A Novel Algorithm for Mining Hybrid-Dimensional Association Rules
semanticscholar(2016)
摘要
The important issue for association rules generation is the discovery of frequent itemset in data mining. Most of the existing real time transactional databases are multidimensional in nature. The classical Apriori algorithm mainly concerned with handling single level, single-dimensional boolean association rules. These algorithms scan the transactional databases or datasets many times to find frequent itemsets. This paper considers mining hybrid-dimensional association rules, from transactional database, which is very interesting and useful in real life business decision making. A novel algorithm is proposed for mining hybrid-dimensional association rules using multi index-structures for storing multidimensional interdimensional and intradimensional frequent-itemset, and it stores all frequent 1-itemsets after scanning the entire database first time in the temporary table for compression of the transactional dataset. From then, k-itemsets are generated with no further scan of the datasets. Compared to traditional algorithms, this algorithm efficiently finds association rules in multidimensional datasets, by scanning the dataset only once, thus enhancing the process of data mining.
更多查看译文
AI 理解论文
溯源树
样例
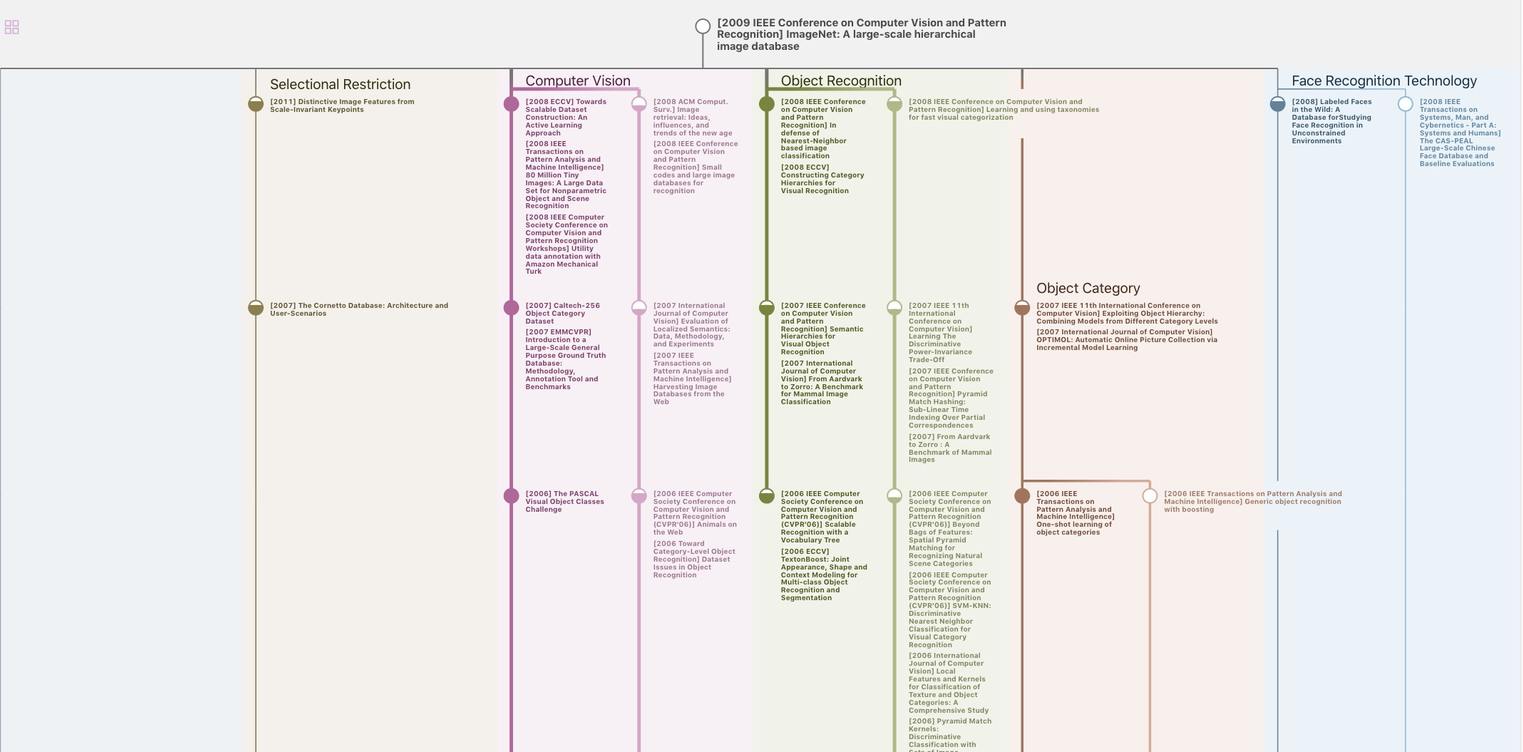
生成溯源树,研究论文发展脉络
Chat Paper
正在生成论文摘要