Video Compression through Deep Bayesian Learning
semanticscholar(2018)
摘要
Motivated by promosing results from neural image compression [1, 5, 4, 24, 19], we propose, to the best of our knowledge, a first step towards innovating beyond block-based hybrid codecs by framing video compression in a deep probabilistic context. Our end-to-end neural video compression scheme is based on sequential variational autoencoders [6, 8, 16] and the approach of Ballé et al. [4] for discretizing and entropy coding a continuous latent representation. Our approach simultaneously learns the optimal transform of the video to a low-dimensional representation and a powerful predictive model that assigns probabilities to video segments, allowing us to efficiently entropy-code the discretized latent representation into a short code length. We introduce both local latent variables, which are inferred from a single frame, and a global latent state, inferred from an entire segment, to efficiently store a video sequence.
更多查看译文
AI 理解论文
溯源树
样例
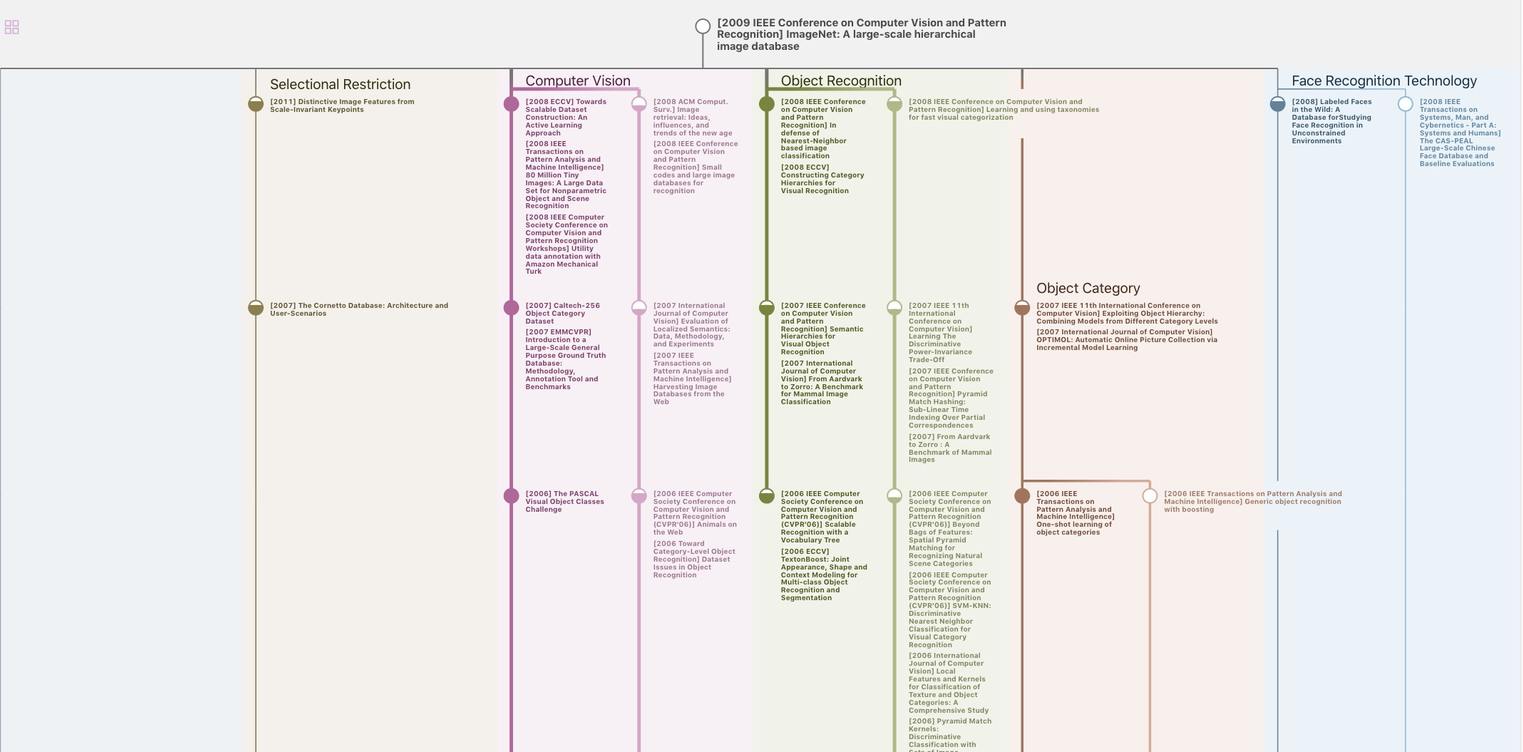
生成溯源树,研究论文发展脉络
Chat Paper
正在生成论文摘要