Improved subcortical segmentation using multiple MR modalities
semanticscholar(2013)
摘要
Purpose Accurate segmentation of subcortical nuclei is required in many neuroscientific studies. Automatic segmentation methods typically depend on T1 contrast to detect boundaries of these nuclei, that is then used to look for between-subject anatomical variability, but T1-weighting does not yield adequate contrast for all boundaries. We aim to improve upon unimodal (T1-weighted) segmentation of subcortical brain structures by using a data-fusion approach that combines multiple images with different contrasts (e.g. T1or T2weighted, FA). As more information is contained in a set of images, the segmentation is more data-driven and has less need to rely on prior knowledge obtained from error-prone manual training data. Methods We use a hierarchical generative model that consists of two parts: 1. Shape model: The mesh that delineates a structure in each individual subject (the ‘shape’) is parameterised by displacements along the normal to a reference surface. The vector contains the displacements at all vertices and is assumed to be distributed as a multivariate normal distribution (MVN) with mean zero and covariance matrix Σ : |Σ | , Σ 2. Intensity model Image intensities are sampled at points along the normals of the reference shape. The profiles from all modalities at vertex are packed into a vector , which is also assumed to be MVN distributed: , , Σ , , Σ , where the mean has dimension and the covariance matrix Σ , has dimension by . The subscript denotes that a subset of length is taken, centred around a displacement . This yields the shorter -dimensional mean vector , and the covariance matrix Σ , with dimension by . We use conjugate priors for both parts of the model and sample from the posterior distribution | , , Α using Gibbs sampling. Here, denotes the training data from which Σ , and Σ are learned and Α denotes all hyperparameters, which are set to reflect our belief that both the shapes and profiles should be smooth. This also serves to regularise the model. Intuitively, the profiles are shifted by amounts to best agree with the reference intensity profiles (part 2) and yield overall shapes that are more probable, as determined from the training data (part 1). The model was trained using displacements generated by FIRST. Data used were from the Human Connectome Project’s Q1 release; with 40 subjects used for training. We used T1-weighted (MPRAGE, 0.7 mm isotropic), T2-weighted (T2-SPACE, 0.7 mm isotropic) and diffusion (SE EPI, monopolar diffusion weighting, multiband, 1.25 mm isotropic) data. The diffusion data are corrected for gradient non-linearity distortions, eddy-current distortions and susceptibility-induced distortions. Inter-modal registrations were carefully evaluated to ensure great accuracy in the alignment. Results Examples of areas where multimodal segmentation obviously improves on the results from FIRST (which only uses the T1-weighted image) are displayed in Fig. 1. The figure illustrates how the inclusion of multiple modalities helps segmentation: at the point highlighted in the globus pallidus (Fig. 1c), there is no perceivable contrast in the T1-weighted volume, but the T2-weighted and FA volumes can inform segmentation here. Discussion Initial results indicate that the approach is successful at integrating information from multiple modalities. It performs better than FIRST in areas with low T1-weighted contrast, as FIRST has to rely on its shape model. Because the training data were generated with FIRST in this case, the boundaries may be biased in areas where FIRST consistently overor underestimates the extent of the structure. However, that has not prevented the current method correcting errors in FIRST segmentations in many cases, as can be seen in the figures here. In the future we intend to refine the training data by manually examining and correcting these training segmentations. Conclusion Parts of subcortical structures may be clearly visible with one MR contrast but not with another. A multimodal approach to segmentation can take advantage of this to produce more accurate results. References 1. Patenaude, B. et al. A Bayesian Model of Shape and Appearance for Subcortical Brain Segmentation. NeuroImage, 56(3):907-922, 2011. 2. Van Essen, D.C. et al. for the WU-Minn HCP Consortium. (2013). The WU-Minn Human Connectome Project: An Overview. NeuroImage 80:62-79, 2013. 3. Sotiropoulos, S.N. et al. for the WU-Minn HCP Consortium. Advances in Diffusion MRI Acquisition and Processing in the Human Connectome Project. NeuroImage 80:125–143, 2013. Data were provided by the Human Connectome Project, WU-Minn Consortium (Principal Investigators: David Van Essen and Kamil Ugurbil; 1U54MH091657) funded by the 16 NIH Institutes and Centers that support the NIH Blueprint for Neuroscience Research; and by the McDonnell Center for Systems Neuroscience at Washington University. Figure 1. Fitted shapes for the left pallidum (a-c) and left putamen (d-f, different subject). Images show results from the proposed method (green) and FIRST (red). Plots (b and e) show measured normalised profiles (black) and reference profiles with standard deviation (red) corresponding to the selected point (magenta marker).
