Dynamics of learning in feature discovery networks
semanticscholar(2017)
摘要
We address the dynamics of learning in neural feature-discovery networks. The models introduced incorporate feed-forward connections modified by a Hebb law, and recurrent lateral connections modified by an anti-Hebb law. The stability of equilibria depends on both the learning rates in the system, and the second order statistics of the ensemble of inputs. We derive conditions for stability of equilibria, and use bifurcation theory to explore the behavior near loss of stability. The bifurcation analysis uncovers previously overlooked behaviors, including equilibria that consist of mixtures of the principal eigenvectors of the input auto-correlation, as well as limit cycles. The results provide a more complete picture of adaptation in Hebbian feature-discovery networks. 'This work was supported by the Office of Naval Research under contracts N00014-88K-0329 and N00014-90-1349 and by DARPA grant MDA 972-88-5-1004.
更多查看译文
AI 理解论文
溯源树
样例
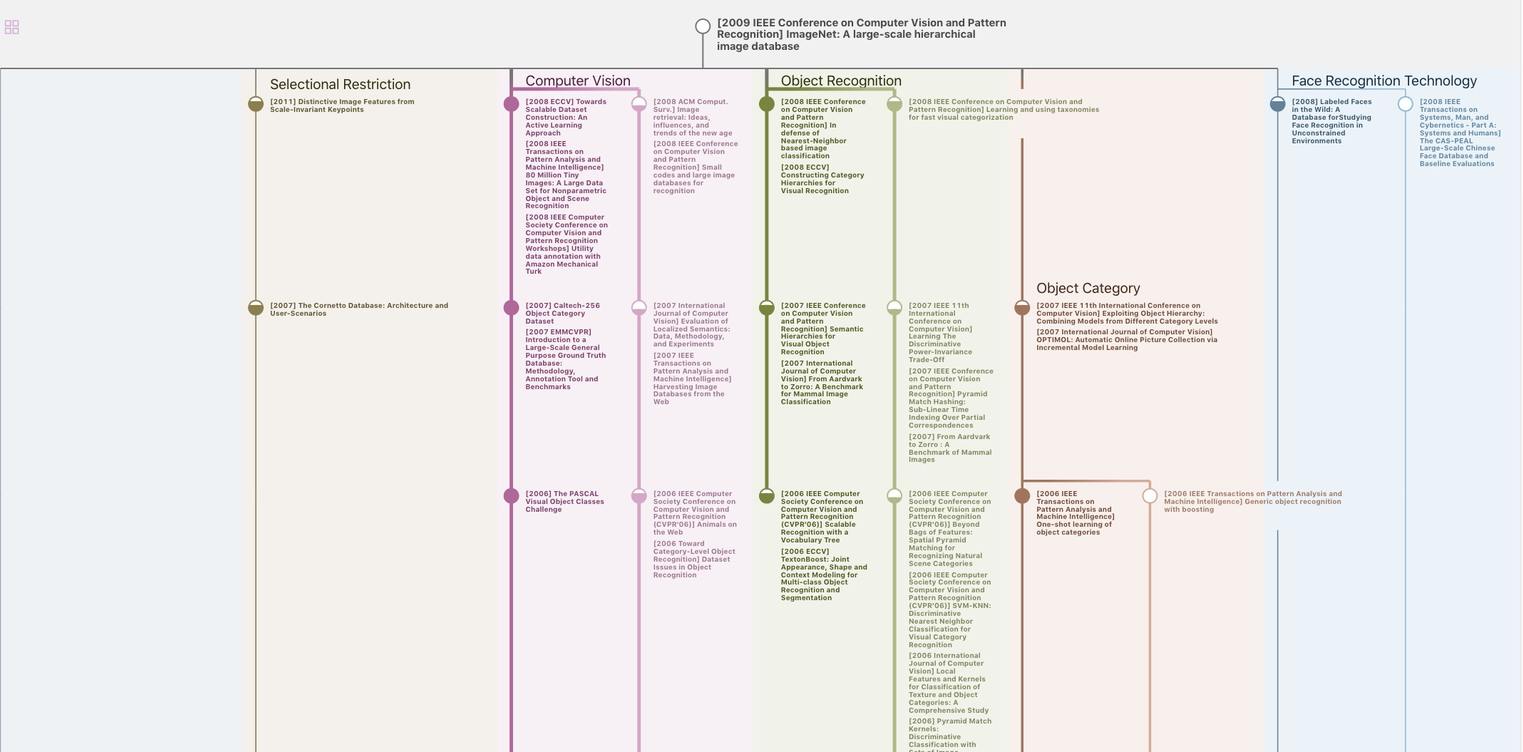
生成溯源树,研究论文发展脉络
Chat Paper
正在生成论文摘要