A Novel Approach Of Tensor-Based Data Missing Estimation For Internet Of Vehicles
INTERNATIONAL JOURNAL OF COMMUNICATION SYSTEMS(2020)
摘要
In the face of the current huge amount of intelligent traffic data, collecting and statistical processing is a necessary and important process. But the inevitable data missing problem is the current research focus. In this paper, a novel approach of tensor-based data missing estimation for Internet of Vehicles is proposed for the problem of missing the Internet of Vehicles data: Integrated Bayesian tensor decomposition (IBTD). In the data model construction stage, the random sampling principle is used to randomly extract the missing data to generate a subset of data. And the optimized Bayesian tensor decomposition algorithm is used for interpolation. Introduce the integration idea, analyze, and sort the error results after multiple interpolations, consider the space-time complexity, and choose the optimal average to get the best result. The performance of the proposed model was evaluated by mean absolute percentage error (MAPE) and root mean square error (RMSE). The experimental results show that the proposed method can effectively interpolate the traffic data sets with different missing quantities and get good interpolation results.
更多查看译文
关键词
Bayesian tensor decomposition, data missing, Internet of Vehicles, random sampling, tensor
AI 理解论文
溯源树
样例
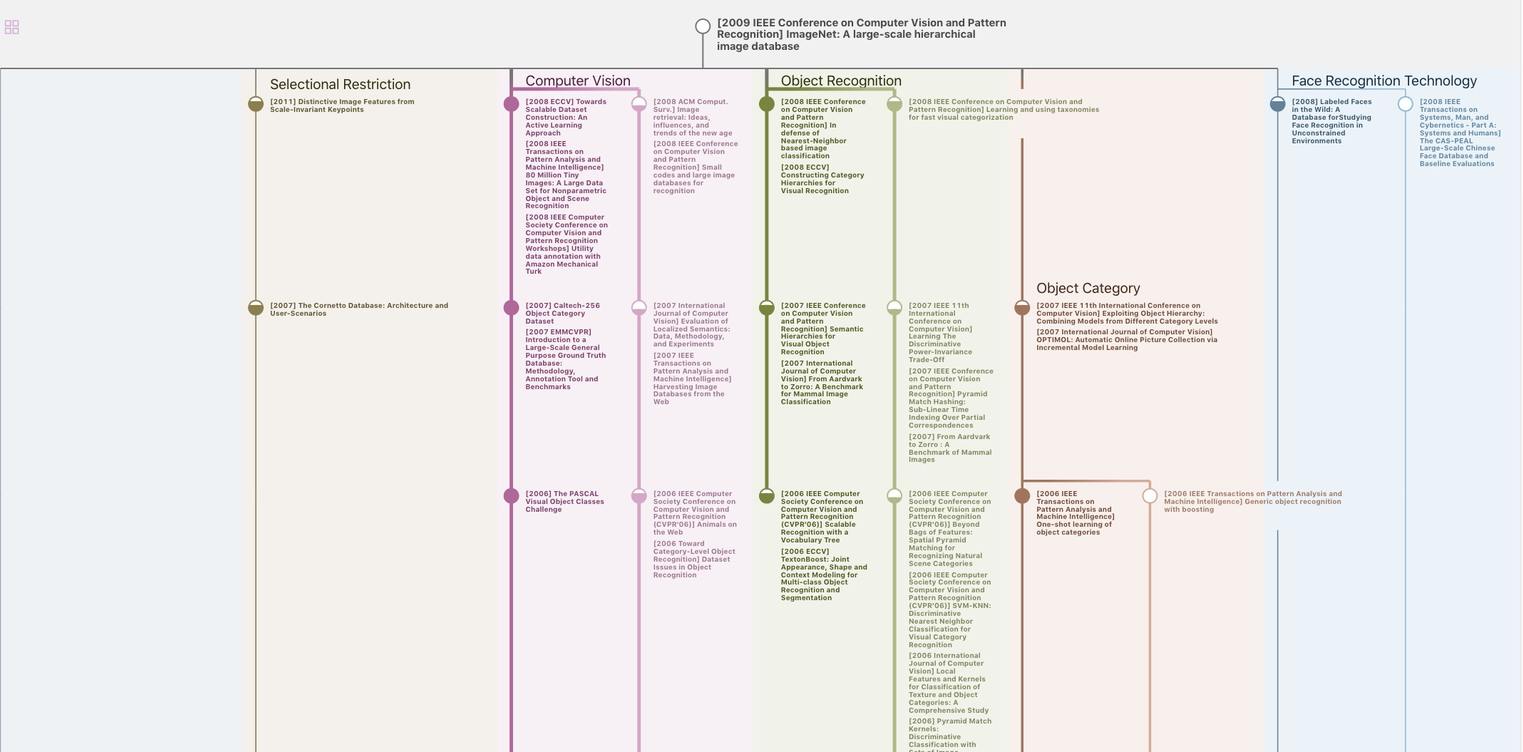
生成溯源树,研究论文发展脉络
Chat Paper
正在生成论文摘要