A Deep Learning Model To Predict Dose-Volume Histograms Of Organs At Risk In Radiotherapy Treatment Plans
MEDICAL PHYSICS(2020)
摘要
Purpose To develop a deep learning-based model to predict achievable dose-volume histograms (DVHs) of organs at risk (OARs) for automation of inverse planning. Methods The model was based on a connected residual deconvolution network (CResDevNet) and compared with UNet as a baseline. The DVHs of OARs are dependent on patient anatomical features of the planning target volumes and OARs, and these spatial relationships can be learned automatically from prior high-quality plans. The contours of planning target volumes and OARs were parsed from the plan database and used as the input to the model, and the dose-area histograms (DAHs) of the OARs were output from the model. The model was trained from scratch by correlating anatomical features with DAHs of OARs, then accumulating these histograms to obtain the final predicted DVH for each OAR. Helical tomotherapy plans for 170 nasopharyngeal cancer patients were used to train and validate the model. An additional 60 patient treatment plans were used to test the predictive accuracy of the model. The DVHs and dose-volume indices (DVIs) of clinical interest for each OAR in the testing dataset were predicted to evaluate the accuracy of the models. The mean absolute errors in the DVIs for each OAR were calculated using each model and statistically compared using a paired-samplest-test. Dice similarity coefficients for areas of the DVHs were also evaluated. Results Dose-volume histograms of 21 OARs in nasopharyngeal cancer were predicted using the models. For each patient, 63 DVIs for all OARs were calculated. Using the 60 patient treatment plans in the testing dataset, 79% and 73% of the DVIs predicted using the CResDevNet and UNet models, respectively, were within 5% of the clinical values. The median value of the DVIs' mean absolute errors was 3.2 +/- 2.5% and 3.7 +/- 2.9% for the CResDevNet and UNet models, respectively. The average dice similarity coefficient for all OARs was 0.965 using the CResDevNet model and 0.958 using the UNet model. Conclusions A deep learning model was developed for predicting achievable DVHs of OARs. The prediction accuracy of the CResDevNet model was evaluated using a planning database of nasopharyngeal cancer cases and shown to be more accurate than the UNet model. Prediction accuracy was also higher for larger-volume OARs. The model can be used for automation of inverse planning and quality assessment of individual treatment plans.
更多查看译文
关键词
dose-volume histogram, deep learning, dose-area histogram, inverse planning
AI 理解论文
溯源树
样例
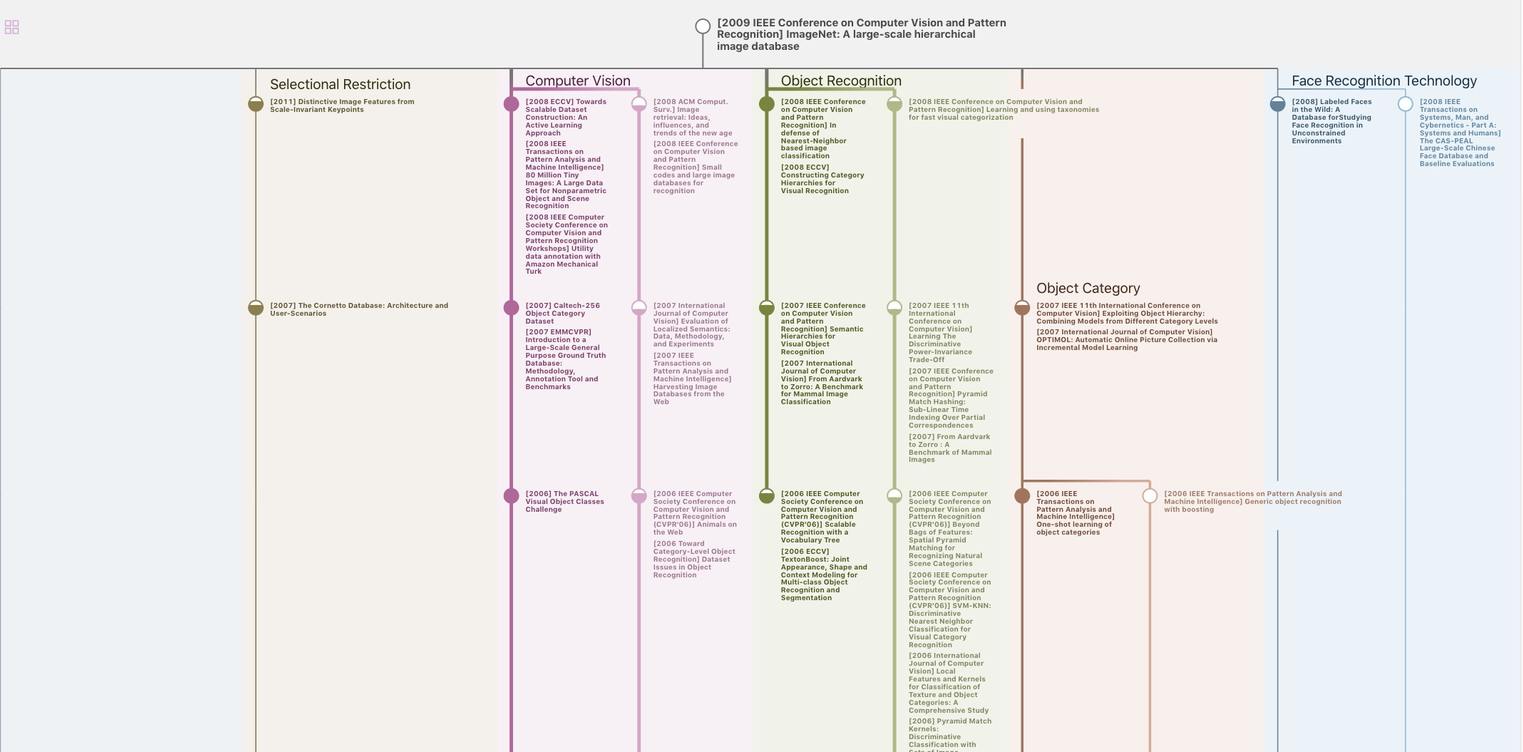
生成溯源树,研究论文发展脉络
Chat Paper
正在生成论文摘要