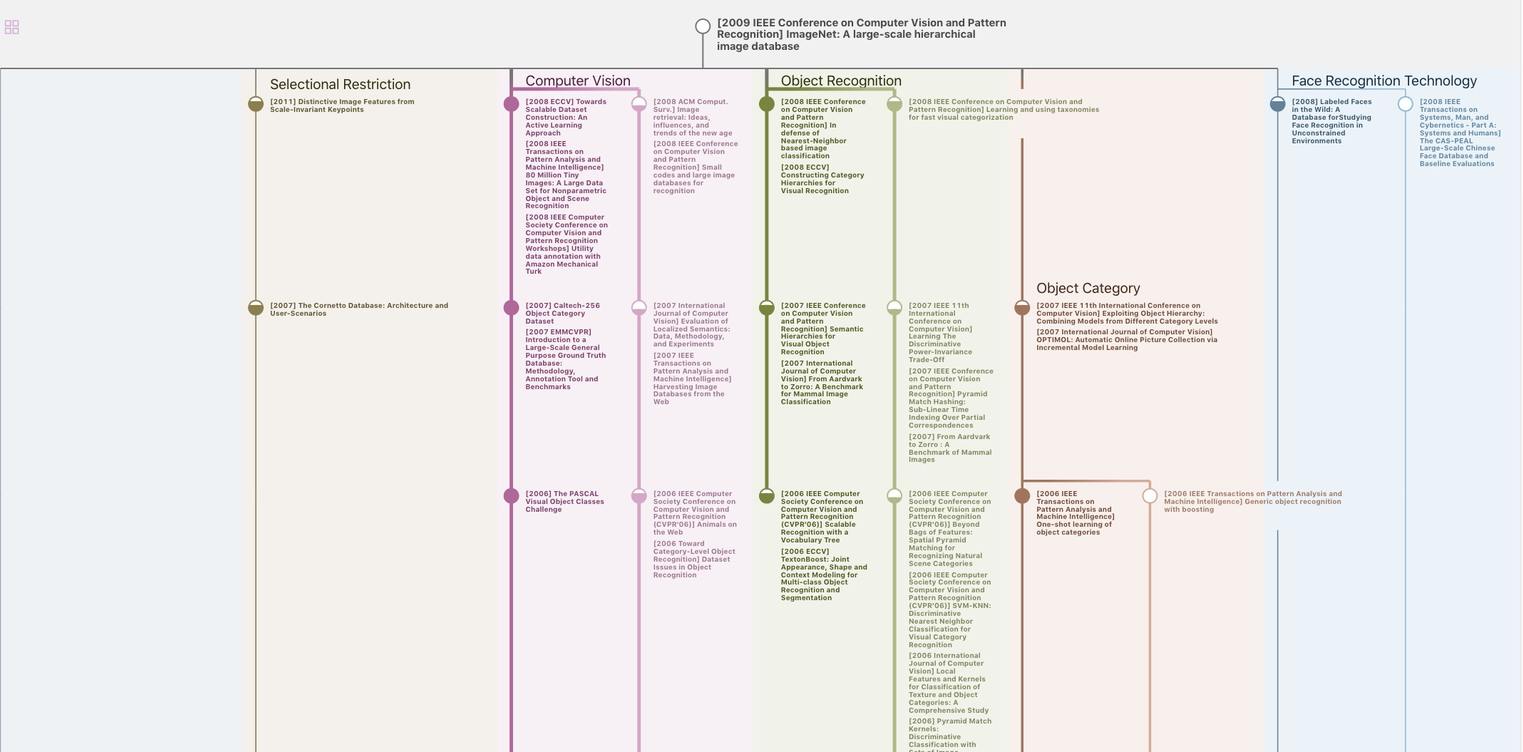
生成溯源树,研究论文发展脉络
Chat Paper
正在生成论文摘要