REPrune: Filter Pruning via Representative Election
arxiv(2020)
摘要
Even though norm-based filter pruning methods are widely accepted, it is questionable whether the "smaller-norm-less-important" criterion is optimal in determining filters to prune. Especially when we can keep only a small fraction of the original filters, it is more crucial to choose the filters that can best represent the whole filters regardless of norm values. Our novel pruning method entitled "REPrune" addresses this problem by selecting representative filters via clustering. By selecting one filter from a cluster of similar filters and avoiding selecting adjacent large filters, REPrune can achieve a better compression rate with similar accuracy. Our method also recovers the accuracy more rapidly and requires a smaller shift of filters during fine-tuning. Empirically, REPrune reduces more than 49% FLOPs, with 0.53% accuracy gain on ResNet-110 for CIFAR-10. Also, REPrune reduces more than 41.8% FLOPs with 1.67% Top-1 validation loss on ResNet-18 for ImageNet
更多查看译文
关键词
filter pruning,representative election
AI 理解论文
溯源树
样例
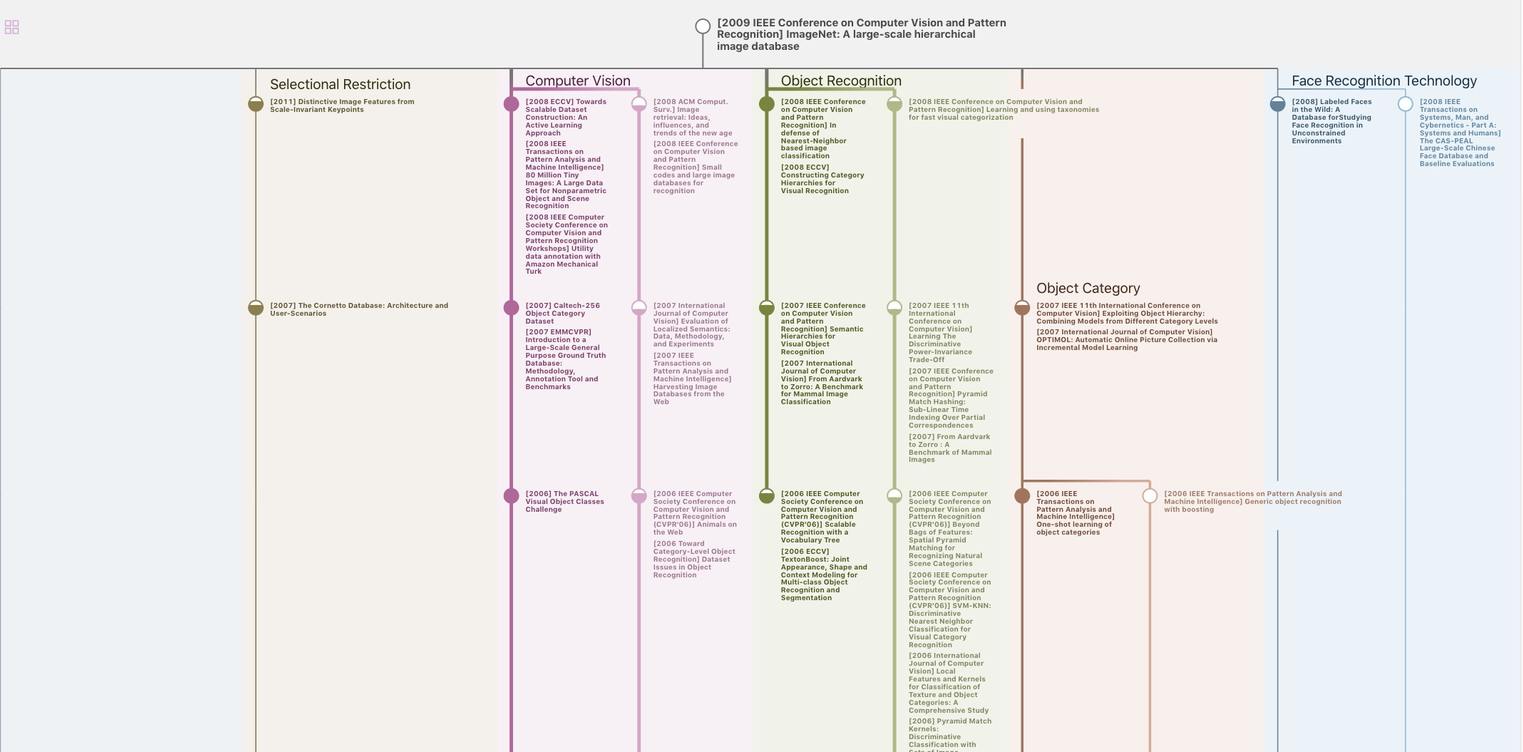
生成溯源树,研究论文发展脉络
Chat Paper
正在生成论文摘要