PFsuper: Simulation-Based Prognostics to Monitor and Predict Sparse Time Series
Annual Conference of the PHM Society(2017)
摘要
Commercial systems for predicting remaining useful life (RUL) of serviceable parts like engine oil tend to use either generic regression models (practical, e.g., widely deployed in the automotive industry), or dynamic models for which software lags behind theory (impractical, e.g., ‘one-trick’hardcodings). We describe an arguably more realistic framework using both generic and vehicle-specific dynamic models of time-series for simulation-based condition monitoring and RUL forecasting, suitable in situations where: (a) measured time-series are sparse or slowly sampled, and (b) health condition signals tend to follow relatively simple paths (low-degree polynomial stationary trends, unit-root stochastic trends, exponential growths, quasiperiodic oscillations). This combination unlocks affordability of PFsuper, a prognostics algorithm that implements online Bayesian learning with particle filters to jointly estimate hidden condition state and optionally a handful of unknown parameters, coupled withsubsimulations characterizing failure progression and RUL probability density function. The overall method converts a generic static time-as-a-regressor model into a stochastic differential equation, then has PFsuper adapt the initially generic model into a vehicle-specific one as datameasurements arrive.
更多查看译文
AI 理解论文
溯源树
样例
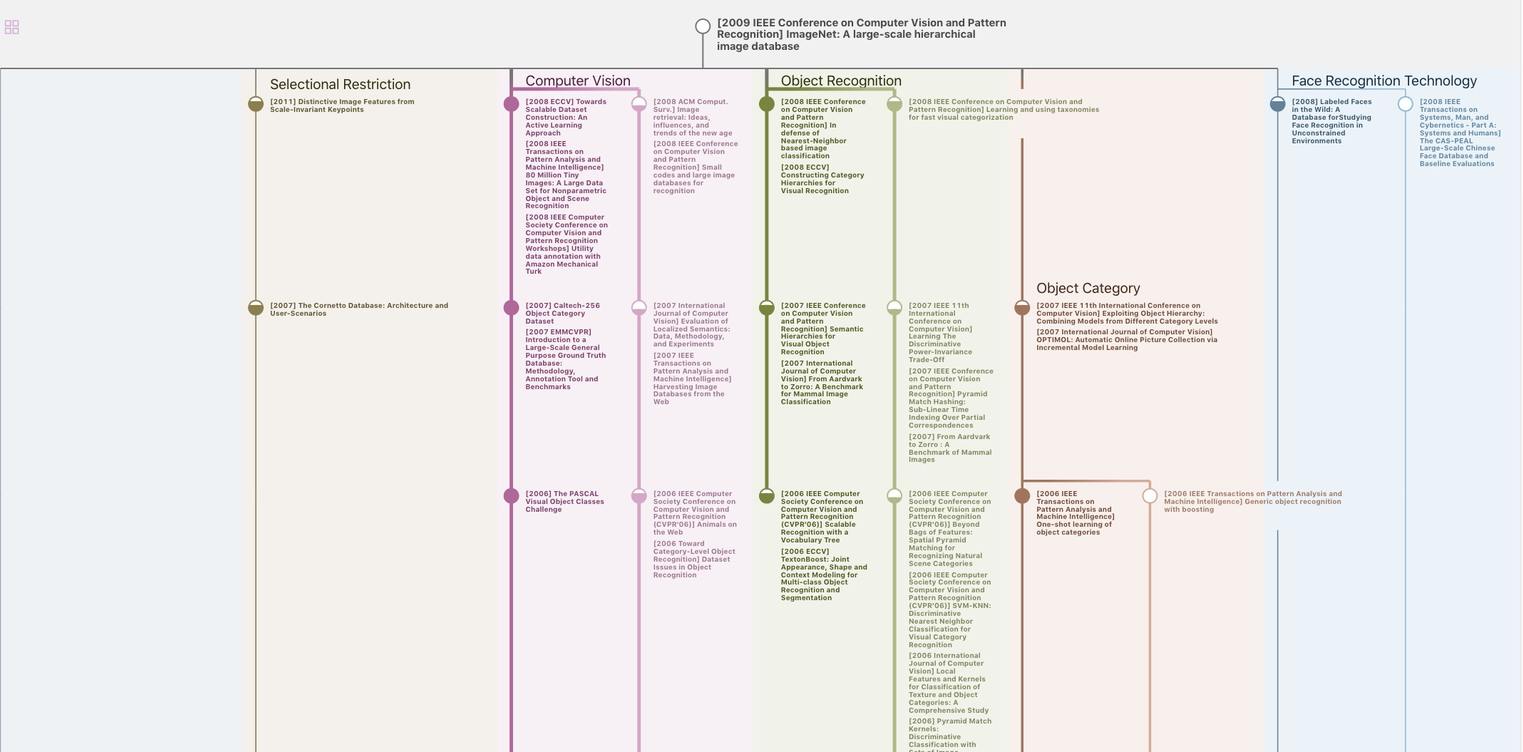
生成溯源树,研究论文发展脉络
Chat Paper
正在生成论文摘要