EXTENDING INFERENCE IN CONTINUOUS TIME BAYESIAN NETWORKS by Liessman
semanticscholar(2013)
摘要
The continuous time Bayesian network (CTBN) has been defined to enable reasoning about complex systems in continuous time by representing the system as a factored, finite-state, continuous-time Markov process. The dynamics of the CTBN are described by each node’s conditional intensity matrices, determined by the states of the parents in the network. As the CTBN is a relatively new model, many extensions that have been defined and researched with respect to Bayesian networks (BNs) have not yet been extended to CTBNs. The proposed research intends to address some of these. First, we intend to formally prove several complexity results with respect to CTBNs. Specifically, it is known that exact inference in CTBN is NP-hard due to the use of a Bayesian network to set the initial states of the nodes. However, we propose to prove that exact inference in CTBNs is still NP-hard even when the initial states are fully observed. Furthermore, we suspect and intend to prove that approximate inference in CTBNs, as with Bayesian networks, is also NP-hard. Second, we propose to formalize both uncertain and negative evidence in the context of CTBNs and extend existing inference algorithms to be able to support these new types of evidence. Third, we show how methods for sensitivity analysis of Markov processes can be applied to the CTBN while exploiting the conditional independence structure of the network. This is done through what we call “node isolation,” which approximates a nodes’ unconditional intensity matrix, analogous to marginalization in a Bayesian network. Lastly, we intend to investigate how and when the node isolation process might be used in approximate inference to increase efficiency without significantly decreasing accuracy. This prospectus reviews preliminary progress on these goals and outlines the direction of future research for the completion of this doctoral research.
更多查看译文
AI 理解论文
溯源树
样例
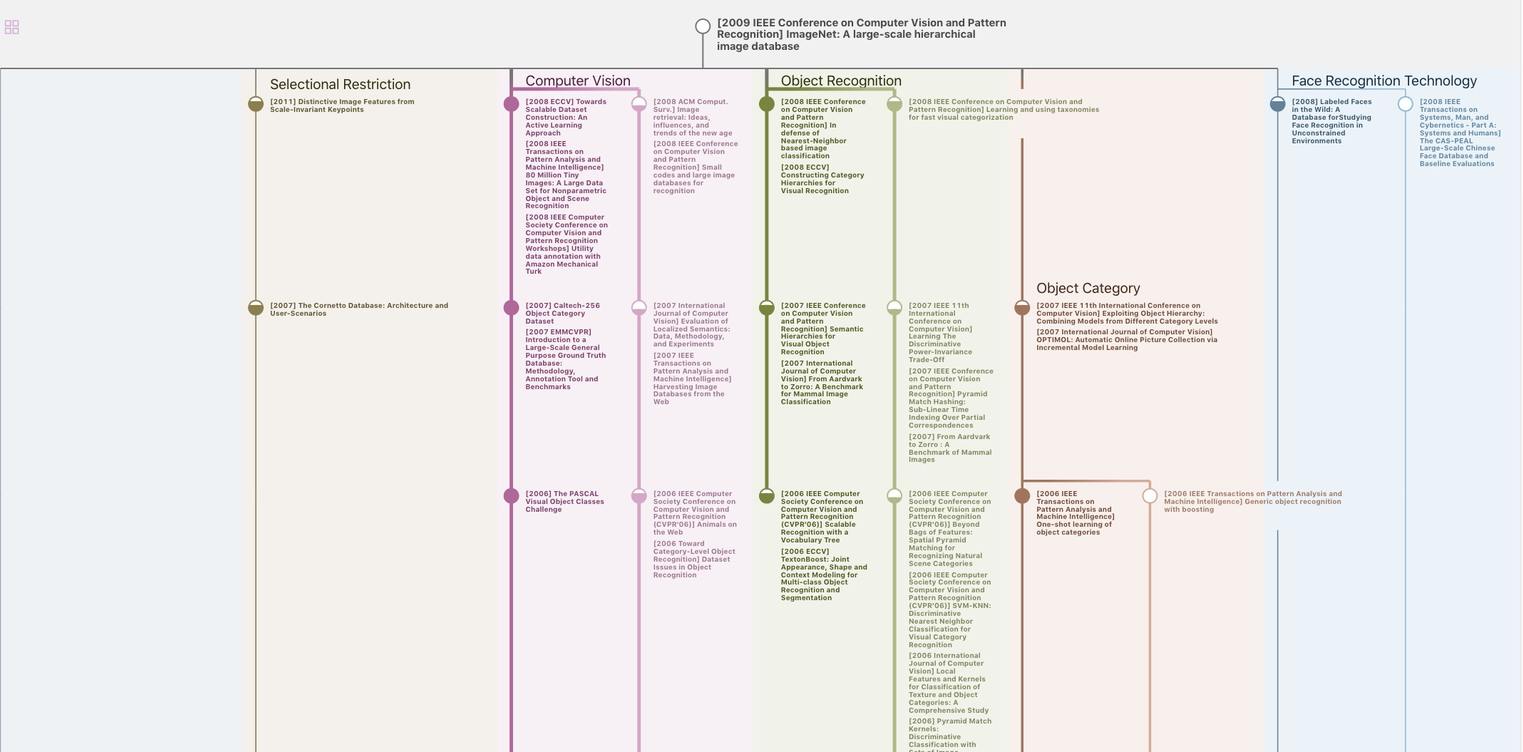
生成溯源树,研究论文发展脉络
Chat Paper
正在生成论文摘要