16-831 Final Report : Online Anomaly Detection in Videos
semanticscholar(2014)
摘要
This project address the problem of detecting interesting or anomalous frames in lengthy surveillance videos in an online unsupervised manner. The motivation of this project is based on the fact that long stretches of video are becoming a prevalent data source. It is estimated that there are 210 million cameras in use today, generating over 1 billion gigabytes of data per day [1]. These lengthy videos pose a challenge for anomaly frame detections because first, it is difficult to acquire ground truth labels for the hours long lengthy videos for anomaly detection. Second, labeled anomalous frames may not be beneficial for test-time predictions since new anomalies tend to differ from known scenes. Third, anomaly frames are rare, making training data highly imbalanced. Last, learning with video data is difficult because of the dataset size. To meet these challenges we explore online unsupervised learning methods for anomaly detection. In this work we aim to form models of normality and use them to detect anomalous, or novel, frames.
更多查看译文
AI 理解论文
溯源树
样例
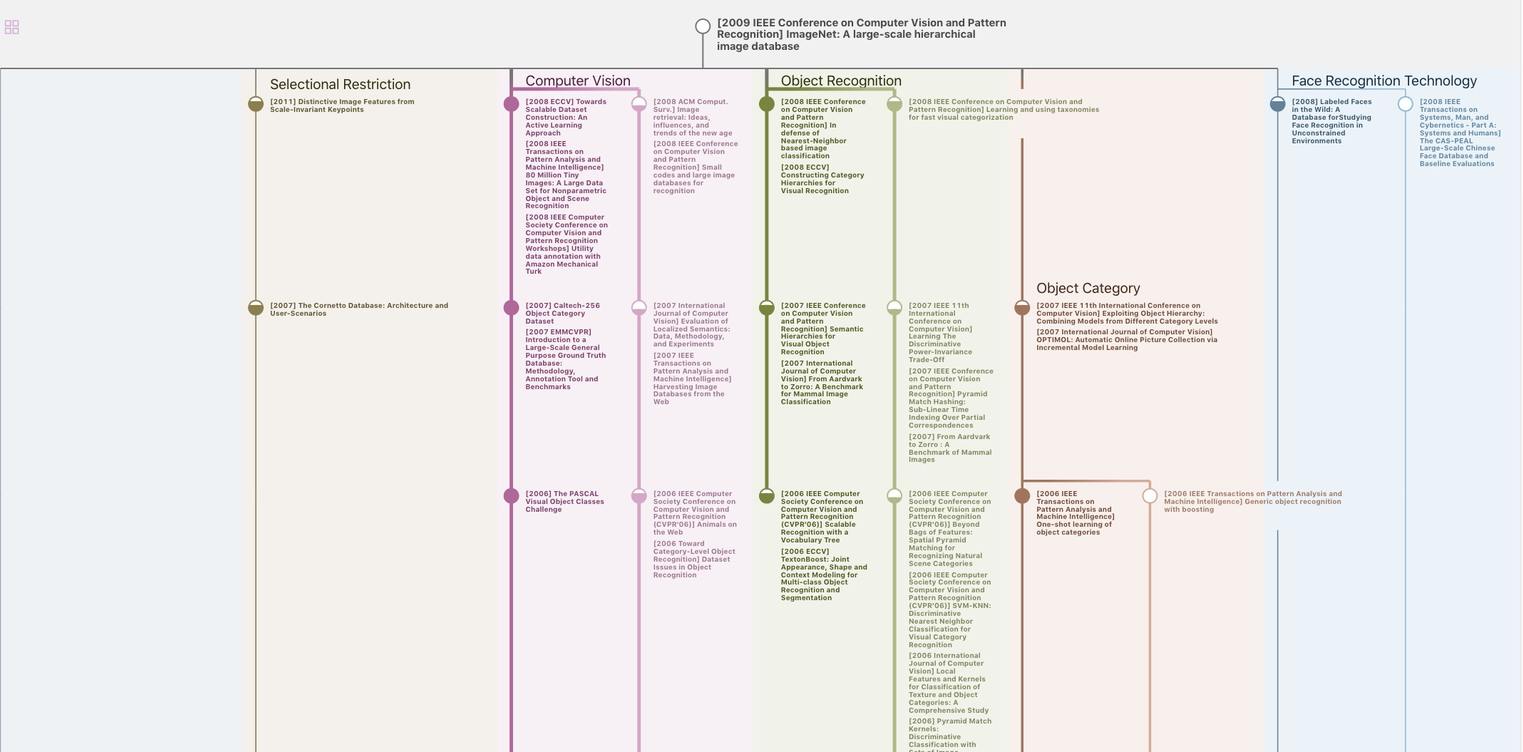
生成溯源树,研究论文发展脉络
Chat Paper
正在生成论文摘要