A model order reduction framework for parametrized nonlinear PDE constrained optimization
semanticscholar(2015)
摘要
In this paper, we propose a model order reduction framework for parametrized quadratic optimization problems constrained by nonlinear stationary PDEs. Once the solutions of the optimization problem are characterized as the solutions of the corresponding optimality system, we build a reduced-order model following a suitable all-at-once optimize-then-reduce paradigm. Lowdimensional spaces for the state, control and adjoint variables are simultaneously constructed by means of either the Reduced Basis greedy algorithm or Proper Orthogonal Decomposition. In order to estimate the error between the high-fidelity and reduced solutions, we derive an a posteriori bound based on Brezzi-Rappaz-Raviart theory; further, a bound for the error on the cost functional is obtained. Then, for the sake of computational efficiency, we integrate into this framework the ROMES method presented in Drohmann et al. (2015) to generate tighter error indicators. Finally, this methodology is applied to the boundary optimal control of parametrized Navier-Stokes equations.
更多查看译文
AI 理解论文
溯源树
样例
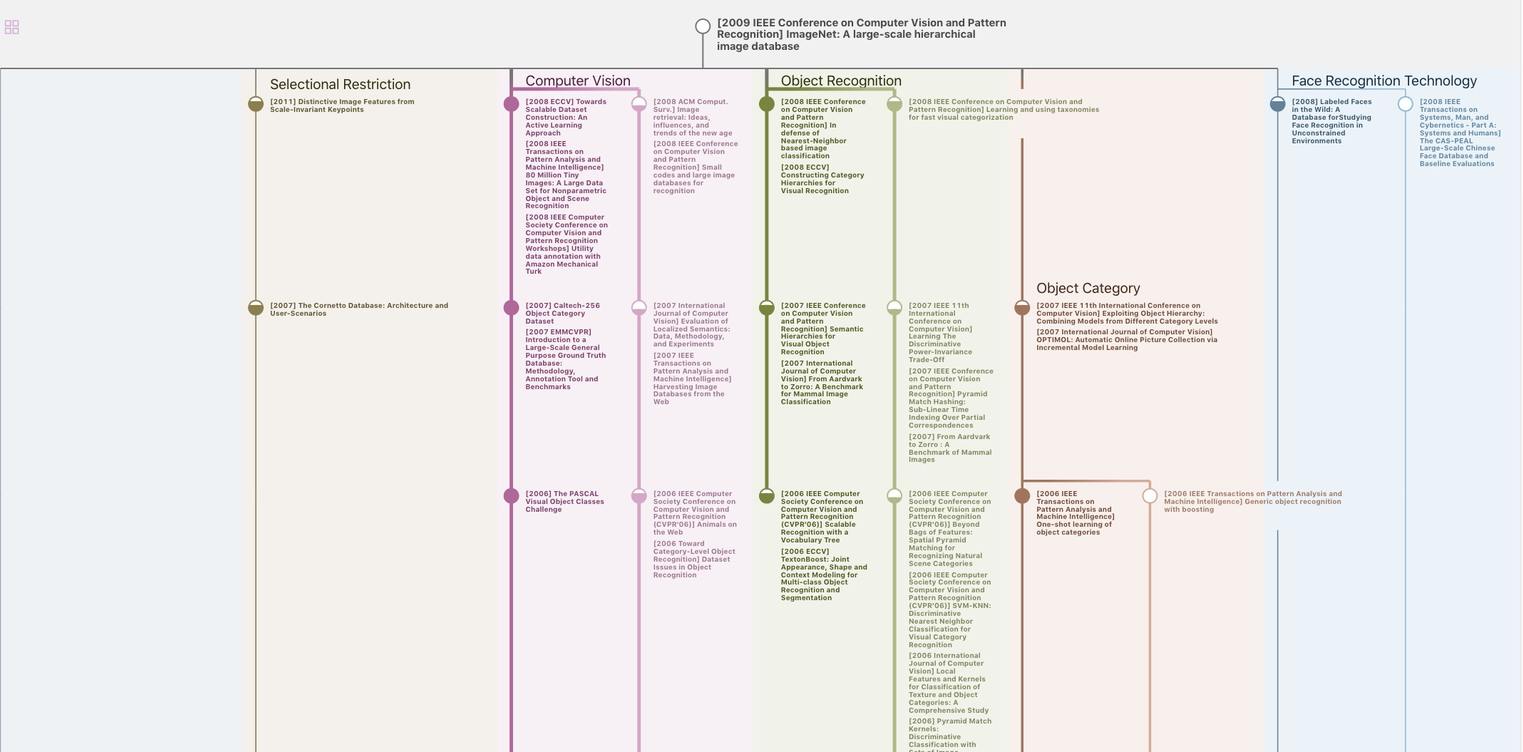
生成溯源树,研究论文发展脉络
Chat Paper
正在生成论文摘要