Differential Privacy in Recommenders
semanticscholar(2014)
摘要
Differential privacy is a relatively recent privacy definition based on the idea that output of a computation should not allow inference about any record’s presence in or absence from the computation input. Nowadays, recommenders are widely used to provide a personalized selection of relevant items to customers. We present various types of privacy risks which can be associated with recommenders [1]. We then describe algorithms which take a moderate amount of auxiliary information about a customer and infer this customer’s transactions from temporal changes in the public outputs of a recommender [2]. These results motivate to design and analyze a realistic recommender built to provide modern privacy guarantees [3]. Finally, we introduce the concept of D2P which is a novel ( , λ)−differential privacy protocol, which provides recommendation quality that is comparable to a non-private approach while providing stronger privacy guarantees than −differential privacy.
更多查看译文
AI 理解论文
溯源树
样例
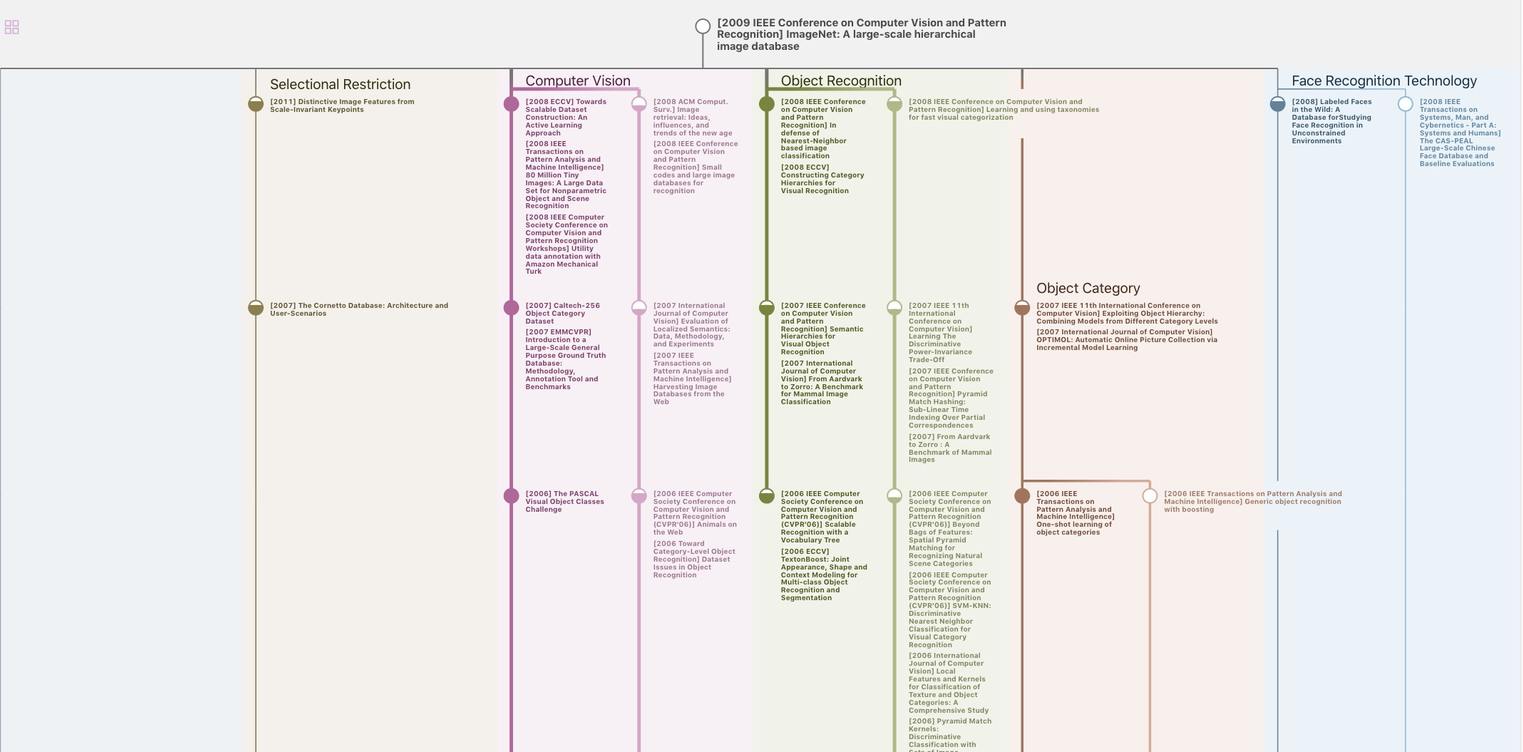
生成溯源树,研究论文发展脉络
Chat Paper
正在生成论文摘要