Training Deep Gaussian Processes with Sampling
semanticscholar(2016)
摘要
In this workshop paper, we explore deep Gaussian processes (deep GPs), a class of models for regression that combines Gaussian processes (GPs) with deep architectures. Exact inference on deep GPs is intractable, and while variational approximation methods have been proposed, these models are difficult to implement and do not extend easily to arbitrary kernels. We propose a stochastic gradient algorithm which relies on sampling to circumvent the intractability hurdle and uses pseudo data to ease the computational burden. To illustrate the model properties, we train various deep architectures on a noisy step-function and toy non-stationary data. By comparing the predictive distributions of each model, we show that deep GPs are well-suited to fit non-stationary functions.
更多查看译文
AI 理解论文
溯源树
样例
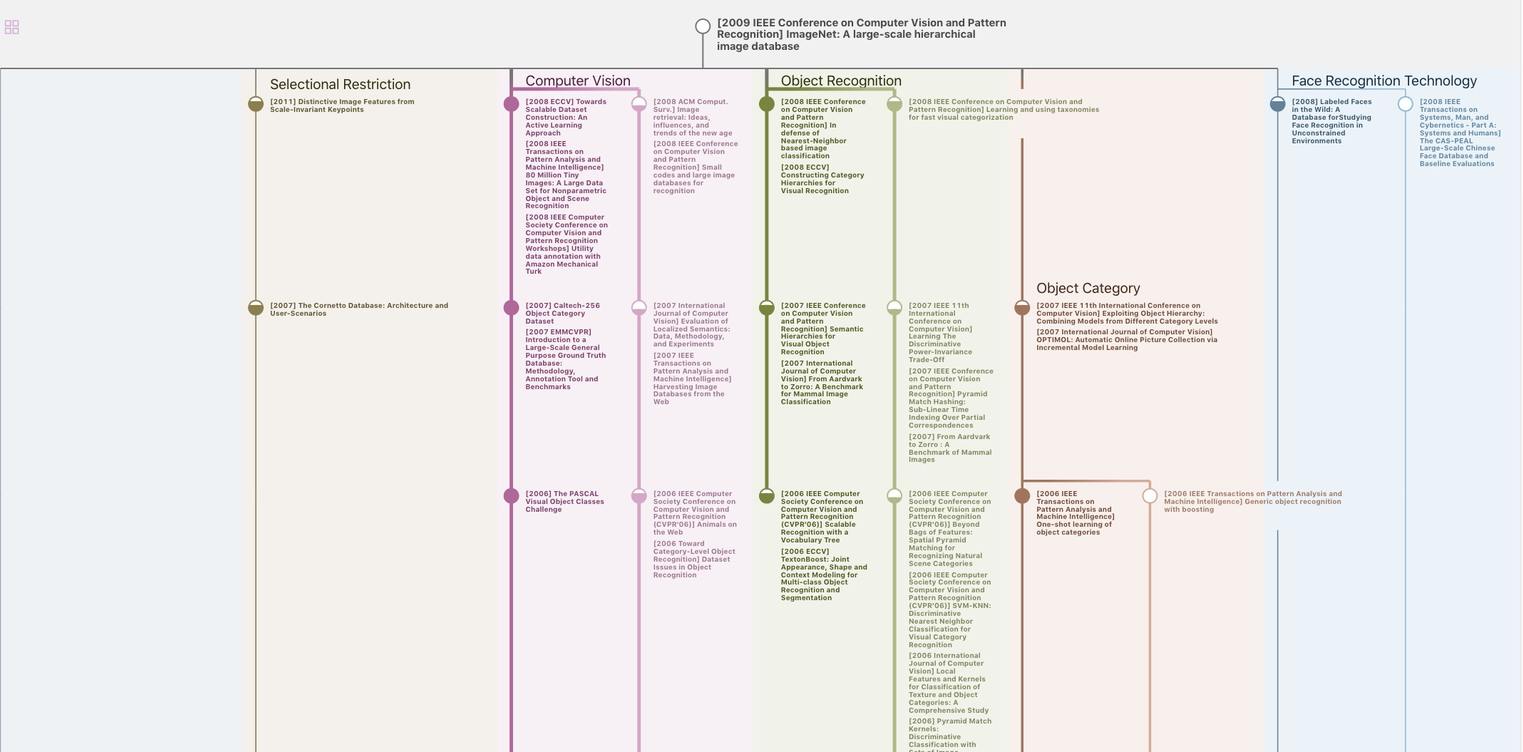
生成溯源树,研究论文发展脉络
Chat Paper
正在生成论文摘要