Mentor : A Visualization and Quality Assurance Framework for Crowd-Sourced Data Generation
semanticscholar(2013)
摘要
Crowdsourcing is a feasible method for collecting labeled datasets for training and evaluating machine learning models. Compared to the expensive process of generating labeled datasets using dedicated trained judges, the low cost of data generation in crowdsourcing environments enables researchers and practitioners to collect significantly larger amounts of data for the same cost. However, crowdsourcing is prone to noise and, without proper quality assurance processes in place, may generate low quality data that is of limited value. In this paper we propose a human-in-the-loop approach to deal with quality assurance (QA) in crowdsourcing environments. We contribute various visualization methods and statistical tools that can be used to identify defective or fraudulent data and unreliable judges. Based on these tools and principles we have built a system called Mentor for conducting QA for datasets used in a large commercial search engine. We describe various tools from Mentor and demonstrate their effectiveness through real cases for generating training and test data for search engine caption generation. Our conclusions and the tools described are generalizable and applicable to processes that collect categorical and ordinal discrete datasets for machine learning.
更多查看译文
AI 理解论文
溯源树
样例
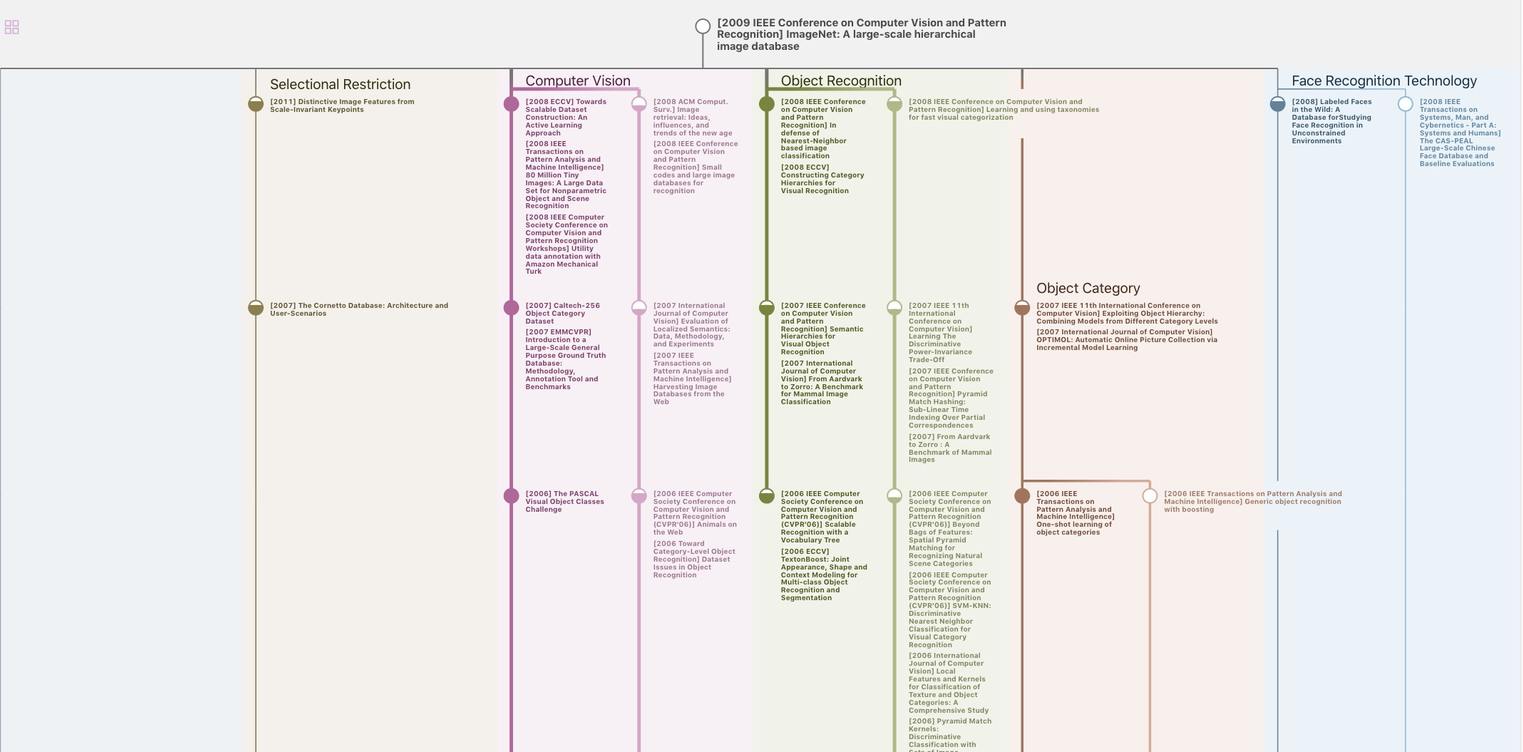
生成溯源树,研究论文发展脉络
Chat Paper
正在生成论文摘要