ST ] 4 N ov 2 01 6 Kernel-based Tests for Joint Independence
semanticscholar(2018)
摘要
We investigate the problem of testing whether d possibly multivariate random variables, which may or may not be continuous, are jointly (or mutually) independent. Our method builds on ideas of the two variable Hilbert-Schmidt independence criterion (HSIC) but allows for an arbitrary number of variables. We embed the joint distribution and the product of the marginals into a reproducing kernel Hilbert space and define the d-variable Hilbert-Schmidt independence criterion (dHSIC) as the squared distance between the embeddings. In the population case, the value of dHSIC is zero if and only if the d variables are jointly independent, as long as the kernel is characteristic. Based on an empirical estimate of dHSIC, we investigate three different non-parametric hypothesis tests: a permutation test, a bootstrap analogue and a procedure based on a Gamma approximation. We apply nonparametric independence testing to a problem in causal discovery and illustrate the new methods on simulated and a real dataset.
更多查看译文
AI 理解论文
溯源树
样例
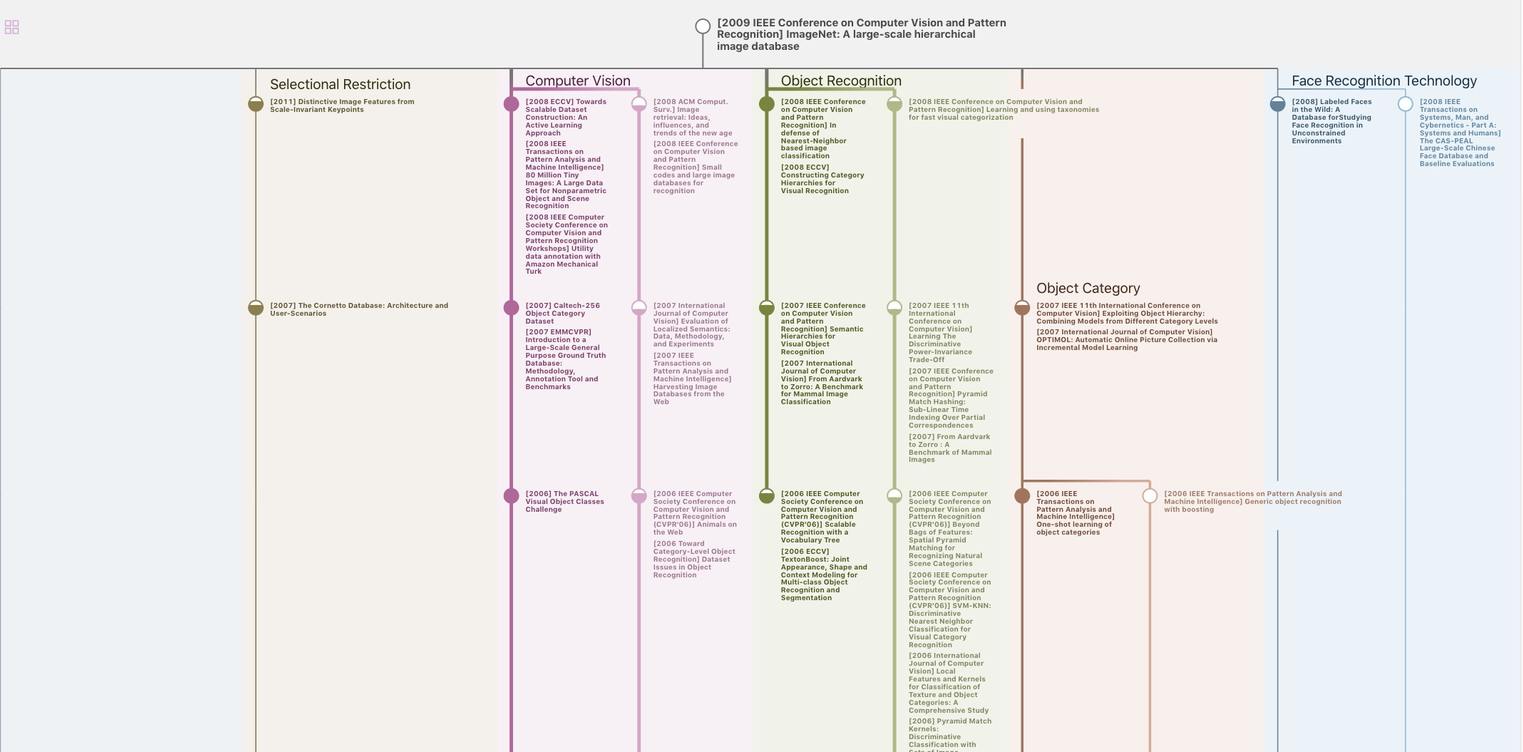
生成溯源树,研究论文发展脉络
Chat Paper
正在生成论文摘要