Black-Box Watermarking for Generative Adversarial Networks
arxiv(2021)
摘要
As companies start using deep learning to provide value to their customers, the demand for solutions to protect the ownership of trained models becomes evident. Several watermarking approaches have been proposed for protecting discriminative models. However, rapid progress in the task of photorealistic image synthesis, boosted by Generative Adversarial Networks (GANs), raises an urgent need for extending protection to generative models. We propose the first watermarking solution for GAN models. We leverage steganography techniques to watermark GAN training dataset, transfer the watermark from the dataset to GAN models, and then verify the watermark from generated images. In the experiments, we show that the hidden encoding characteristic of steganography allows preserving generation quality and supports the watermark secrecy against steganalysis attacks. We validate that our watermark verification is robust in wide ranges against several image perturbations. Critically, our solution treats GAN models as an independent component: watermark embedding is agnostic to GAN details and watermark verification relies only on accessing the APIs of black-box GANs. We further extend our watermarking applications to generated image detection and attribution, which delivers a practical potential to facilitate forensics against deep fakes and responsibility tracking of GAN misuse.
更多查看译文
关键词
generative adversarial networks,black-box
AI 理解论文
溯源树
样例
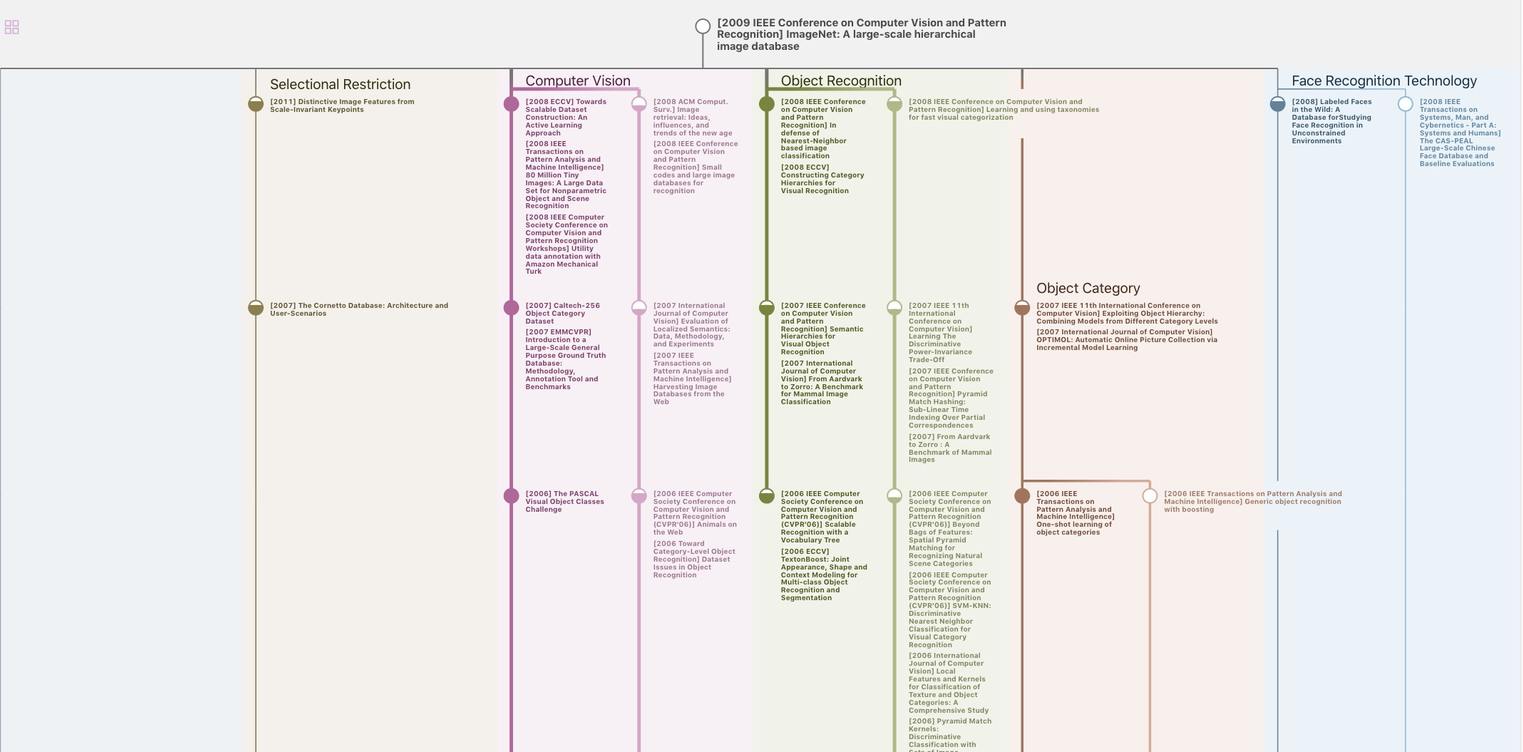
生成溯源树,研究论文发展脉络
Chat Paper
正在生成论文摘要