Diagnosis for Reconfiguration
semanticscholar(2019)
摘要
Many domains require scalable algorithms that help to determine diagnoses efficiently and often within predefined time limits. Anytime diagnosis is able to determine solutions in such a way and thus is especially useful in real-time scenarios such as production scheduling, robot control, and communication networks management where diagnosis and corresponding reconfiguration capabilities play a major role. Anytime diagnosis in many cases comes along with a trade-off between diagnosis quality and the efficiency of diagnostic reasoning. In this paper we introduce and analyze FLEXDIAG which is an anytime direct diagnosis approach. We evaluate the algorithm with regard to performance and diagnosis quality using a configuration benchmark from the domain of feature models and an industrial configuration knowledge base from the automotive domain. Results show that FLEXDIAG helps to significantly increase the performance of direct diagnosis search with corresponding quality tradeoffs in terms of minimality and accuracy. Alexander Felfernig Applied Software Engineering Group, Institute for Software Technology, TU Graz, Austria E-mail: alexander.felfernig@ist.tugraz.at Rouven Walter Symbolic Computation Group, WSI Informatics, Universität Tübingen, Germany E-mail: rouven.walter@uni-tuebingen.de José A. Galindo Computer Languages and Systems Department, University of Sevilla, Spain E-mail: jagalindo@us.es David Benavides Computer Languages and Systems Department, University of Sevilla, Spain E-mail: benavides@us.es Seda Polat-Erdeniz Applied Software Engineering Group, Institute for Software Technology, TU Graz, Austria E-mail: spolater@ist.tugraz.at Müslüm Atas Applied Software Engineering Group, Institute for Software Technology, TU Graz, Austria E-mail: muatas@ist.tugraz.at Stefan Reiterer SelectionArts, Austria E-mail: stefan.reiterer@selectionarts.com 2 A. Felfernig et al.
更多查看译文
AI 理解论文
溯源树
样例
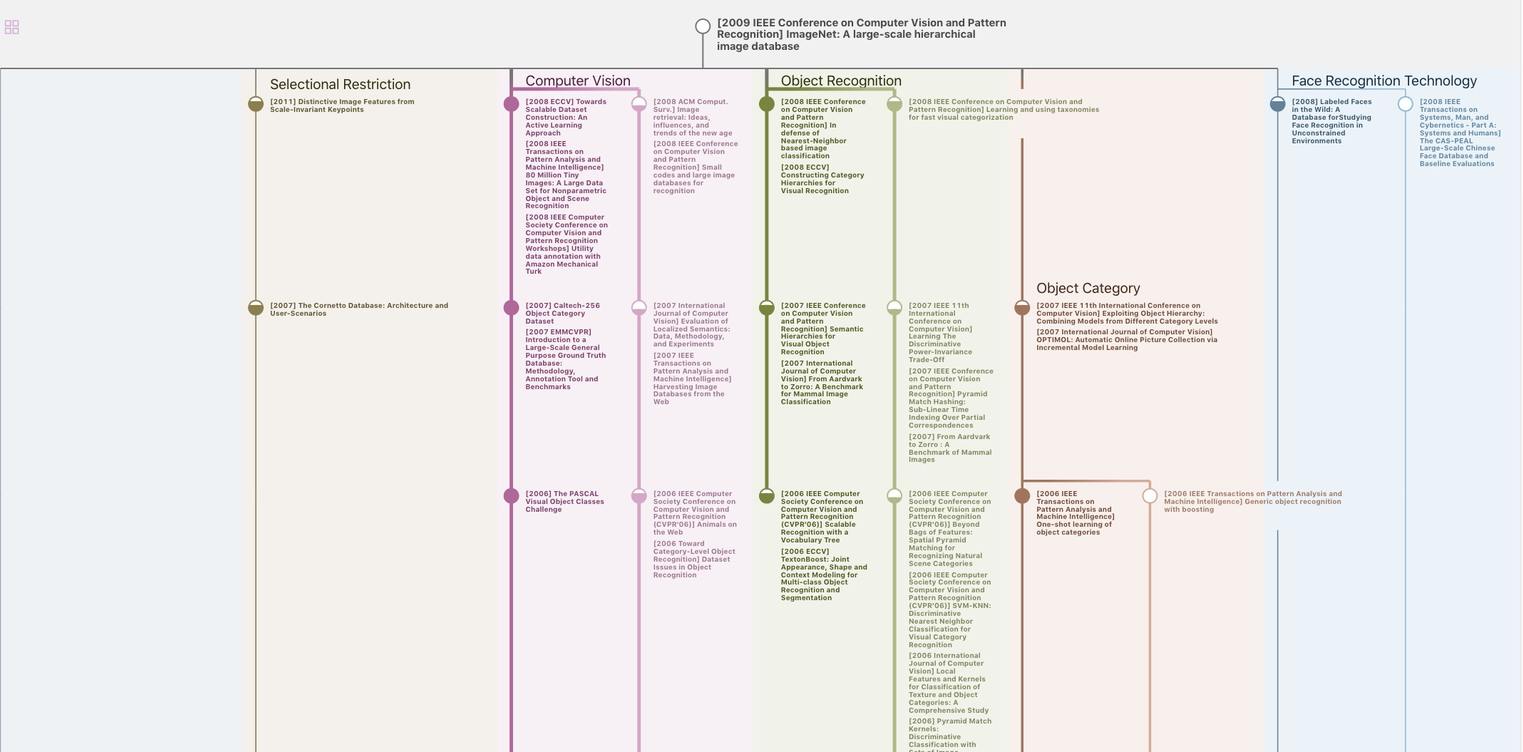
生成溯源树,研究论文发展脉络
Chat Paper
正在生成论文摘要