Relational Bayesian models of on-line user behavior
semanticscholar(2003)
摘要
We examine the utility of relational probabilistic methods for modeling user behavior at web sites. Web logs (aka "click streams"), taken as datasets for traditional machine learning algorithms, violate the iid assumption of most algorithms. Requests ("clicks") are not independent within a session, sessions for a visitor are not independent of one another, and page types, in their interaction with behavioral profile, are highly correlated, both by static link structure and dynamic navigation sequence. We compare a series of increasingly sophisticated models, ranging from a simple, non-relational Bayesian network model of an individual click through traditional HMMs to fully relational extensions of HMMs, including visitors, sessions, clicks, and pages as participating entities. We measure the performance of the series of models on the task of predicting whether or not the current request is the last in a session. Results show a smooth and significant increase in performance, as measured by ROC AUC (area under the curve).
更多查看译文
AI 理解论文
溯源树
样例
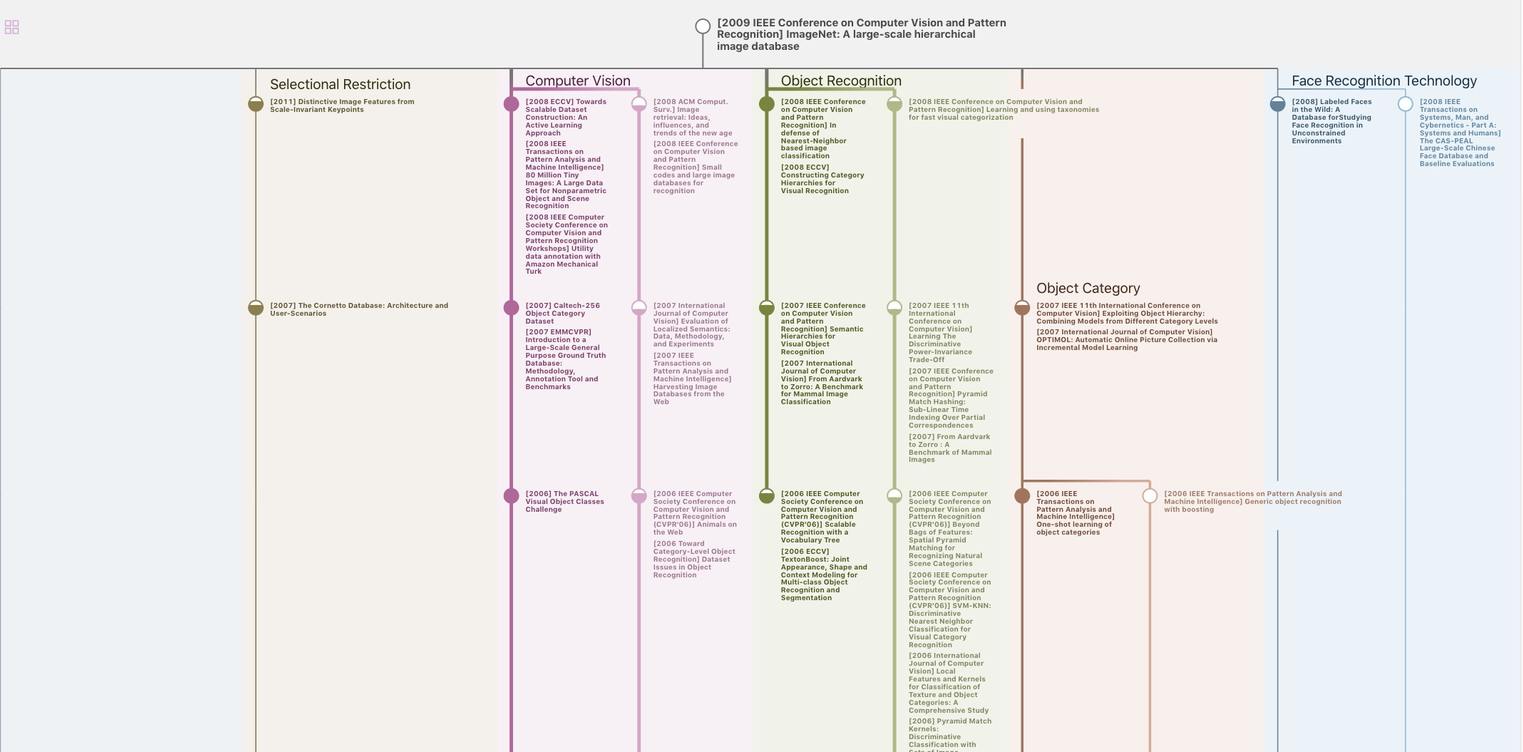
生成溯源树,研究论文发展脉络
Chat Paper
正在生成论文摘要