Physics-informed machine learning for backbone identification in discrete fracture networks
Computational Geosciences(2020)
摘要
The phenomenon of flow-channeling, or the existence of preferential pathways for flow in fracture networks, is well known. Identification of the channels (“backbone”) allows for system reduction and computational efficiency in simulation of flow and transport through fracture networks. However, the purpose of machine learning techniques for backbone identification in fractured media is two-pronged system reduction for computational efficiency in simulation of flow and transport as well as physical insight into the phenomenon of flow channeling. The most critical aspect of this problem is the need to have a truly “physics-informed” technique that respects the constraint of connectivity. We present a method that views a network as a union of connected paths with each path comprising a sequence of fractures. Thus, the fundamental unit of selection becomes a sequence of fractures, classified based on attributes that characterize the sequence. In summary, this method represents a parametrization of the sample space that ensures every selected sample sub-network (which is the union of all selected sequences of fractures) always respects the constraint of connectivity, demonstrating that it is a truly physics-informed method. The algorithm has a user-defined parameter which allows control of the backbone size when using the random forest or logistic regression classifier. Even when the backbones are less than 30% in size (leading to computational savings), the backbones still capture the behavior of the breakthrough curve of the full network. Moreover, there is no need to check for path connectedness in the backbones unlike previous methods since the backbones are guaranteed to be connected.
更多查看译文
关键词
Discrete fracture networks,Fractured porous media,Physics-informed,Data-driven,Machine learning,Classification
AI 理解论文
溯源树
样例
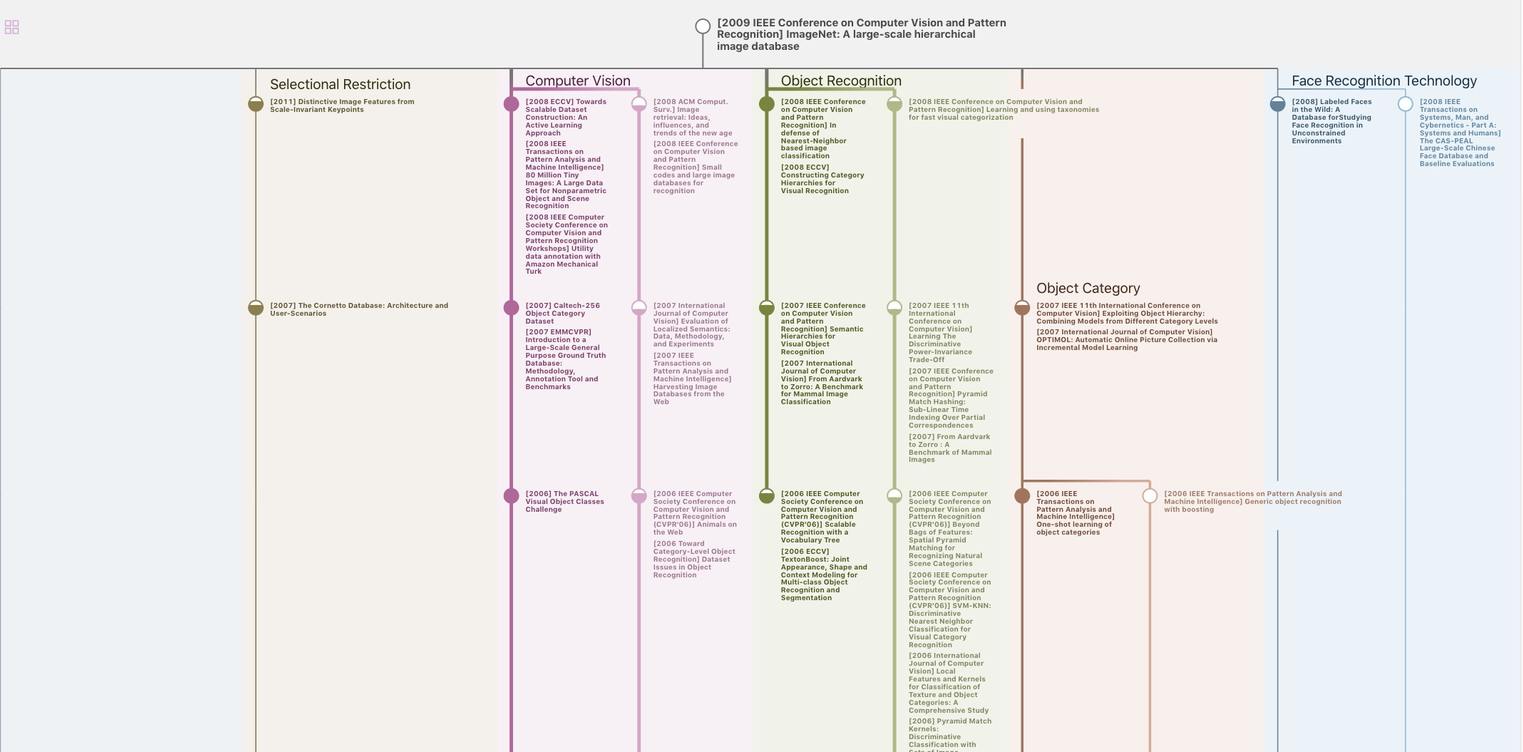
生成溯源树,研究论文发展脉络
Chat Paper
正在生成论文摘要