Seafloor Exploration using the Linearised Differential Entropy of Gaussian Process Classifiers
semanticscholar(2015)
摘要
While seafloor bathymetry have been mapped extensively over the last few decades, geological and ecological observations of seafloor benthic zones only began recently. Unlike bathymetric mapping, data collection of benthic imagery requires in situ exploration a significantly slower and costly endeavour. An efficient exploration policy would therefore require solving the informative path-planning problem. This paper investigates a receding horizon approach to the informative path-planning problem using linearised differential entropy as the proposed acquisition function. We model the benthic environment using five bathymetric features through Gaussian process classifiers, whose linearised differential entropy (LDE) would be defined and derived. We demonstrate that LDE acquisition achieves the lowest misclassification rate under a receding horizon formulation, outperforming acquisition under both Monte Carlo based joint information entropy and marginalised information entropy. In particular, it avoids the time complexity of Monte Carlo sampling, while still retaining a measure of mutual information. We also show the benefits of a receding horizon approach over simpler techniques such as greedy and open loop methods. Finally, we test our method on collected benthic datasets from past AUV missions to Scott Reef, Western Australia.
更多查看译文
AI 理解论文
溯源树
样例
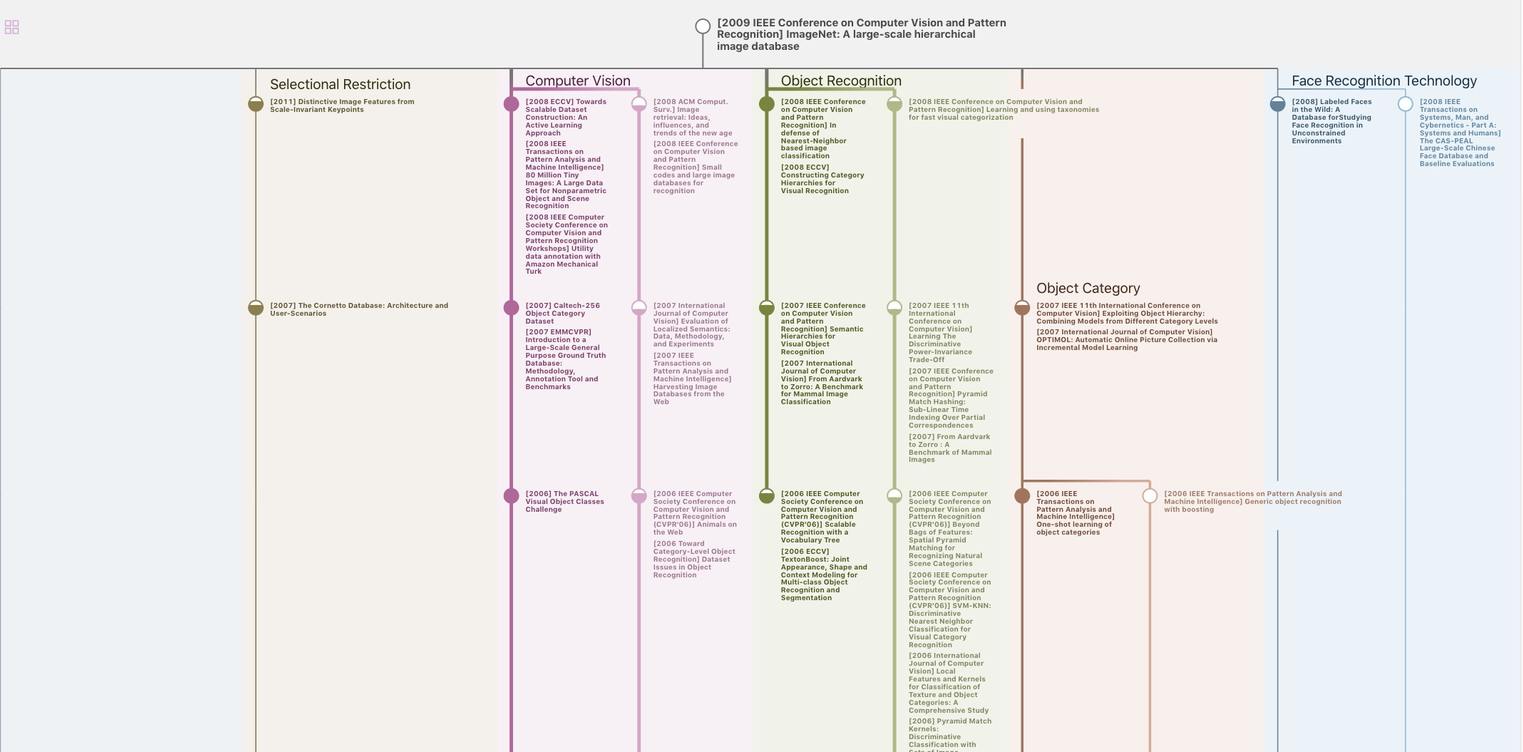
生成溯源树,研究论文发展脉络
Chat Paper
正在生成论文摘要