Huber-type principal expectile component analysis
Computational Statistics & Data Analysis(2020)
摘要
In principal component analysis (PCA), principal components are identified by maximizing the component score variance around the mean. However, a practitioner might be interested in capturing the variation in the tail rather than the center of a distribution to, for example, identify the major pollutants from air pollution data. To address this problem, we introduce a new method called Huber-type principal expectile component (HPEC) analysis that uses an asymmetric Huber norm to provide a kind of robust-tail PCA. The statistical properties of HPECs are derived, and a derivative-free optimization approach called particle swarm optimization (PSO) is used to identify HPECs numerically. As a demonstration, HPEC analysis is applied to real and simulated data with encouraging results.
更多查看译文
关键词
Asymmetric norm,Expectile,Huber’s criterion,Particle swarm optimization,Principal component
AI 理解论文
溯源树
样例
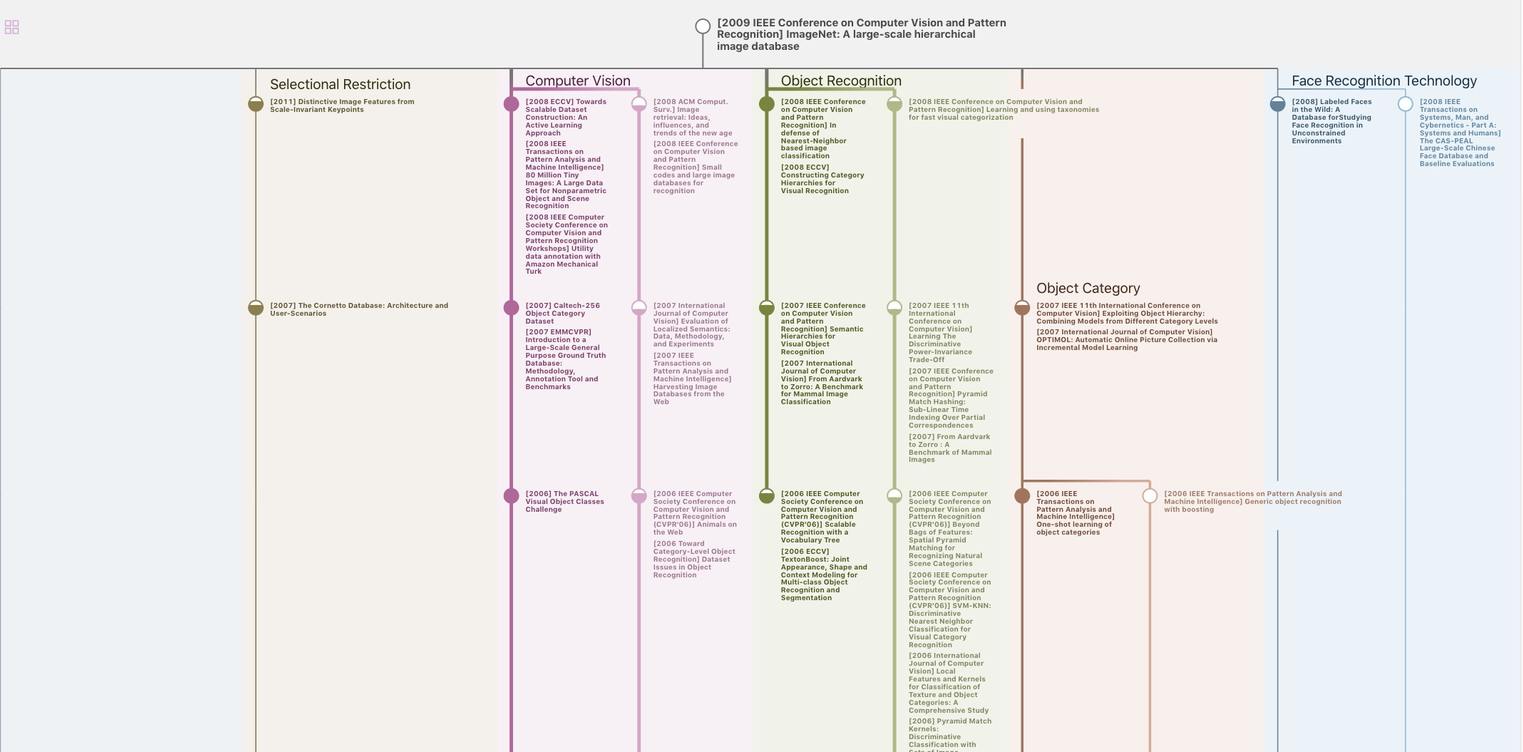
生成溯源树,研究论文发展脉络
Chat Paper
正在生成论文摘要