Ensemble of Cubist models for soy yield prediction using soil features and remote sensing variables
semanticscholar(2017)
摘要
The goal of this work is to develop a predictive model for selecting elite soy variants for commercial production. Current breeding practices for new soy variants require rigorous evaluation over three stages of field tests, corresponding to three successive growing seasons. We propose to leverage machine learning methods for identifying high yielding variants using remote sensing and soil features. To support this proposition, we trained an ensemble of fifteen decision tree models, one for each relative maturity band. Collectively, our models identified fifteen elite varieties from 21 predictive variables to forecast soybean yields in 2015 at 58 test locations. This method can boost commercial soy yields by about 5% and shorten the time for commercial variant development. CCS Concepts •CCS → Applied computing → Computers in other domains → Agriculture
更多查看译文
AI 理解论文
溯源树
样例
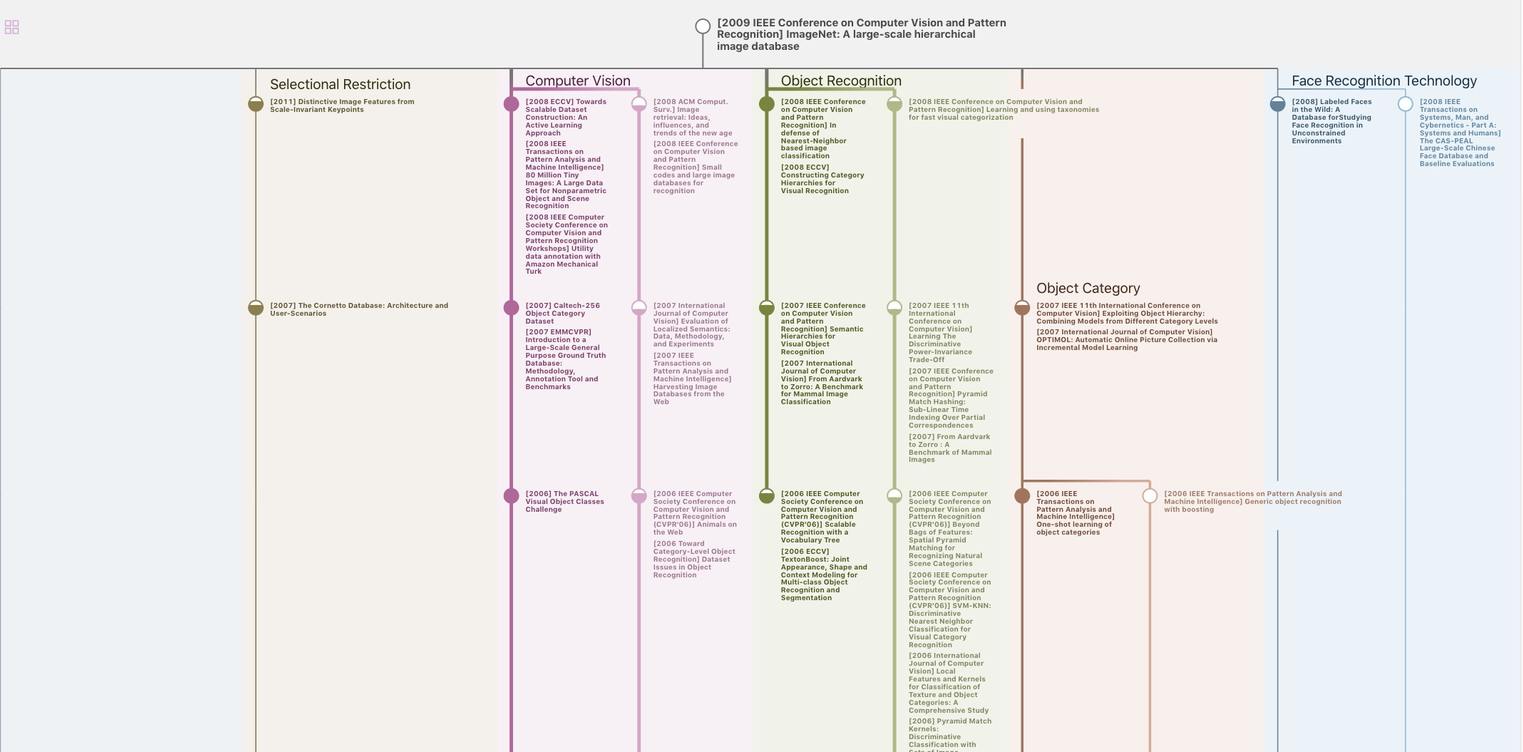
生成溯源树,研究论文发展脉络
Chat Paper
正在生成论文摘要