Error bound for Slope SVM in High Dimension
semanticscholar(2017)
摘要
In this paper, we propose a new estimator: the Slope SVM, which minimizes the hinge loss with the Slope penalization introduced by [3]. We study the asymptotical behavior of the `2 error between the theoretical hinge loss minimizer and the Slope estimator. We prove Slope achieves a (k/n) log(p/k) rate with high probability and in expectation under the Weighted Restricted Eigenvalue Condition. This bound is similar to the exact minimax one for regression and, to the best of our knowledge, it is the best achievable for a classification estimator.
更多查看译文
AI 理解论文
溯源树
样例
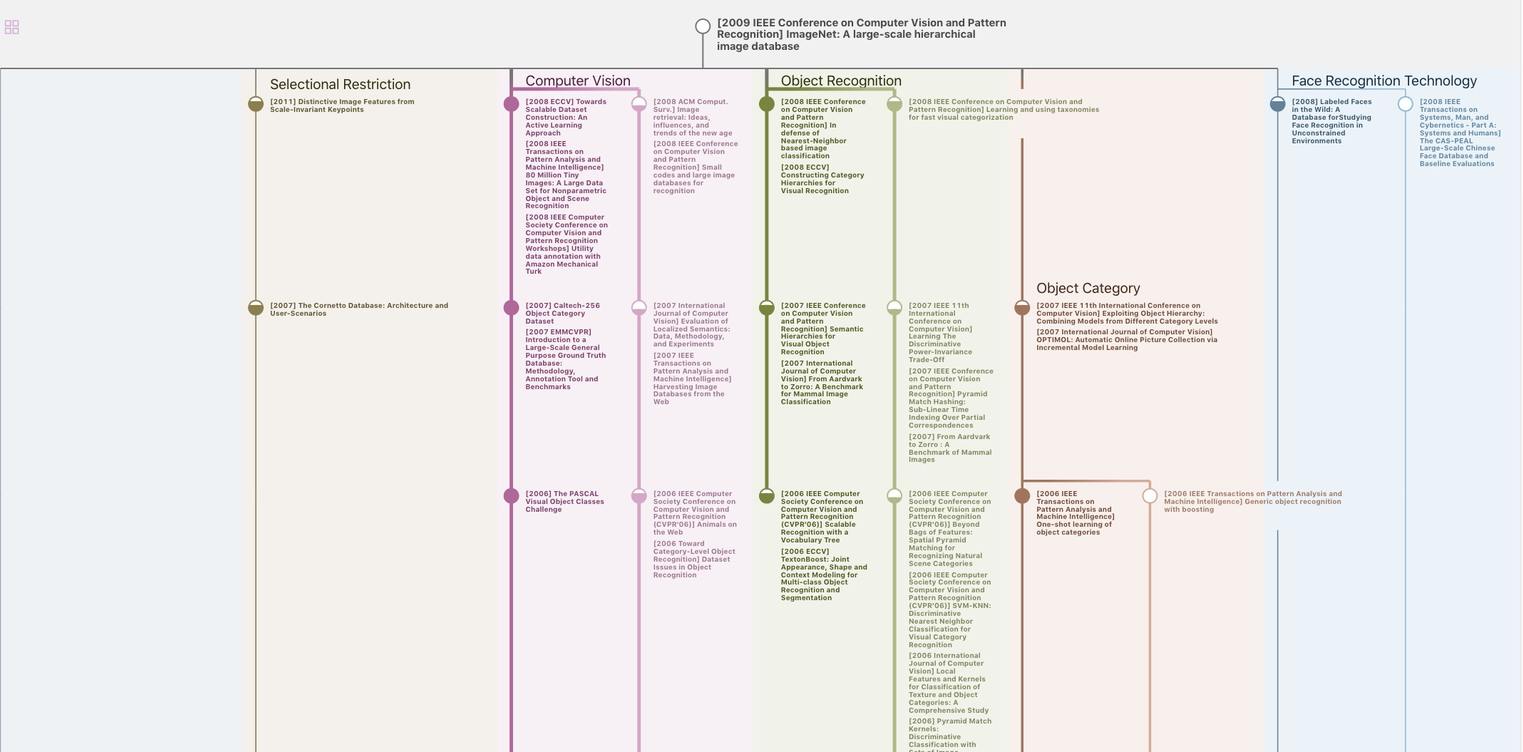
生成溯源树,研究论文发展脉络
Chat Paper
正在生成论文摘要