Augmented B Path Network For Few-Shot Learning
2020 25TH INTERNATIONAL CONFERENCE ON PATTERN RECOGNITION (ICPR)(2020)
摘要
Few-shot Learning (FSL) which aims to learn from few labeled training data is becoming a popular research topic, due to the expensive labeling cost in many real-world applications. One kind of successful FSL method learns to compare the testing (query) image and training (support) image by simply concatenating the features of two images and feeding it into the neural network. However, with few labeled data in each class, the neural network has difficulty in learning or comparing the local features of two images. Such simple image-level comparison may cause serious mis-classification. To solve this problem, we propose Augmented Bi-path Network (ABNet) for learning to compare both global and local features on multi-scales. Specifically, the salient patches are extracted and embedded as the local features for every image. Then, the model learns to augment the features for better robustness. Finally, the model learns to compare global and local features separately, i.e., in two paths, before merging the similarities. Extensive experiments show that the proposed ABNet outperforms the state-of-the-art methods. Both quantitative and visual ablation studies are provided to verify that the proposed modules lead to more precise comparison results.
更多查看译文
关键词
learning,bi-path,few-shot
AI 理解论文
溯源树
样例
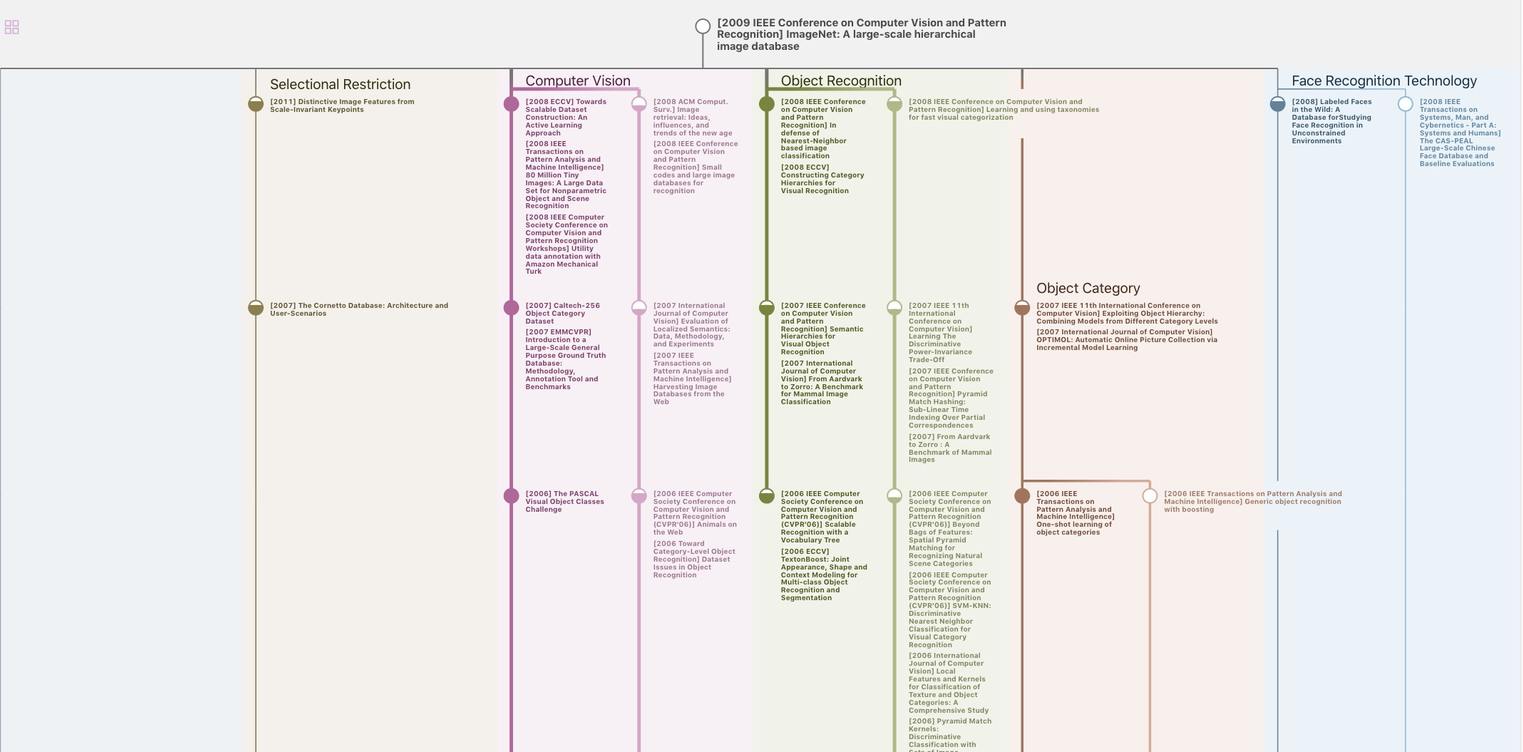
生成溯源树,研究论文发展脉络
Chat Paper
正在生成论文摘要