Multivariate Mutually Regressive Point Processes
semanticscholar(2018)
摘要
Many real-world datasets involve sequences of interdependent events unfolding over time, which are naturally modeled as realizations of a point process. Despite many potential applications, existing point process models are limited in their ability to capture complex patterns of interaction. Hawkes processes (Hawkes, 1971) admit many efficient inference algorithms, but are limited to mutually excitatory interactions. Nonlinear Hawkes processes allow for more complex influence patterns, but we typically must resort to discrete-time approximations to estimate their parameters. In this paper, we introduce a new general class of point processes models extended with a nonlinear component that accounts for inhibitory interactions. We derive a fully Bayesian, continuous time inference algorithm for these processes using Pólya-Gamma augmentation and Poisson thinning. We illustrate the proposed model with an application to analyze multi-neuronal spike train recordings.
更多查看译文
AI 理解论文
溯源树
样例
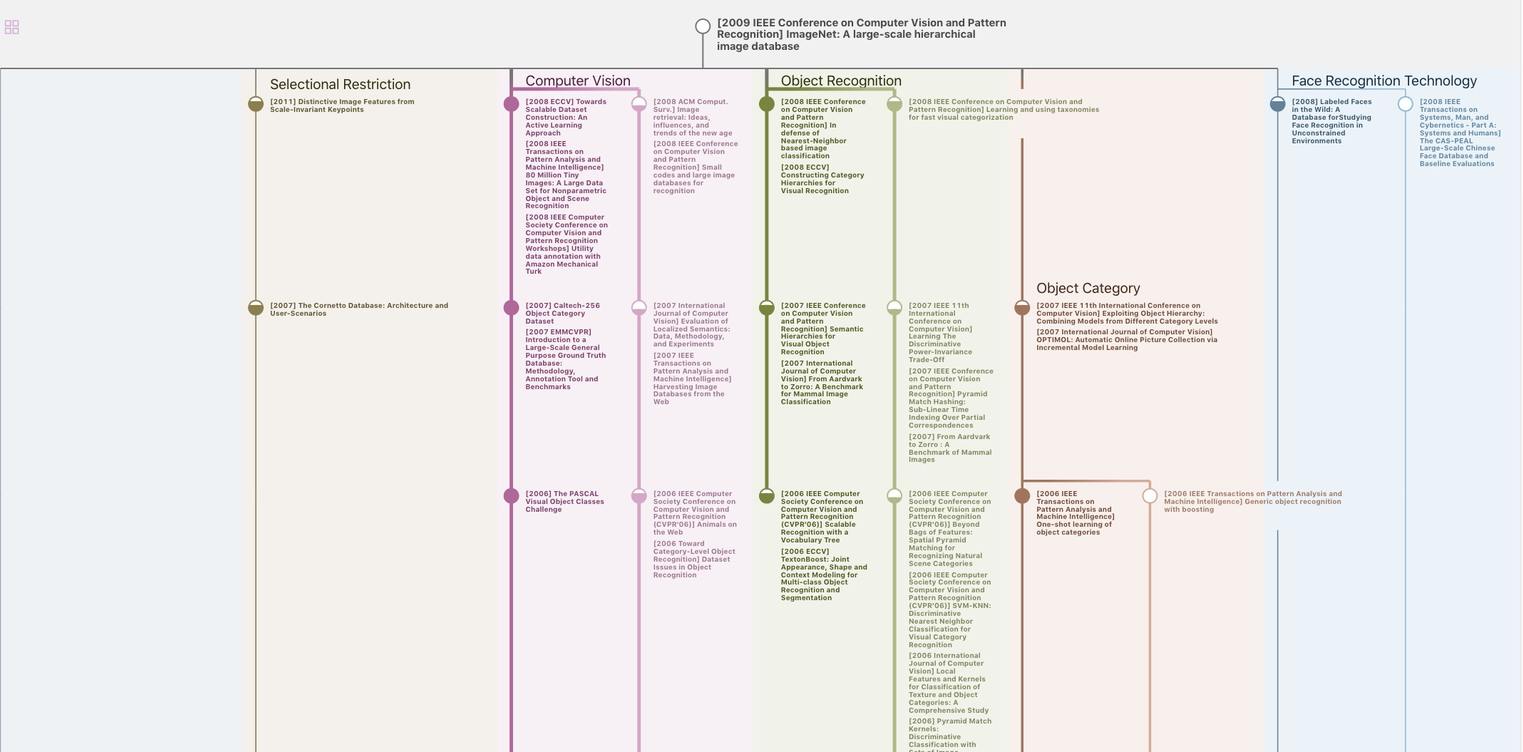
生成溯源树,研究论文发展脉络
Chat Paper
正在生成论文摘要