An atlas of variant impact maps for human disease genes
semanticscholar(2018)
摘要
Background: One of the most surprising results to emerge from genome-wide association studies (GWAS) is 95% of all disease associated single nucleotide polymorphisms (SNPs) identified by this method reside in non-coding regions of the genome. Despite this finding, non-coding SNPs remain hugely understudied, due in part to the uncertain functional consequences of such mutations. However, a large proportion of these SNPs reside within regulatory regions of the genome, such as transcription factor binding sites (TFBSs). TFBSs only cover 8.1% of the genome, yet they contain 31% of GWAS SNPs. SNPs in these binding sites may alter the binding affinity of transcription factors, leading to changes in downstream gene expression, and ultimately human disease. Here, we propose a novel screening tool, SEMpl, which estimates transcription factor (TF) binding affinity to better predict disease causing SNPs in TFBSs. Methods: SEMpl generates its predictions through observation of existing variants in TFBSs genome-wide using publically available data from the ENCODE database to generate SNP effect matrixes (SEMs). SEM scores represent the predicted change in binding affinity from average binding of the target TF. Results: SEMpl has demonstrated a better correlation with experimental estimates of TF binding affinity than the current standard, position weight matrices (PWMs). Significance: We hypothesize that SEMpl scores will allow researchers to better predict disease causing SNPs in TFBSs genome wide. Predicting the impact of genetic variants with BioFolD tools Emidio Capriotti* Department of Pharmacy and Biotechnology (FaBiT), University of Bologna. Via F. Selmi 3. 40126 Bologna (Italy) email: emidio.capriotti@unibo.it During the last few years we developed several tools for predicting the impact of genetic variants at protein and nucleotide levels. The implemented methods are characterized by the types and number used for discriminating between pathogenic and benign variants. The simplest algorithm is PhD-SNP (Capriotti, et al., 2006), which is a support vector machine based method that takes in input only sequence-based extracted from the protein sequence profile. The most complex tool is SNPs&GO (Capriotti, et al., 2013b) which includes in the input features functional information encoded by Gene Ontology terms and, when available, protein structure features. More recent algorithms such as Meta-SNP (Capriotti, et al., 2013a) implements a meta prediction method combining 4 well-establish methods while PhD-SNP (Capriotti and Fariselli, 2017) uses the information retrieved on the UCSC genome browser to predict the impact of variants in non coding regions. During the last edition of the CAGI we used modified version of these methods to predict the impact of the variants released for four challenges, namely the Cell-Cycle-Checkpoint Kinase 2 (CHEK2), the Acid Alpha-Glucosidase (GAA), the Calmodulin 1 (CALM1) and the Pericentriolar Material 1 (PCM1). Among these challenges we verified that PhD-SNP reached a good level of performances in the prediction of the fraction of tumor cases associated to a set of variants in the CHEK2 protein. In particular on a set composed by 34 coding CHEK2 variants PhD-SNP achieved a balanced accuracy of 0.71 a Matthews’ Correlation Coefficient of 0.41 and an Area Under the Curve (AUC) of 0.72. All the tools used for the CAGI challenges are available at http://snps.biofold.org/
更多查看译文
AI 理解论文
溯源树
样例
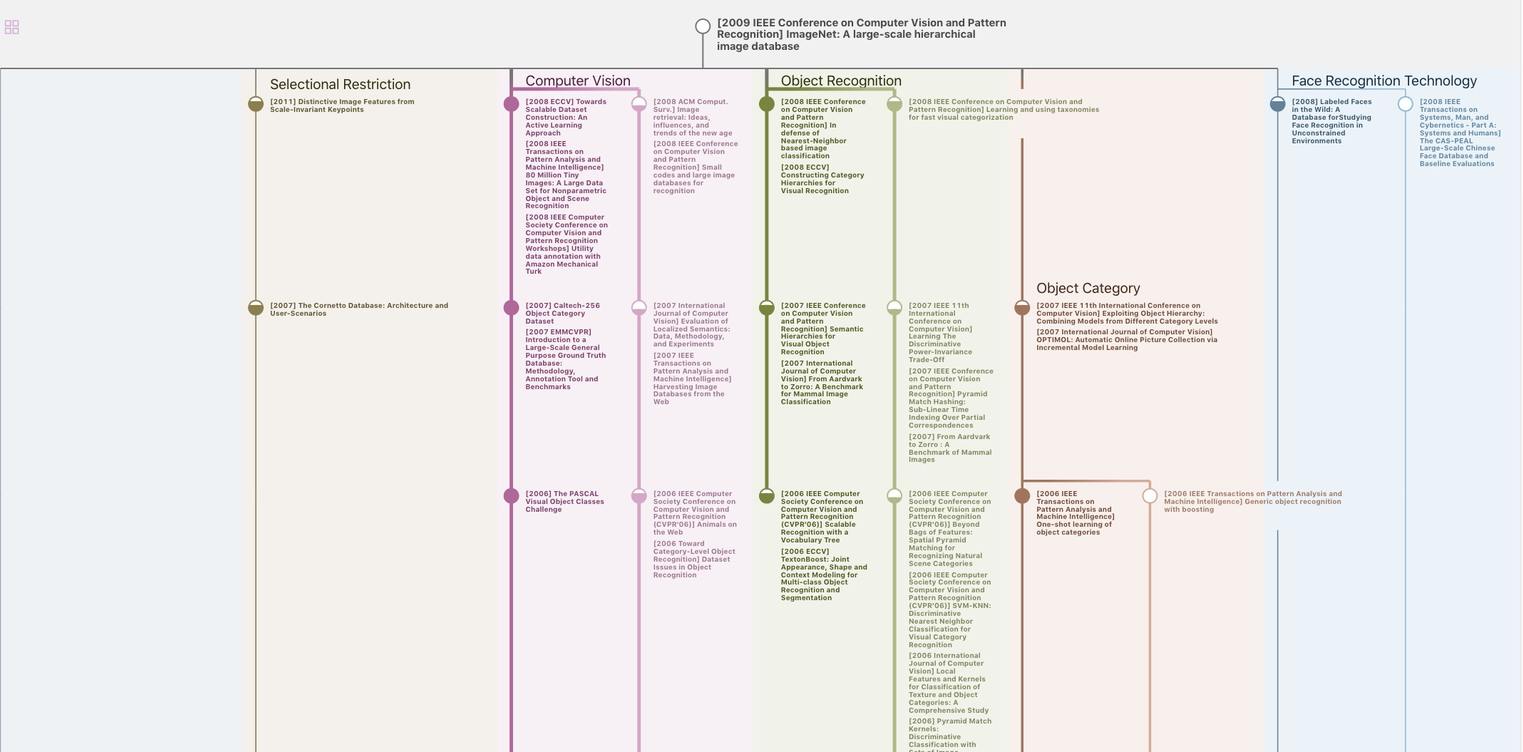
生成溯源树,研究论文发展脉络
Chat Paper
正在生成论文摘要