Exploiting Relationships Between Outcomes In Bayesian Multivariate Network Meta-Analysis With An Application To Relapsing-Remitting Multiple Sclerosis
STATISTICS IN MEDICINE(2020)
摘要
In multivariate network meta-analysis (NMA), the piecemeal nature of the evidence base means that there may be treatment-outcome combinations for which no data is available. Most existing multivariate evidence synthesis models are either unable to estimate the missing treatment-outcome combinations, or can only do so under particularly strong assumptions, such as perfect between-study correlations between outcomes or constant effect size across outcomes. Many existing implementations are also limited to two treatments or two outcomes, or rely on model specification that is heavily tailored to the dimensions of the dataset. We present a Bayesian multivariate NMA model that estimates the missing treatment-outcome combinations via mappings between the population mean effects, while allowing the study-specific effects to be imperfectly correlated. The method is designed for aggregate-level data (rather than individual patient data) and is likely to be useful when modeling multiple sparsely reported outcomes, or when varying definitions of the same underlying outcome are adopted by different studies. We implement the model via a novel decomposition of the treatment effect variance, which can be specified efficiently for an arbitrary dataset given some basic assumptions regarding the correlation structure. The method is illustrated using data concerning the efficacy and liver-related safety of eight active treatments for relapsing-remitting multiple sclerosis. The results indicate that fingolimod and interferon beta-1b are the most efficacious treatments but also have some of the worst effects on liver safety. Dimethyl fumarate and glatiramer acetate perform reasonably on all of the efficacy and safety outcomes in the model.
更多查看译文
关键词
correlated outcomes, evidence synthesis, multivariate network meta-analysis, relapsing-remitting multiple sclerosis, sparse data
AI 理解论文
溯源树
样例
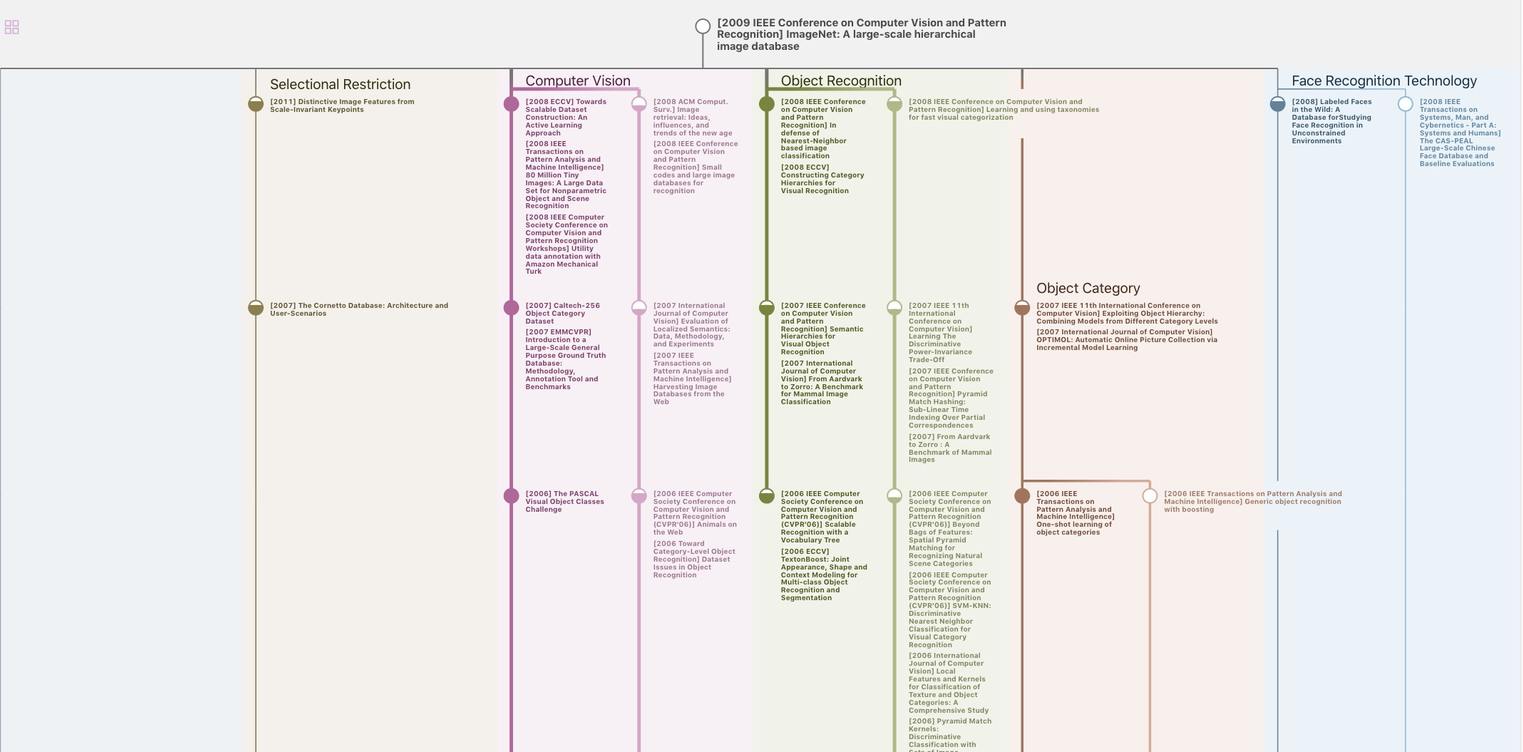
生成溯源树,研究论文发展脉络
Chat Paper
正在生成论文摘要