Self-Supervised Deep Depth Denoising (Supplementary Material)
semanticscholar(2019)
摘要
In this supplementary material we complement our original manuscript with additional quantitative and qualitative results, which better showcase the advantages of the proposed self-supervised denoising model over traditional filtering and supervised CNN-based approaches. In particular, we present the adopted implementation details used for training our model, as well as additional qualitative results for the two 3D application experiments presented in the original manuscript, namely 3D scanning with KinectFusion [8] and full-body 3D reconstructions using Poisson 3D surface reconstruction [4]. A comparative evaluation with the learning-based state-of-the-art methods on InteriorNet (IN) [7] follows, while an ablation study concludes the document. The aforementioned results based on all methods presented in the originally manuscript, namely Bilateral Filter (BF [10]), Joint Bilateral Filter (JBF [6]), Rolling Guidance (RGF [12]), and data-driven approaches (DRR [3], DDRNet [11]). Note that for the DRR and DDRNet methods, additional results aim to highlight the over-smoothing effect of the former and the weakness of the latter to denoise depth maps captured by the Intel RealSense D415 sensors. In more detail, DRR is trained on static scenes that contain dominant planar surfaces and, thus tends to flatten (i.e. oversmooth) the input data. On the other hand, the available DDRNet model 1 that we used, produces high levels of flying pixels (i.e. spraying, see Fig. 1) which can be attributed to background (zero depth values) and foreground blending, even though its predictions are appropriately masked. While the authors have not provided the necessary information, it is our speculation that the available model is trained using Kinect 1 data, which is partly supported by the suboptimal results it produces on Kinect 2 data. Qualitatively, the remaining traditional filters (BF, JBF, RGF) perform similarly, with RGF showcasing the most competitive results to our method. However, note that
更多查看译文
AI 理解论文
溯源树
样例
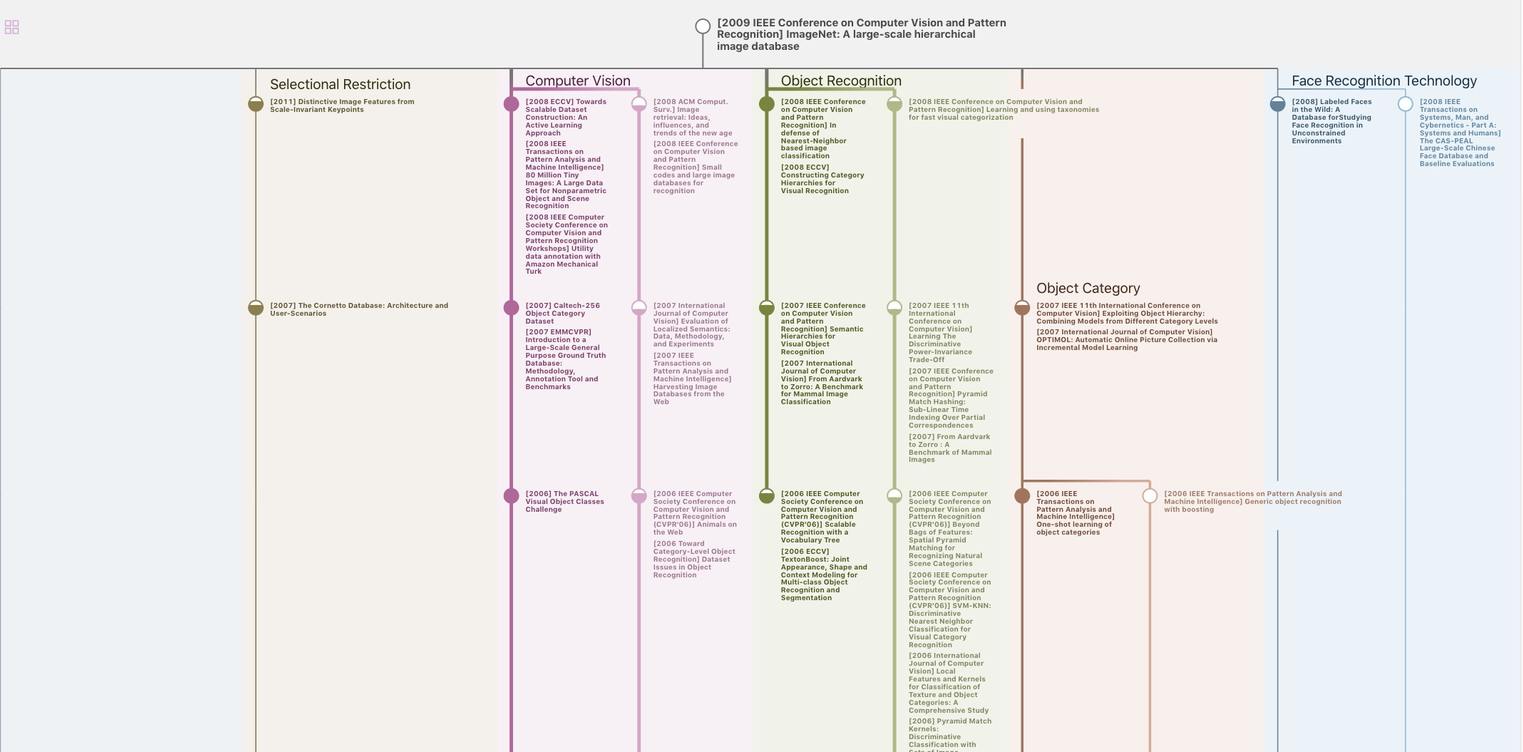
生成溯源树,研究论文发展脉络
Chat Paper
正在生成论文摘要