Safe Exploration for Reinforcement Learning in Real Unstructured Environments
semanticscholar(2015)
摘要
In USAR (Urban Search and Rescue) missions, robots are often required to operate in an unknown environment and with imprecise data coming from their sensors. However, it is highly desired that the robots only act in a safe manner and do not perform actions that could probably make damage to them. To train some tasks with the robot, we utilize reinforcement learning (RL). This machine learning method however requires the robot to perform actions leading to unknown states, which may be dangerous. We develop a framework for training a safety function which constrains possible actions to a subset of really safe actions. Our approach utilizes two basic concepts. First, a “core” of the safety function is given by a cautious simulator and possibly also by manually given examples. Second, a classifier training phase is performed (using Neyman-Pearson SVMs), which extends the safety function to the states where the simulator fails to recognize safe states.
更多查看译文
AI 理解论文
溯源树
样例
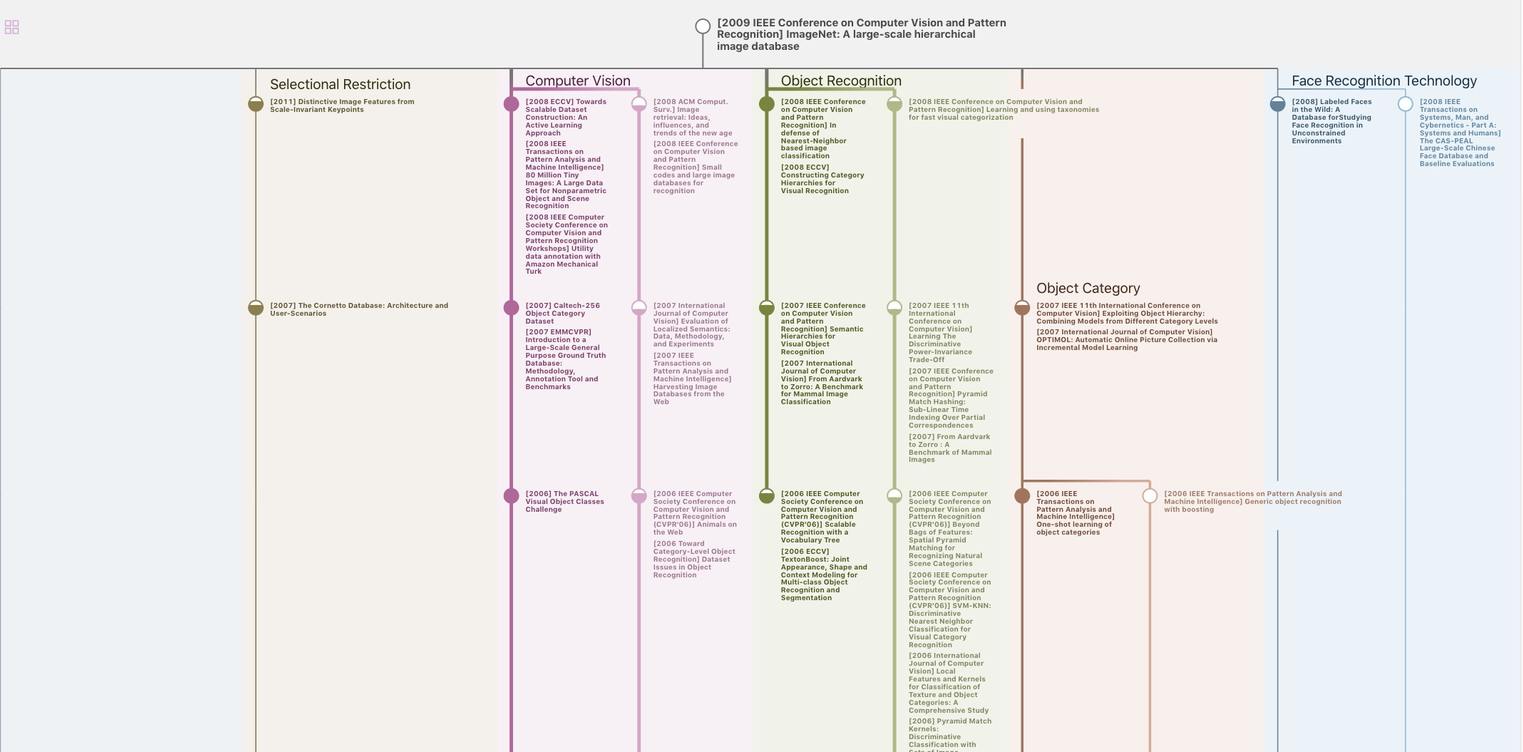
生成溯源树,研究论文发展脉络
Chat Paper
正在生成论文摘要