Adding Uncertainty To Dermatological Assistance
MEDICAL IMAGING 2020: IMAGE PROCESSING(2021)
摘要
Medical advancements have been improving the quality of life, but are experiencing an exponential increase in information. Navigating this vast knowledge base is becoming increasingly difficult for doctors without the help of diagnostic assistance tools. Many theorize that advances in deep learning may be poised to transform the field of dermatology, either by helping a general practitioner make a simple dermatological diagnosis or by helping an experienced dermatologist better understand rare or difficult to diagnose skin conditions. While deep learning technology has shown remarkable success in many fields, it still frequently suffers from difficult to explain failures on what appear to be simple input stimuli. Further, these models are forced to make a categorical classification prediction even when an input sample is random noise. We introduce the concept of a \don't know" prediction for dermatological assistance. We firstly modify deep models by replacing neural network weights in the penultimate layer with both random variables and dropout during inference. Statistical ambiguity and entropy features are extracted from a skin lesion classifier and fed into an uncertainty detection model for final prediction estimates. We then present an alternative approach to use machine learning to learn the uncertainty features. Our methods utilize machine learned features without traditional hard-thresholding approaches while minimizing false-positives from skin-lesion inputs on our own skin-lesion dataset as well as the popular SD-198 skin-disease dataset.
更多查看译文
关键词
Deep learning, uncertainty analysis, dermatology
AI 理解论文
溯源树
样例
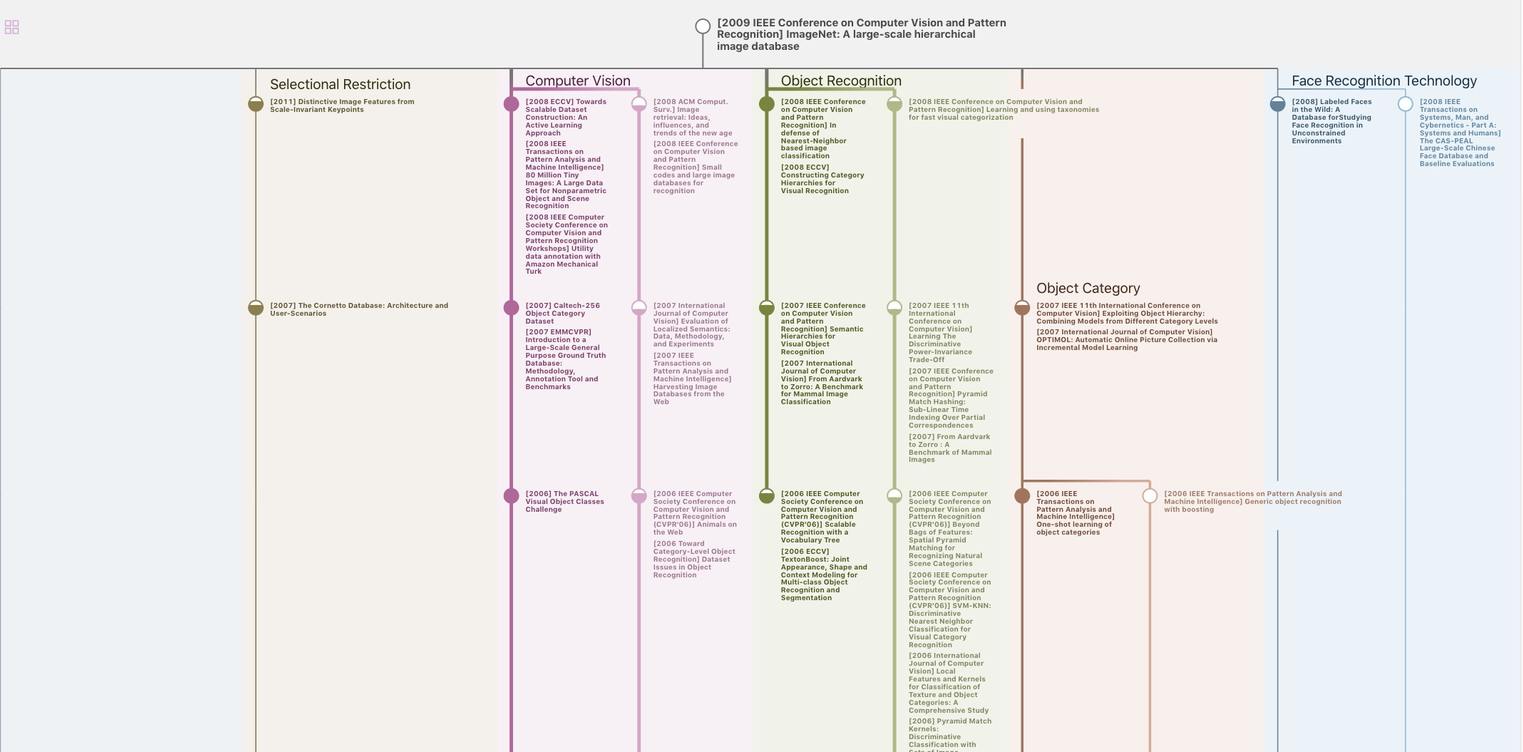
生成溯源树,研究论文发展脉络
Chat Paper
正在生成论文摘要