Related Work Our model can be viewed as a Deep Kalman Filter
semanticscholar(2019)
摘要
System identification of complex and nonlinear systems is a central problem for model predictive control and model-based reinforcement learning. Despite their complexity, such systems can often be approximated well by a set of linear dynamical systems if broken into appropriate subsequences. This mechanism not only helps us find good approximations of dynamics, but also gives us deeper insight into the underlying system. Leveraging Bayesian inference, Variational Autoencoders and Concrete relaxations, we show how to learn a richer and more meaningful state space, e.g. encoding joint constraints and collisions with walls in a maze, from partial and highdimensional observations. This representation translates into a gain of accuracy of learned dynamics showcased on various simulated tasks.
更多查看译文
AI 理解论文
溯源树
样例
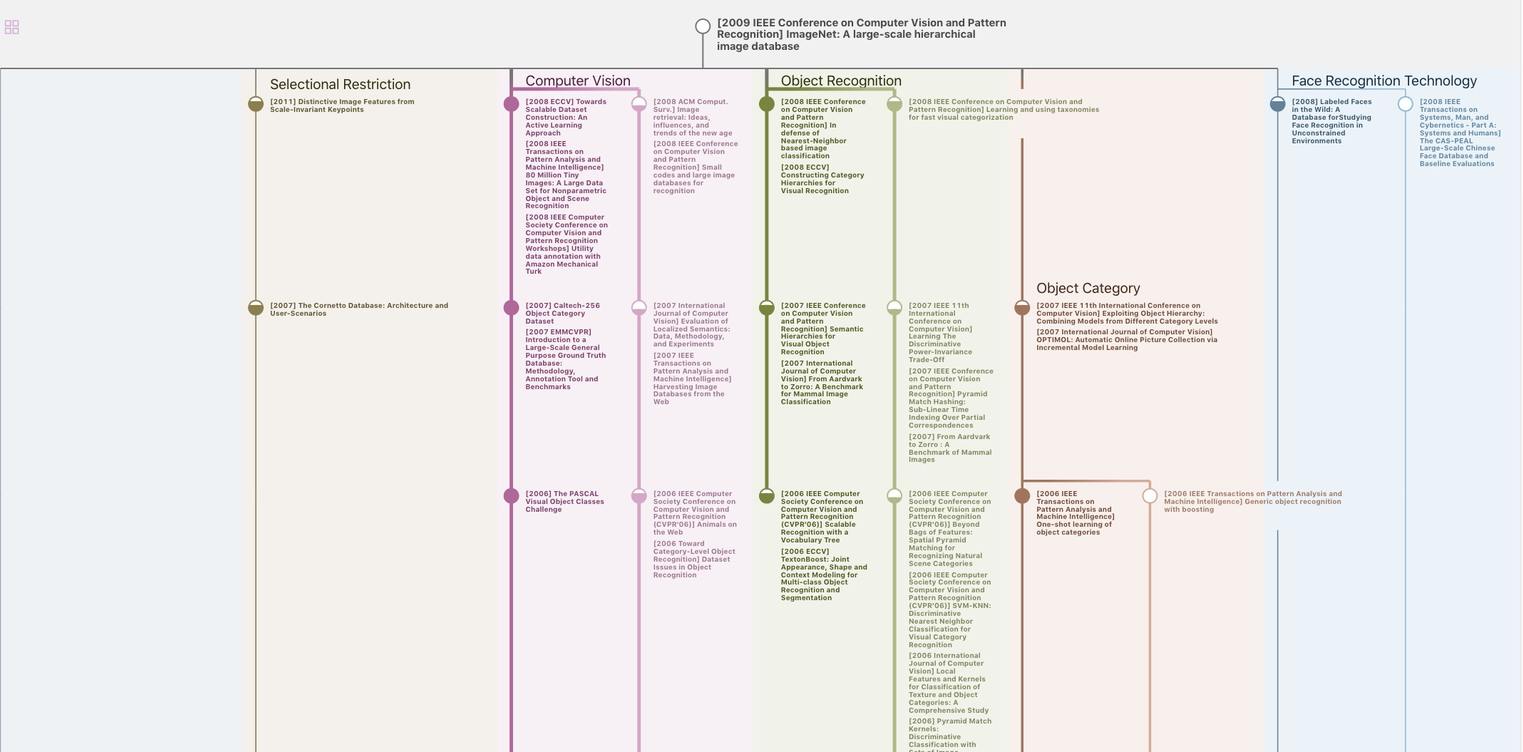
生成溯源树,研究论文发展脉络
Chat Paper
正在生成论文摘要