Learning Human Reconstruction from Synthetic Dataset
semanticscholar(2019)
摘要
Existing stereo reconstruction methods for narrow baseline image pairs [2, 4] give limited performance for wide baseline views. This paper proposes a framework to learn and estimate dense stereo for people from wide baseline image pairs. A synthetic people stereo patch dataset (S2P2) is introduced to learn wide baseline dense stereo matching for people. The proposed framework learns human specific features from synthetic data for patch match and adapts it to real data. In addition to patch match learning, a stereo constraint is introduced in the framework to solve wide baseline stereo reconstruction of humans. Quantitative and qualitative performance evaluation of the proposed approach against state-of-the-art methods demonstrates improved wide baseline stereo reconstruction on challenging datasets. We show that it is possible to learn stereo matching from synthetic people dataset and improve performance on real datasets for stereo reconstruction of people from wide baseline stereo data.
更多查看译文
AI 理解论文
溯源树
样例
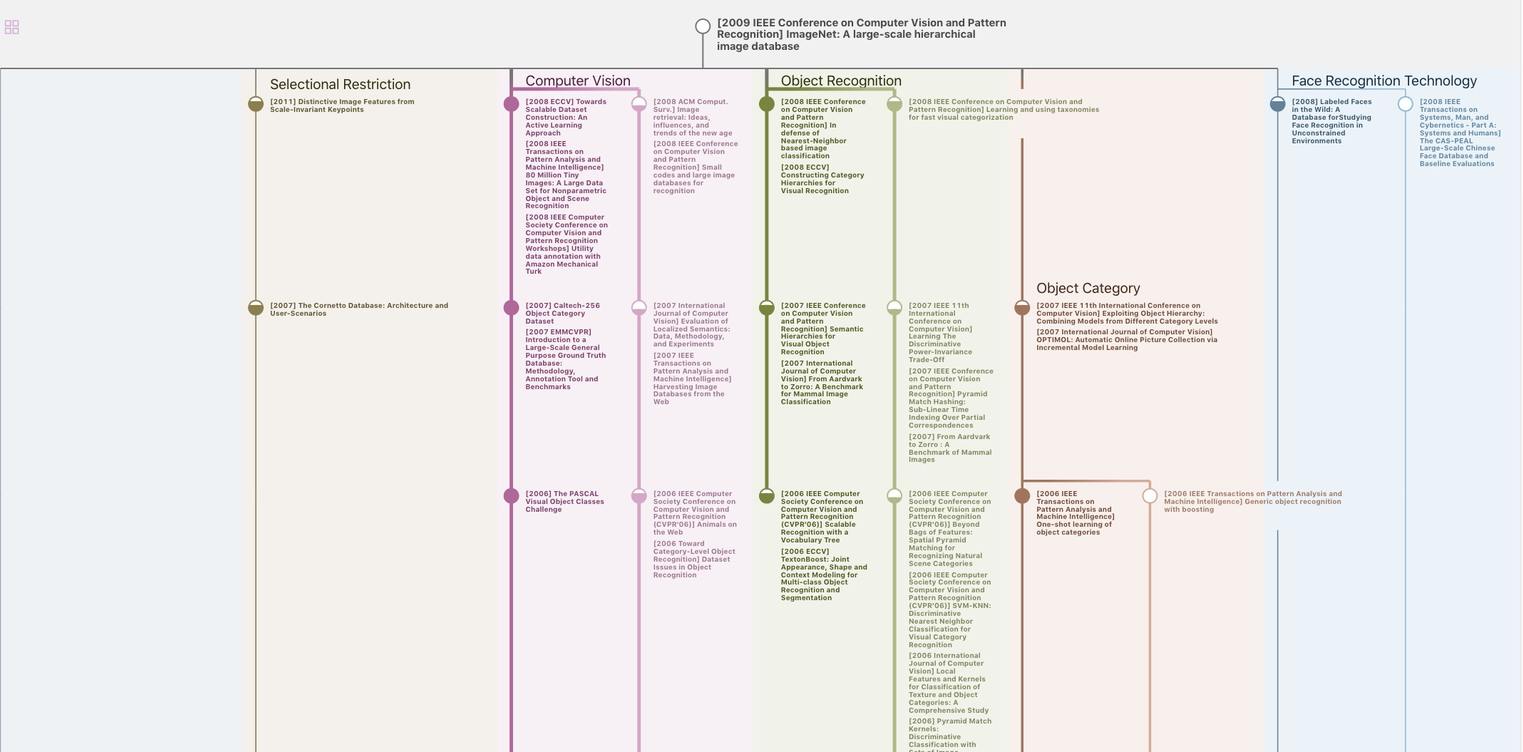
生成溯源树,研究论文发展脉络
Chat Paper
正在生成论文摘要