Communication-efficient k-Means for Edge-based Machine Learning
2020 IEEE 40th International Conference on Distributed Computing Systems (ICDCS)(2020)
摘要
We consider the problem of computing the k-means centers for a large high-dimensional dataset in the context of edge-based machine learning, where data sources offload machine learning computation to nearby edge servers. k-Means computation is fundamental to many data analytics, and the capability of computing provably accurate k-means centers by leveraging the computation power of the edge servers, at a low communication and computation cost to the data sources, will greatly improve the performance of these analytics. We propose to let the data sources send small summaries, generated by joint dimensionality reduction (DR) and cardinality reduction (CR), to support approximate k-means computation at reduced complexity and communication cost. By analyzing the complexity, the communication cost, and the approximation error of k-means algorithms based on state-of-the-art DR/CR methods, we show that: (i) in the single-source case, it is possible to achieve a near-optimal approximation at a near-linear complexity and a constant communication cost, (ii) in the multiple-source case, it is possible to achieve similar performance at a logarithmic communication cost, and (iii) the order of applying DR and CR significantly affects the complexity and the communication cost. Our findings are validated through experiments based on real datasets.
更多查看译文
关键词
Coreset,dimensionality reduction,random projection,k-means,edge-based machine learning
AI 理解论文
溯源树
样例
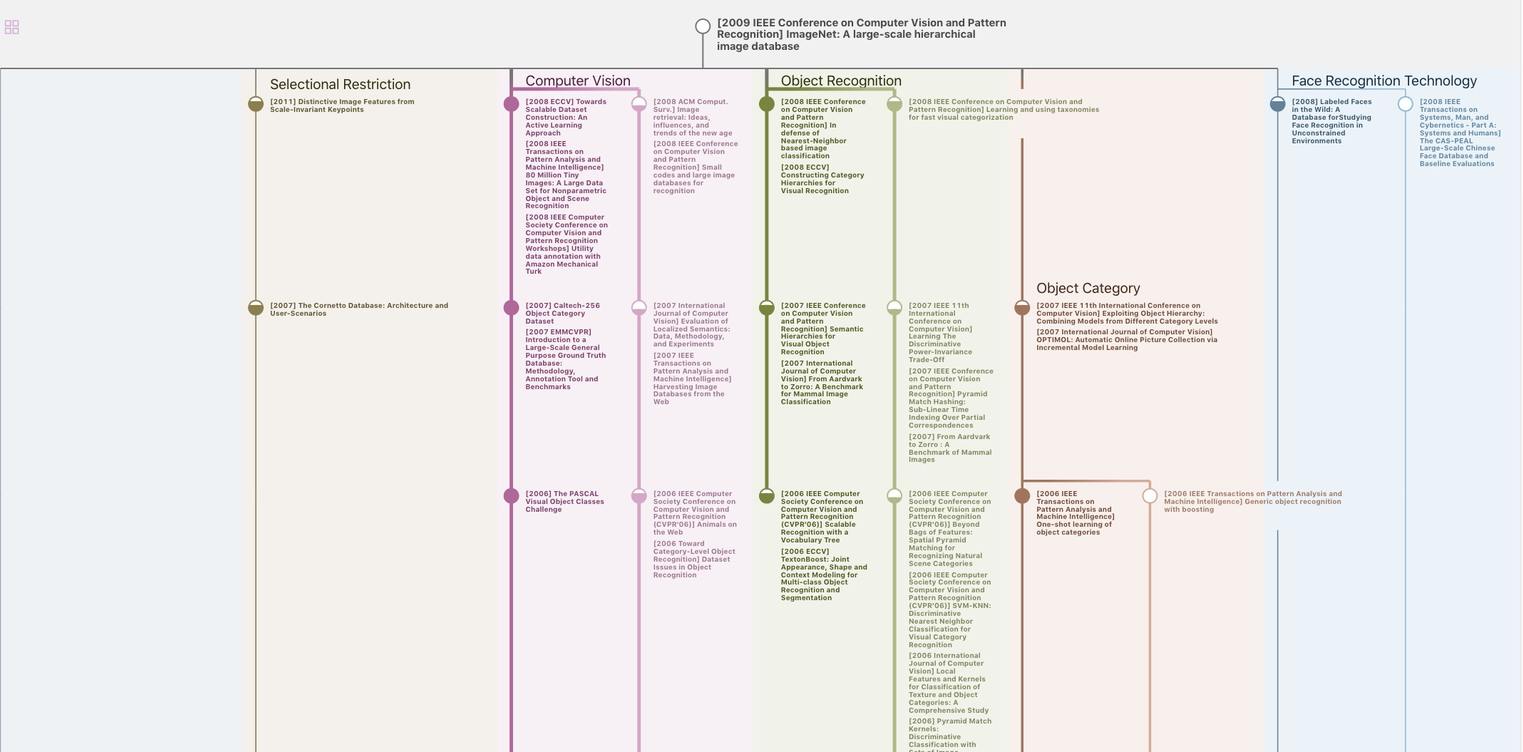
生成溯源树,研究论文发展脉络
Chat Paper
正在生成论文摘要