Automatic Classification Overcoming Network Dependency Measures
semanticscholar(2017)
摘要
The human brain as a complex network is composed of neurons and synapses, forming a network structure. Several studies link the brain cognitive functions to its network structure, and various neurological disorders happen due to an interruption in the functional brain networks. To study the functional brain networks, the first step is to extract the connectivity matrices, and binarize them. Different methods can be used to construct a connectivity matrix and binarize it to reduce the noise level. In this study, we use correlation, mutual information, and phase lag index to evaluate the connectivity matrices. In order to perform binarization, we used a robust tree representation called Minimum Spanning Tree (MST). Studying topological characteristics of infants’ dense-array EEG data across two groups of infants including 6-month-olds with family history (FH+) of Language-based Learning Disorder (LLD), and control groups (FH-), we found that detection of interruption in the network topologies highly depend on the method of connectivity analysis, leading to different results. Therefore, we suggest using Support Vector Machine (SVM) classification and a combination of topological properties gained from different analyses as inputs of the ∗Corresponding author Email addresses: pashakhanloo@ce.sharif.edu (Pardis Pashakhanloo), marziehzare@ipm.ir (Marzieh Zare), benasich@andromeda.rutgers.edu (April A. Benasich), azad@sharif.edu (Hamid Sarbazi-Azad) Preprint submitted to Journal of LTEX Templates January 25, 2017 machine to improve the ability to separate individuals into risk groups.
更多查看译文
AI 理解论文
溯源树
样例
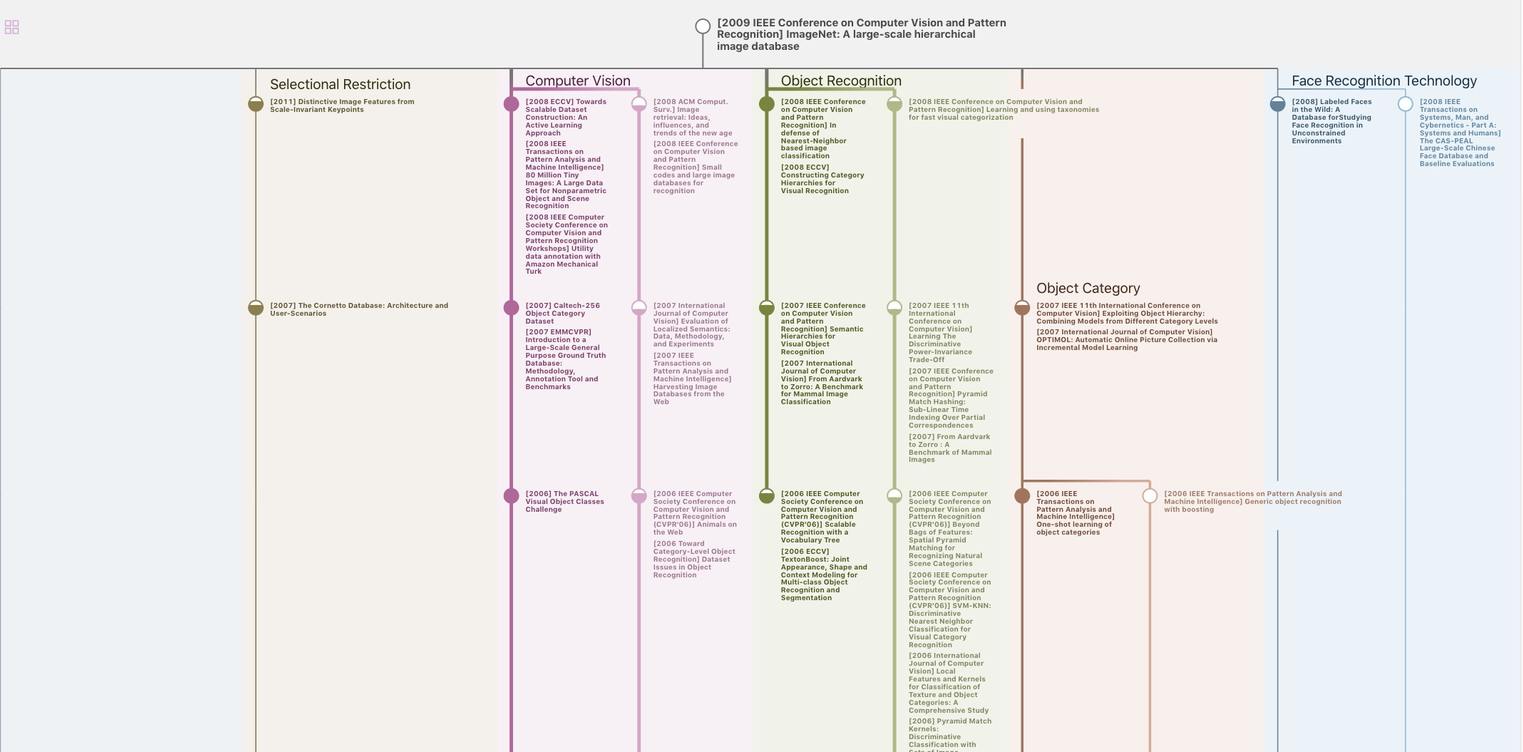
生成溯源树,研究论文发展脉络
Chat Paper
正在生成论文摘要