Extending LINE for Network Embedding With Completely Imbalanced Labels
Periodicals(2020)
摘要
AbstractNetwork embedding is a fundamental problem in network research. Semi-supervised network embedding, which benefits from labeled data, has recently attracted considerable interest. However, existing semi-supervised methods would get biased results in the completely-imbalanced label setting where labeled data cannot cover all classes. This article proposes a novel network embedding method which could benefit from completely-imbalanced labels by approximately guaranteeing both intra-class similarity and inter-class dissimilarity. In addition, the authors prove and adopt the matrix factorization form of LINE (a famous network embedding method) as the network structure preserving model. Extensive experiments demonstrate the superiority and robustness of this method.
更多查看译文
关键词
Imbalance Data Learning, Network Embedding, Social Networks
AI 理解论文
溯源树
样例
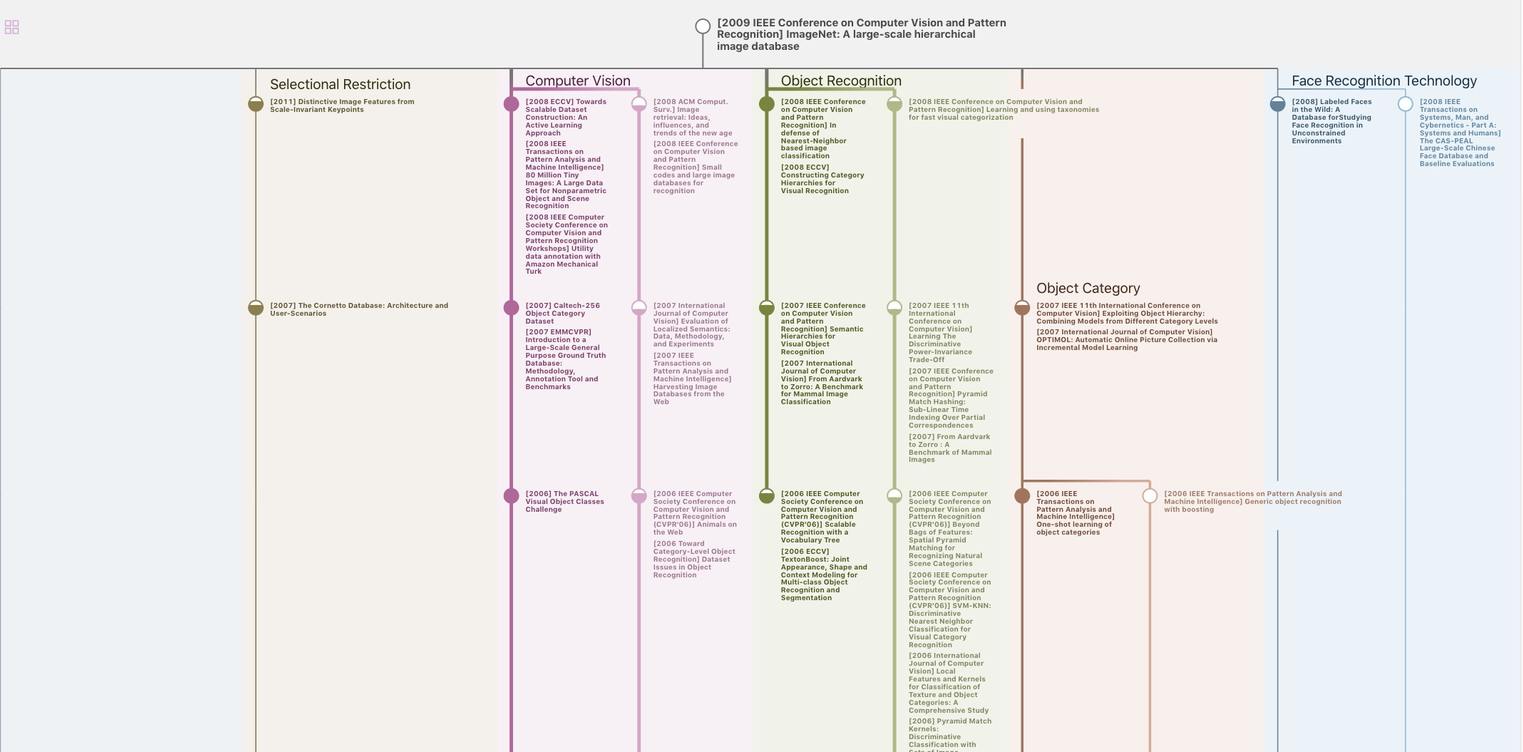
生成溯源树,研究论文发展脉络
Chat Paper
正在生成论文摘要