Latent Allocation Spatiotemporal Models For Indoor Human Mobility
semanticscholar(2018)
摘要
The ability to understand, model and predict human mobility in high-occupancy buildings like corporate offices and campuses can fundamentally change the way buildings are managed. For example, energy efficiency can be improved using more accurate models of the temporal and spatial aspects of building occupancy. Similarly, responding to emergency situations is more effective and less intrusive if the building system has better knowledge not just of where occupants are, but also of their likely next locations and when they will get there. We propose a novel approach to learn a spatiotemporal model of human mobility from observed trajectories. Our approach posits the existence of different mobility profiles that reflect the heterogeneity in the way people move between locations. Our proposed latent allocation model describes the probabilistic relationships between the observed trajectory data and the latent (unobserved) mobility profiles and their parameters. To tackle the problem of inferring these parameters efficiently, we frame the model as a neural network. We engineer the layers of the network to enforce appropriate constraints on the learned spatial and temporal parameters of each profile to best explain the data. We demonstrate our model and learning approach on synthetic data and give initial results from a subset of real data collected from our corporate building. ACM Reference Format: Yiming Gu, Hala Mostafa and B. Aditya Prakash. 2018. Latent Allocation Spatiotemporal Models For Indoor Human Mobility . In UrbComp 2018, August 2018, London, United Kingdom. ACM, New York, NY, USA, Article 4, 9 pages. https://doi.org/10.1145/nnnnnnn.nnnnnnn
更多查看译文
AI 理解论文
溯源树
样例
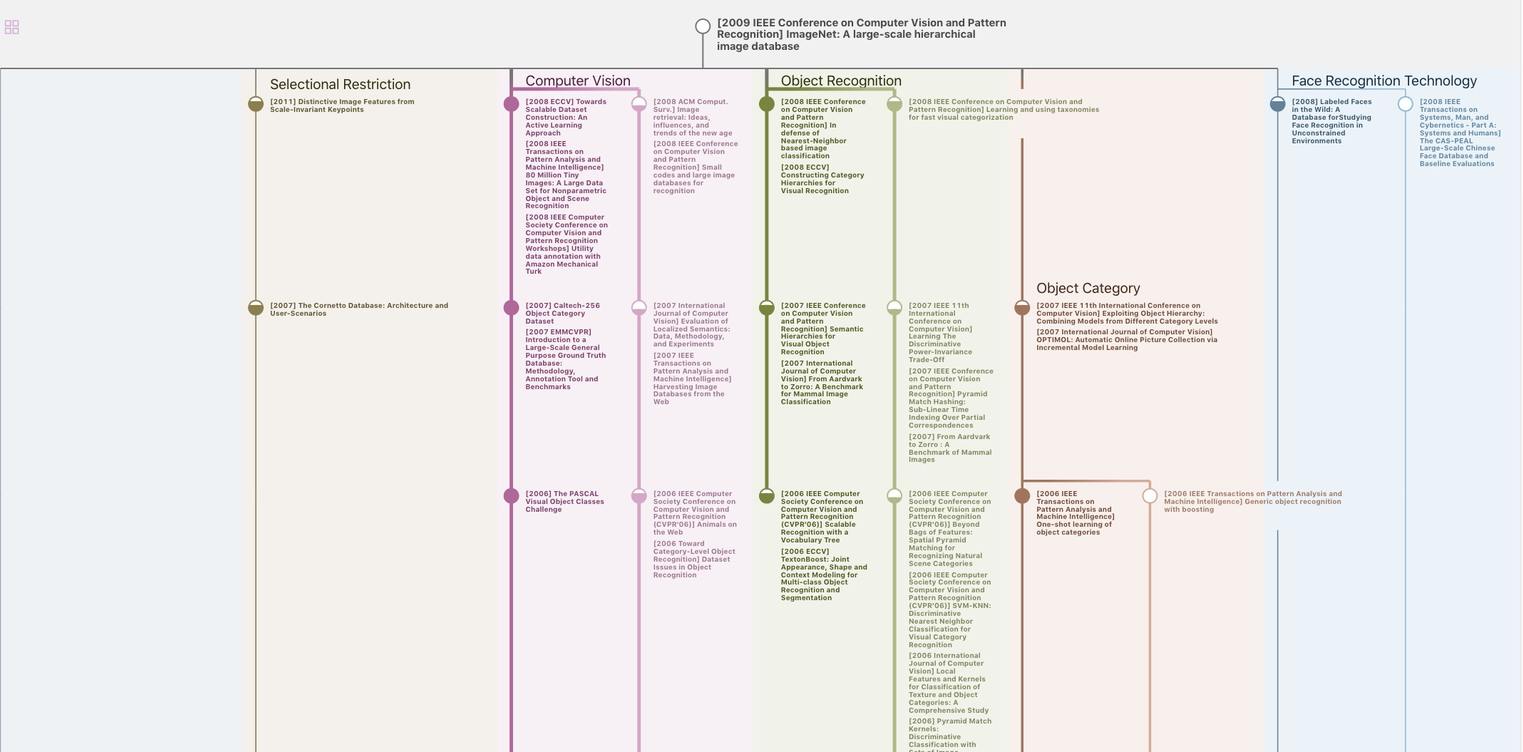
生成溯源树,研究论文发展脉络
Chat Paper
正在生成论文摘要