Learning with Hypernode Graphs Spectral Learning with Hypernode Graphs Application to Skill Rating for Multiple Players Games ∗
semanticscholar(2015)
摘要
The aim of this paper is to propose methods for learning from interactions between groups in networks. For this, we introduce hypernode graphs as a formal tool able to model group interactions. A hypernode graph is a set of weighted binary relations between disjoint sets of nodes. We define Laplacians and kernels for hypernode graphs. And we propose spectral learning algorithms over hypernode graphs allowing to infer node ratings or node labelings. As a proof of concept, we model multiple players games with hypernode graphs and we define skill-rating learning algorithms. We apply these algorithms on multiple players games and obtain very promising results compared to Elo Duelling and Trueskill. We then study the expressive power of hypernode graphs and the associated spectral theory. That is, we prove that hypernode graph kernels strictly generalize over graph kernels. Also, we prove that hypernode graphs correspond to signed graphs such that the matrix D −W is positive semidefinite. This shows that homophilic relations between groups may lead to non homophilic relations between individuals. Finally, we define the notion of connected hypernode graphs and we define a resistance distance generalizing over the resistance distance for graphs.
更多查看译文
AI 理解论文
溯源树
样例
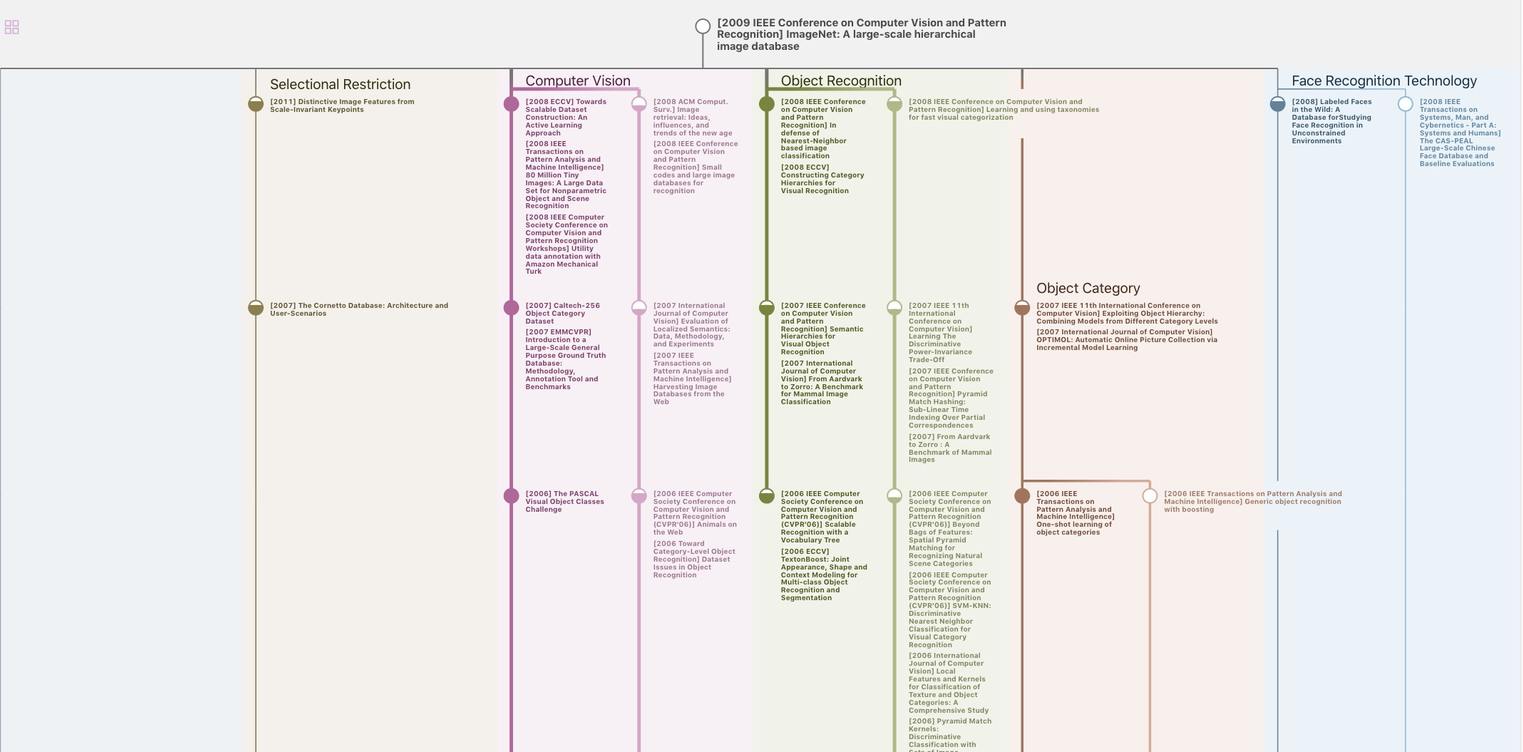
生成溯源树,研究论文发展脉络
Chat Paper
正在生成论文摘要