更多查看译文
AI 理解论文
溯源树
样例
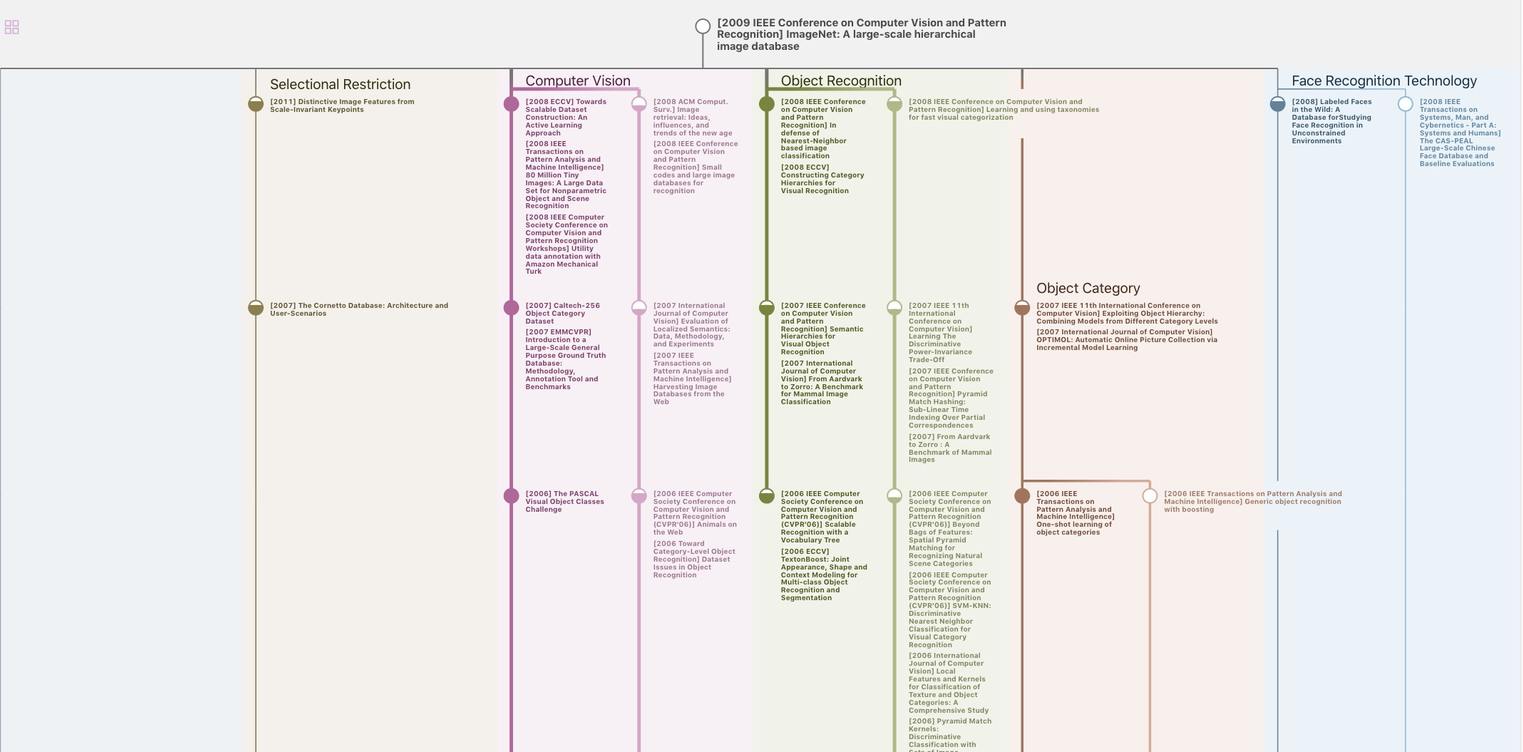
生成溯源树,研究论文发展脉络
Chat Paper
正在生成论文摘要