An Indexing Method for Efficient Model-Based Search
semanticscholar(2013)
摘要
Large databases of patterns, such as faces, body poses, fingerprints, or gestures, are becoming increasingly widespread, thanks to advances in computer technology. In this paper we focus on the problem of efficient search in such databases, when using model-based search. In model-based search, the user submits as a query a classifier, that has been trained to recognize the type of patterns that the user wants to retrieve. While model-based search can lead to good retrieval accuracy, the efficiency of model-based search can be inadequate if we need to apply the query classifier to every single database pattern. We propose a method for improving the efficiency of model-based search. The proposed method assumes that classifiers have been trained using JointBoost, and operates by defining an embedding, which maps both classifiers and database patterns into a common vector space. Using this embedding, the problem of finding the database patterns maximizing the response of the query classifier is reduced to a nearest neighbor search problem in a vector space. This reduction allows the use of standard vector indexing method to speed up the search. In our experiments, we show that the proposed embedding, together with a simple PCAbased indexing scheme, significantly improve the efficiency of model-based search, as measured on a database of face images constructed from the public FRGC-2 dataset.
更多查看译文
AI 理解论文
溯源树
样例
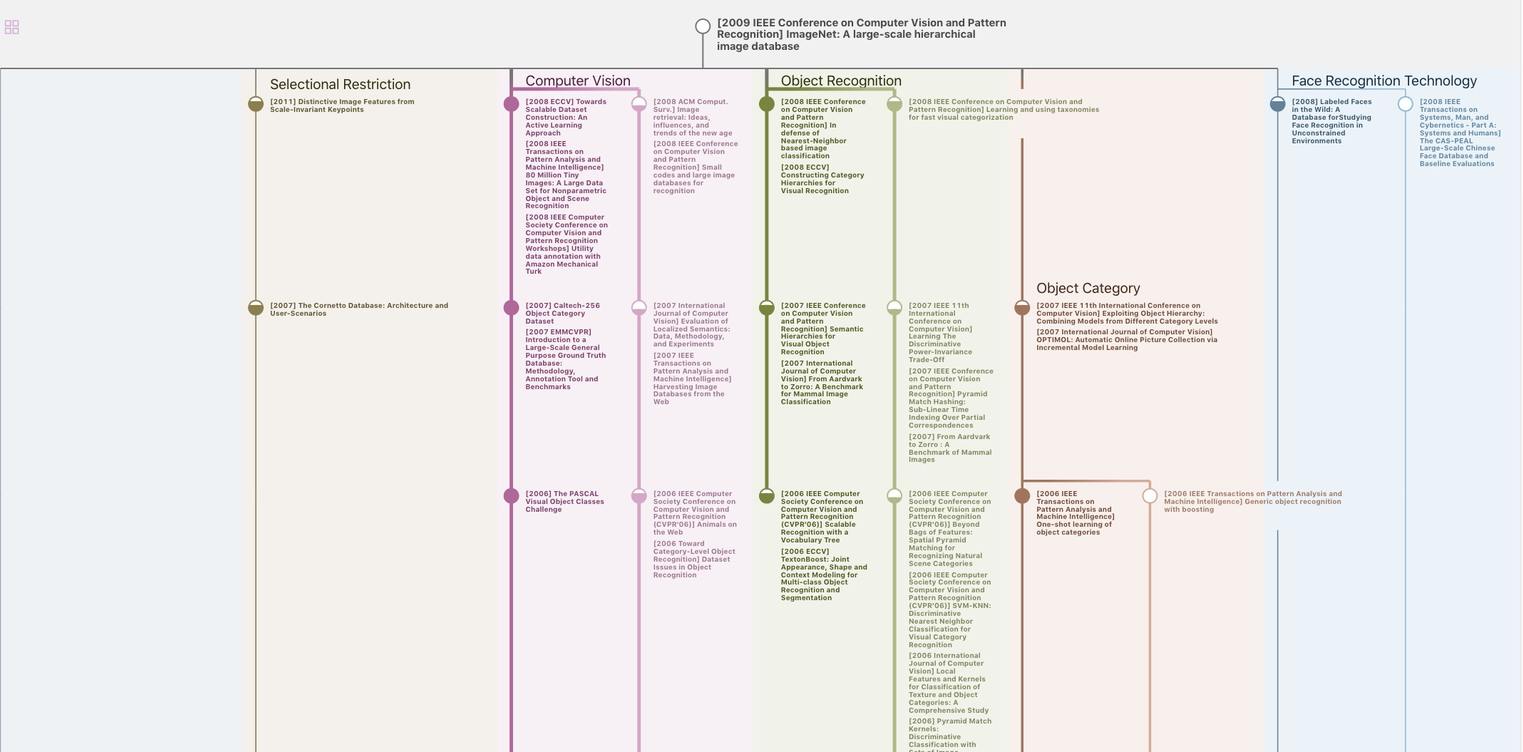
生成溯源树,研究论文发展脉络
Chat Paper
正在生成论文摘要