Scalable Real-Time and One-Shot Multiple-Affordance Detection
semanticscholar(2019)
摘要
This paper develops and evaluates a geometrydriven approach that allows for the detection of affordances in an scalable and multiple-instance manner. True to Gibson’s idea of direct and economical perception, our approach requires little supervision, is straightforward to compute and is agnostic to semantics. The proposed approach is trained with a single interaction example on synthetic data (i.e. simulated), yet is able to generalise to previously unknown RGB-D scenarios without further training. Furthermore, our use of geometric information not only allows to detect what a location in the environment affords but also how it affords, i.e. object-pose. We show results from several dozens of affordances (80+) predicted simultaneously at high frame rates on indoor environments such as kitchens and offices. Our evaluations show high rates of precision and that the algorithm’s predictions align well with crowd-sourced human validations.
更多查看译文
AI 理解论文
溯源树
样例
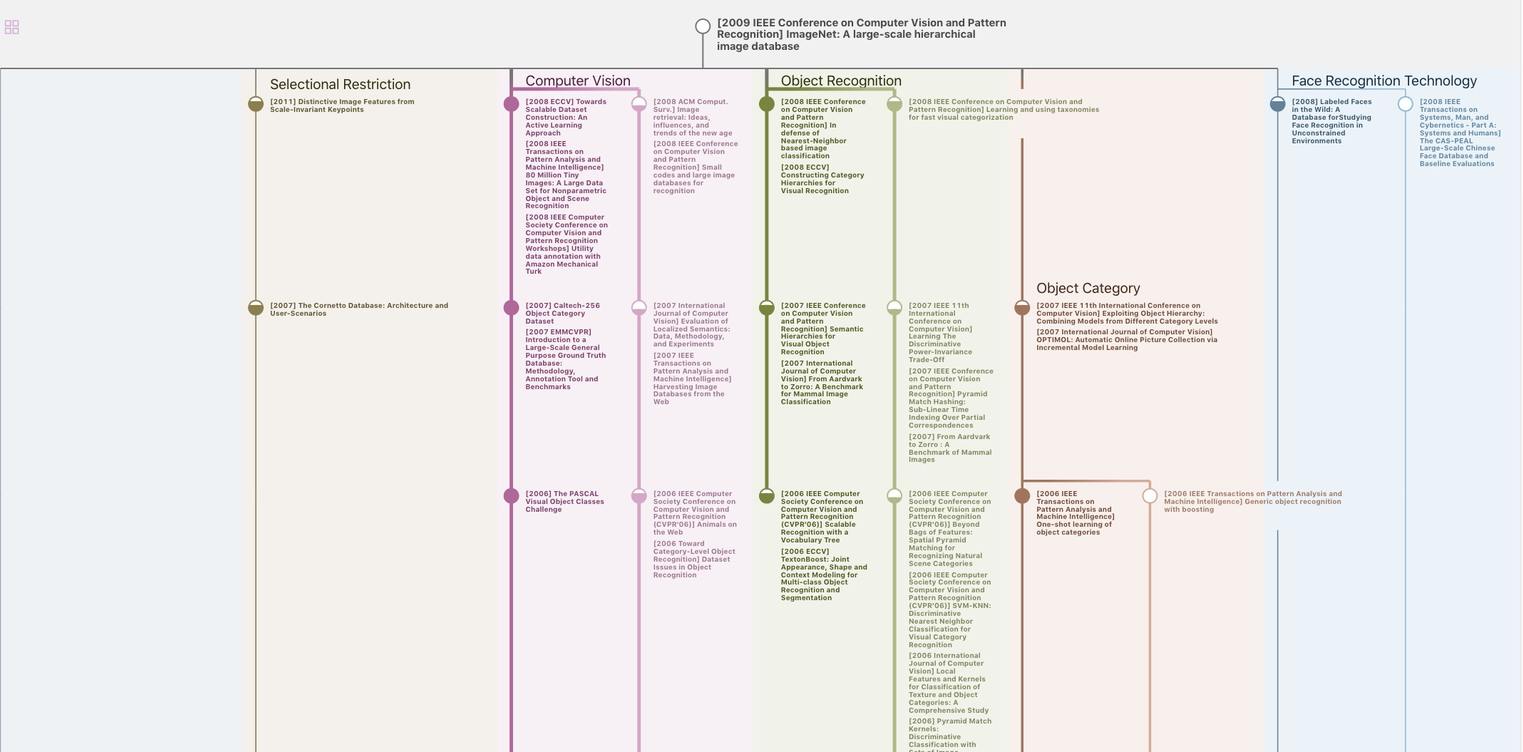
生成溯源树,研究论文发展脉络
Chat Paper
正在生成论文摘要