Learning a Semantic Prior for Guided Navigation
semanticscholar(2018)
摘要
Learning generalizable agents that can adapt to unseen environments remains an open problem in reinforcement learning. We consider visual navigation and address this problem by utilizing latent semantic regularity in human-designed 3D environments, aiming for generalization across scenarios that are visually diverse but semantically consistent. During training, the agent learns subpolicies to reach different semantic concepts, such as ‘move towards the kitchen’, and a prior distribution over their pairwise relationships, such as ‘kitchen is close to dining room’, in the form of a probabilistic graphical model. When testing on new scenarios, the agent dynamically updates its belief of the underlying semantic relationships during exploration and plans its route accordingly towards the final target in an interpretable manner. Our guided navigation method outperforms strong baselines which do not explicitly plan using the semantic content.
更多查看译文
AI 理解论文
溯源树
样例
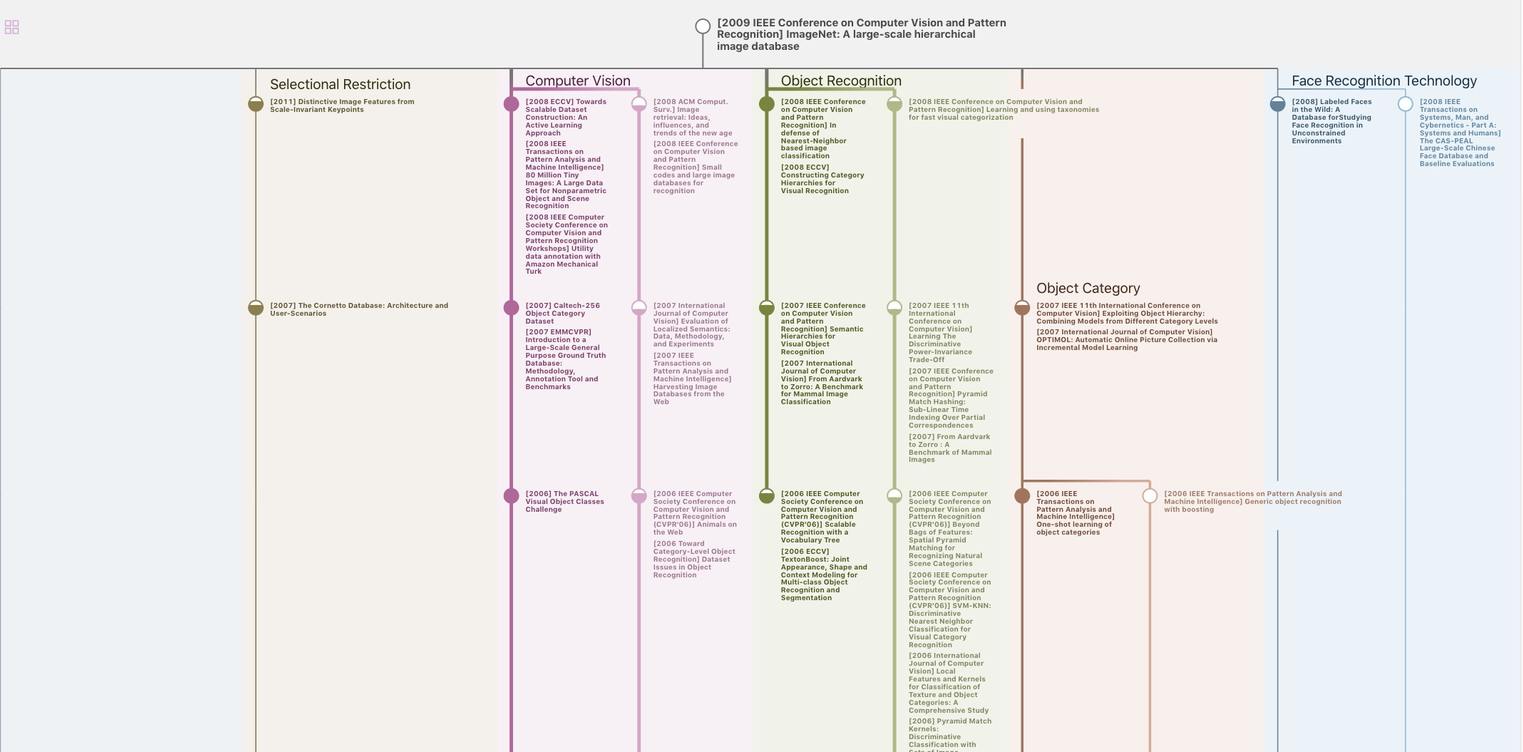
生成溯源树,研究论文发展脉络
Chat Paper
正在生成论文摘要