Learning Graphical Structure of Electronic Health Records with Transformer for Predictive Healthcare
semanticscholar(2019)
摘要
Effective modeling of electronic health records (EHR) is rapidly becoming an important topic in both academia and industry. A recent study showed that utilizing the graphical structure underlying EHR data improves the performance of prediction tasks such as heart failure diagnosis prediction. However, EHR data do not always contain the complete structural information. Moreover, when it comes to claims data, they do not have any structural information to begin with. Under such circumstances, can we still do better than just treating EHR data as a flat-structured bag-of-features? In this paper, we study the possibility of utilizing the implicit structure of EHR by using Transformer for prediction tasks on public electronic health records. Specifically, we make a connection between graph networks and Transformer, then use a variant of Transformer on encounter-based prediction tasks such as medication prediction and masked node prediction. Our model empirically demonstrates superior prediction performance to previous approaches on two publicly available EHR datasets, indicating that it can serve as an effective general-purpose representation learning algorithm for EHR data.
更多查看译文
AI 理解论文
溯源树
样例
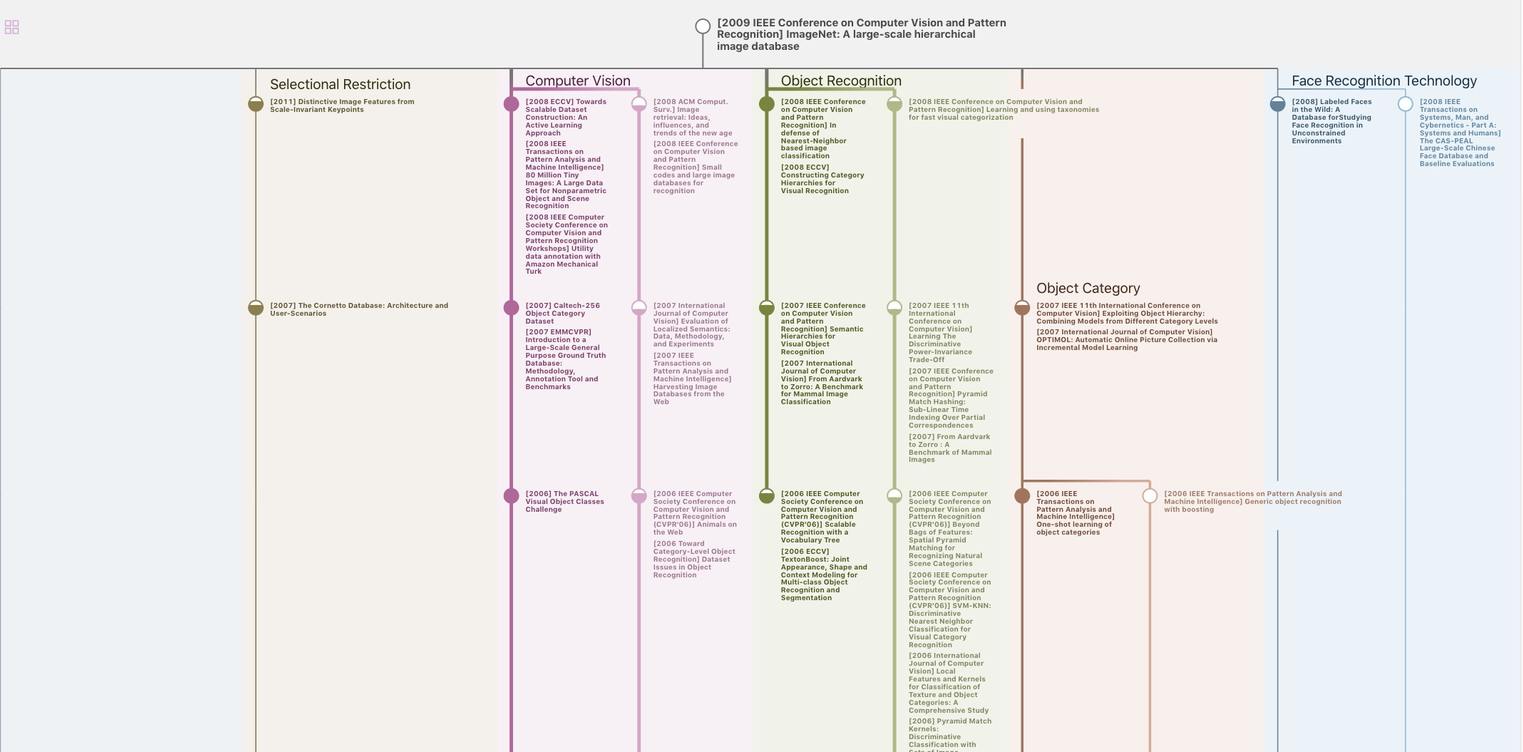
生成溯源树,研究论文发展脉络
Chat Paper
正在生成论文摘要