Identification of Parkinson’s disease related pattern with scaled subprofile model/principle component analysis
semanticscholar(2019)
摘要
Parkinson’s disease (PD) is a severe health problem, the metabolic activity in brain is altered. Using an appropriate brain imaging method and analysing the images by applying some basic algebra principles, such as single value decomposition (SVD), a metabolic brain pattern specific for PD can be identified. The name, established for the statistic method, used for this identificaton, is scaled subprofile model/principle component analysis (SSM/PCA). The purpose of this seminar is to discuss basic principles of SSM/PCA method and its application to F-fluorodeoxyglucose positron emission tomography (FDG-PET) images. All steps of disease related pattern identification process are introduced appropriate patient group selection, pattern identification and validation. In the second part of the seminar application of SSM/PCA to derive a Parkinson’s disease related pattern (PDRP) in a group of Slovenian patients is presented.
更多查看译文
AI 理解论文
溯源树
样例
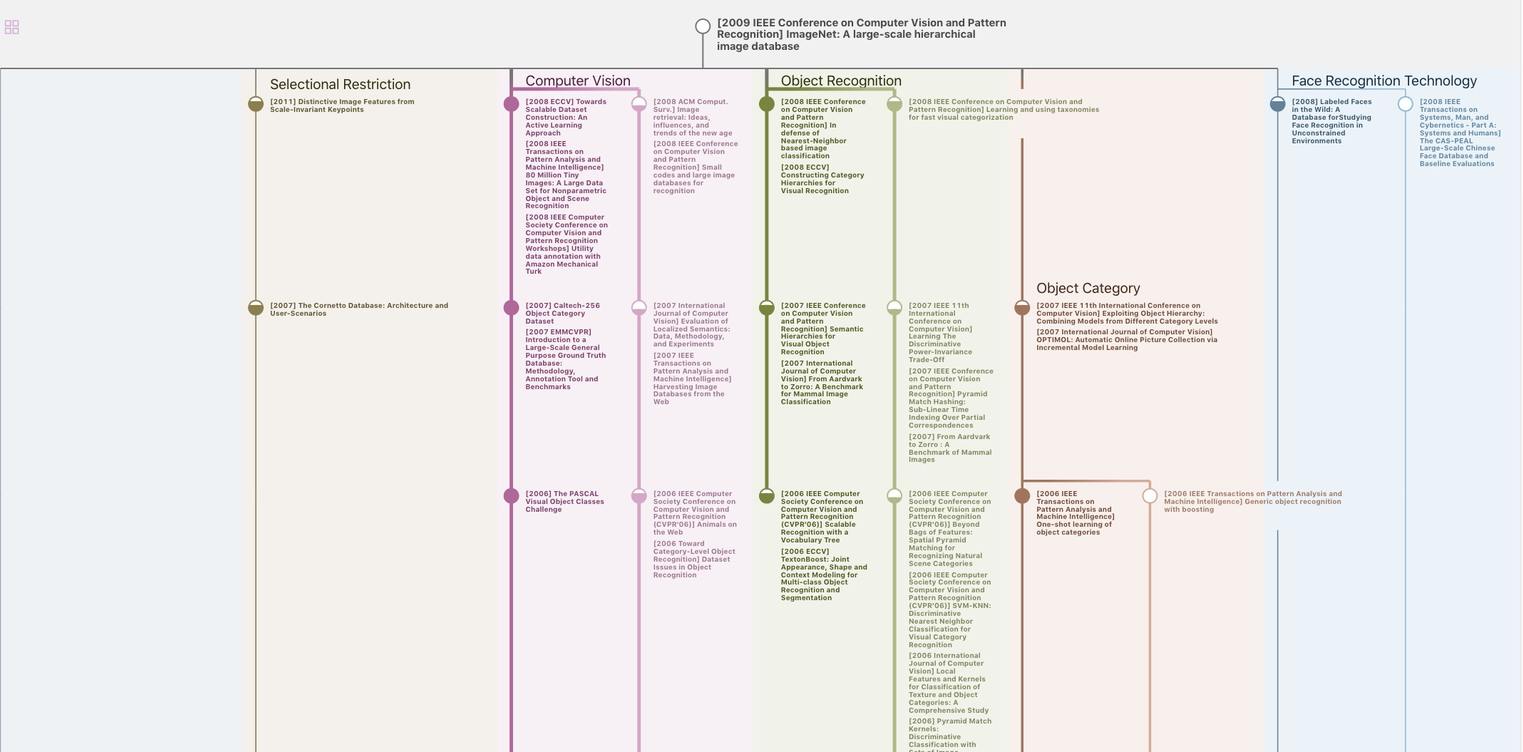
生成溯源树,研究论文发展脉络
Chat Paper
正在生成论文摘要