Mining Large Point Clouds for Feature Matching of LIDAR Datasets using Self-Similarity
semanticscholar(2017)
摘要
LIDAR captured point cloud datasets are another type of big data. Mining such large point clouds for feature selection to perform point cloud matching is a problem of fundamental importance for the geospatial sciences. Standard approaches to point cloud matching face a number of challenges including datasets that exhibit spatial and scale variations. This paper proposes a novel 3D feature descriptor, referred to as “Self-Similar Spin Images”, that provides a robust method to perform spatial matching of point clouds by combining the robustness of local self-similarity with the descriptive power of spin images. This descriptor is used to detect the scale difference between point clouds by introducing the “Self-Similar Keyscale” metric. The proposed method was tested with model and LIDAR datasets.
更多查看译文
AI 理解论文
溯源树
样例
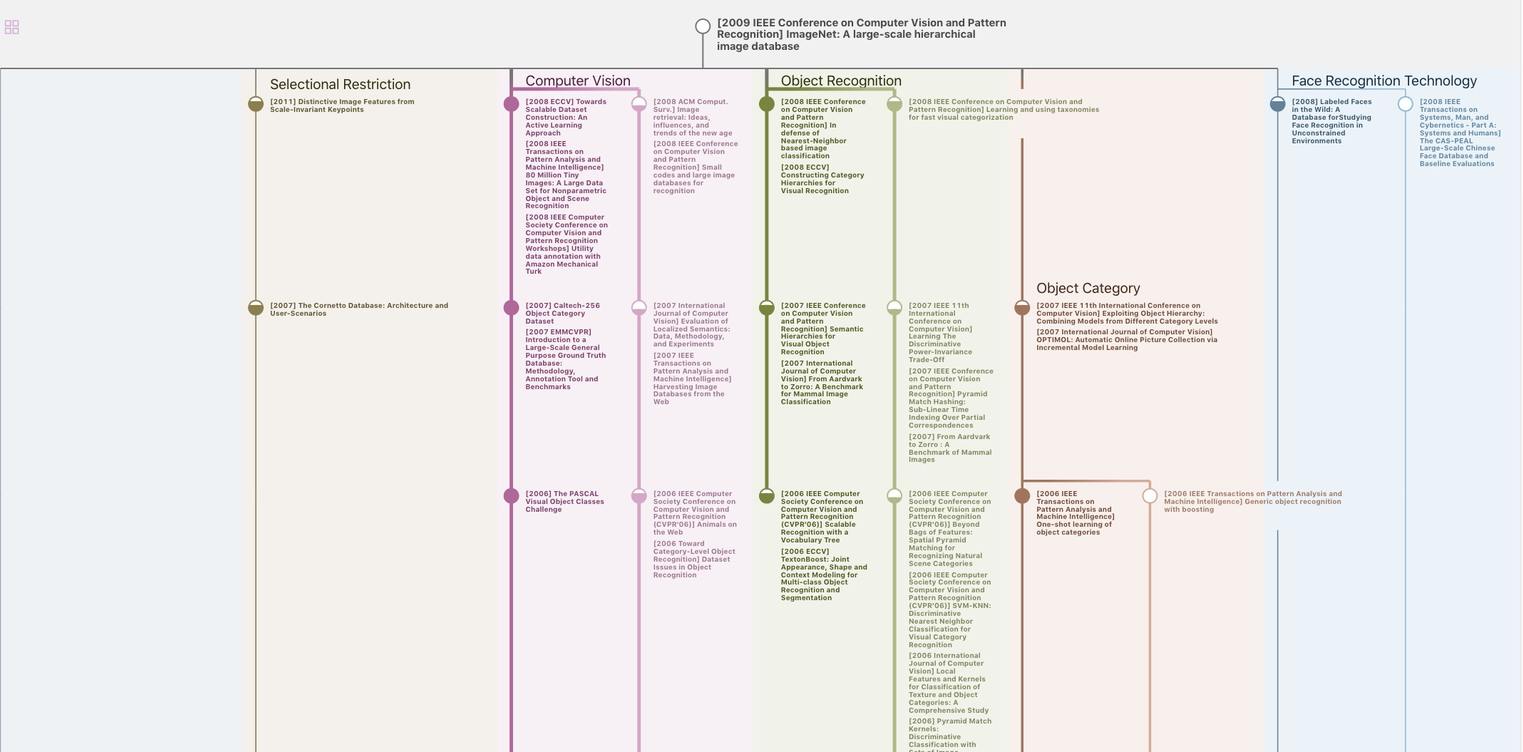
生成溯源树,研究论文发展脉络
Chat Paper
正在生成论文摘要