Threat Analytics and Visualization Solution for Big Security Data
semanticscholar(2016)
摘要
How can organisations make use of the large amounts of security data collected by Security Information and Event Management (SIEM) systems to identify malicious activity? We present our work on analysis of Domain Name System (DNS) data collected from Hewlett Packard Labs' DNS servers over several months. Our solution combines novel visualization and analytic techniques. A working prototype is being used in ongoing investigations with HP Labs IT and HP GCS (Global Cyber Security) Cyber Defense Center (CDC). Our solution has been successfully validated in pilots. This paper describes our work carried out in 2013-2014, that was transferred to Hewlett Packard Enterprise (HPE) SW/ArcSight Security Business in 2015 and productized, as Hewlett Packard DNS Malware Analytics (DMA). External Posting Date: December 14, 2016 [Fulltext] Internal Posting Date: December 14, 2016 [Fulltext] Copyright 2016 Hewlett Packard Enterprise Development LP 1 Threat Analytics and Visualization Solution for Big Security Data Yolanta Beresna, Marco Casassa Mont Hewlett Packard Enterprise {yolanta.beres, marco.casassa-mont}@hpe.com Abstract How can organisations make use of the large amounts of security data collected by Security Information and Event Management (SIEM) systems to identify malicious activity? We present our work on analysis of Domain Name System (DNS) data collected from Hewlett Packard Labs’ DNS servers over several months. Our solution combines novel visualization and analytic techniques. A working prototype is being used in ongoing investigations with HP Labs IT and HP GCS (Global Cyber Security) Cyber Defense Center (CDC). Our solution has been successfully validated in pilots. This paper describes our work carried out in 2013-2014, that was transferred to Hewlett Packard Enterprise (HPE) SW/ArcSight Security Business in 2015 and productized, as Hewlett Packard DNS malware Analytics (DMA) [8]. 1. Problem statement The key problem addressed in our work is to help organizations identify sophisticated security threats and attacks, by analyzing large amounts of security data, collected as event logs or as network packets, and to ensure that these findings are actionble by security analysts dealing with such threats (for example in Cyber Defense Centers), without requiring these analysts to have deep data science expertise. The security domain poses unique challenges in terms of (big) data analytics and visualization as compared to traditional Business Intelligence analytics: it requires detection of relatively rare events – attacks within huge amounts of data. Many organizations use Security Information and Event Management (SIEM) solutions to collect security events from a wide variety of systems and logs. These solutions primarily focus on real-time alerting tools, optimized to do event-driven signature matching for a subset of the overall collected data. As attacks become more sophisticated, threats are harder to detect just with signature-based systems: there is an emerging need to complement these solutions with more extensive analytics, to identify major anomalies, combine multiple indicators of attackers’ activities, and detect customised malware infections. What makes the problem of identifying malicious behavior hard is that we don’t always know what we are looking for: not only must the behavior be anomalous but it must also be an attack, and we do not know signatures upfront for all attacks. Therefore, it is important to present findings to CDC analysts for human-driven analysis. These analysts may not be data science experts, so to make the security data analytics easier to interpret, we use visual data representation and visual analytics, tailored specifically for security data. Achieving this demands much at the infrastructure level (streaming data from collectors to large data stores), the analysis level (dealing with a variety of analytics and designing analytics that scale well) and at the data presentation/visualization level. From a business perspective Hewlett Packard Enterprise (HPE) already has core, point solutions in this space: ArcSight (SIEM), Vertica and Autonomy, some of which are part of HPE HAVEn [2] initiative. However, at the time of doing this work, HPE had no security specific commercial offerings to complement the real-time SIEM solution with tools that can analyse past data. Our competitors already had compelling products in this space: however, existing solutions tend to require deep data science expertise by security analysts.
更多查看译文
AI 理解论文
溯源树
样例
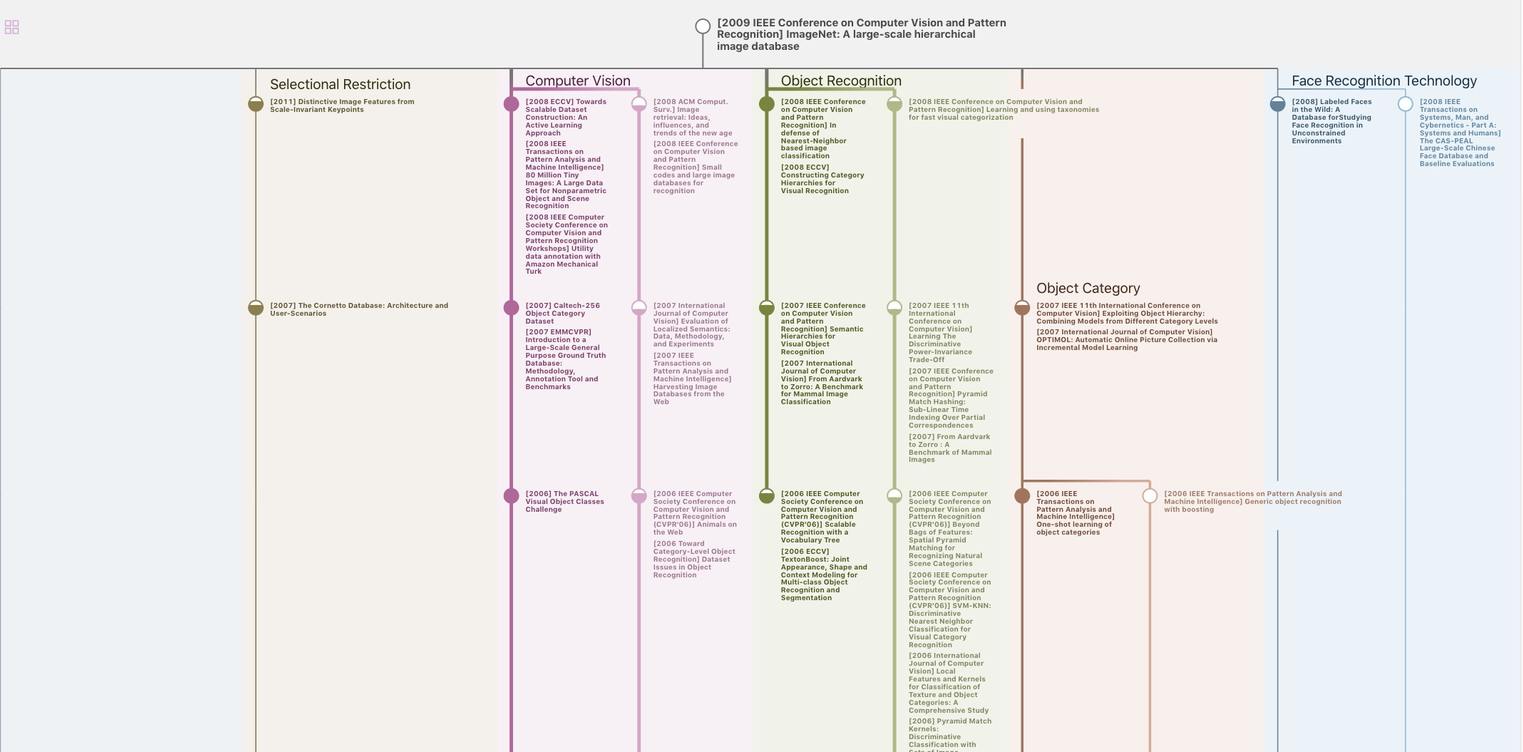
生成溯源树,研究论文发展脉络
Chat Paper
正在生成论文摘要