A Coordinate Descent Algorithm for Learning Compact Ranking Functions
semanticscholar(2010)
摘要
Algorithms for learning to rank can be inefficient when they use cost functions that involve more structure than the basic all-pair approach. To achieve efficient ranking, we consider the domination loss, which is designed to rank a small number of positive examples above a large number of negative ones, and extends to several layers of such relationships. In that context, we propose an efficient coordinate descent approach that scales linearly with the number of examples. We then present a number of extensions to the basic algorithm, including regularization, layers of examples, and feature induction. Experiments performed on several benchmark datasets show that the proposed approach yields significantly more compact models than existing algorithms do for similar performance.
更多查看译文
AI 理解论文
溯源树
样例
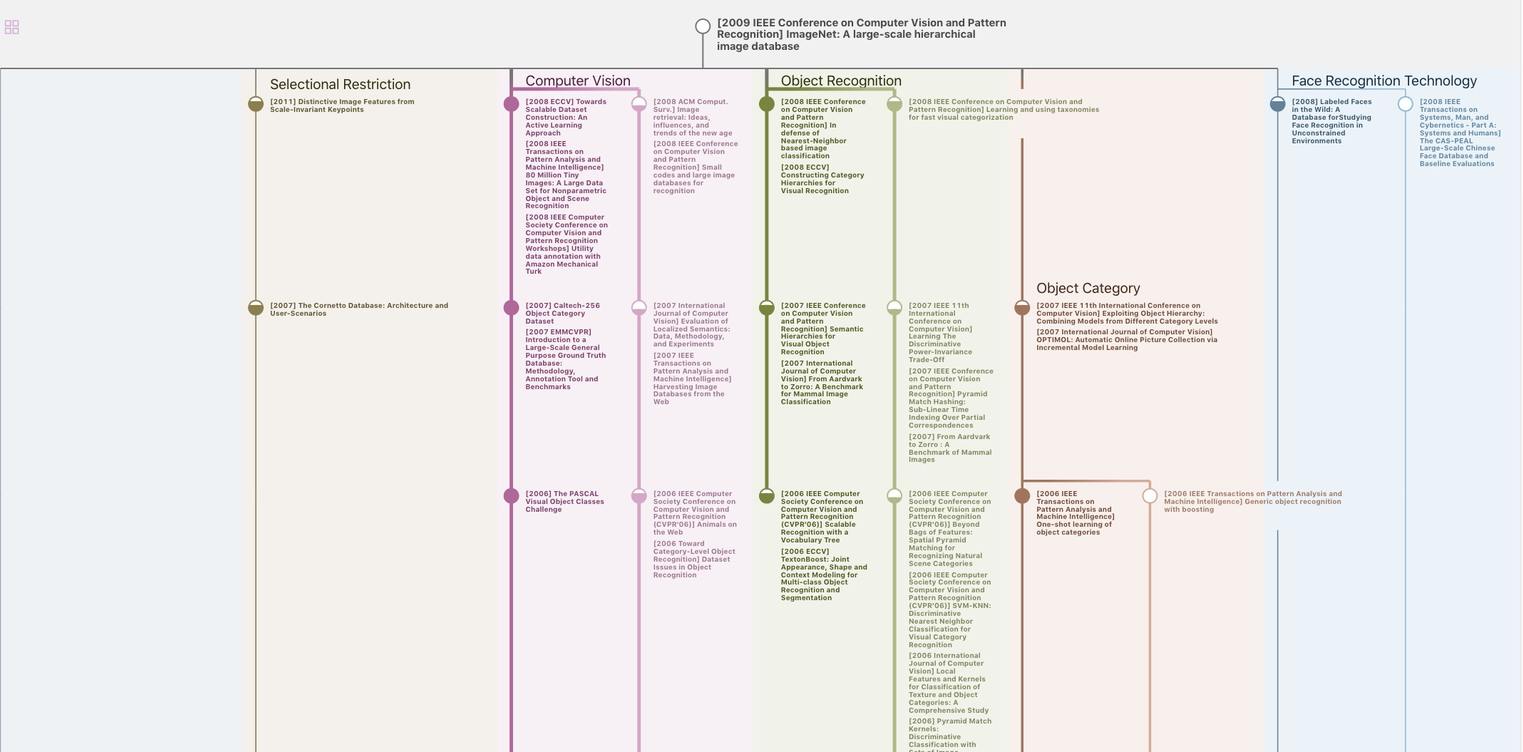
生成溯源树,研究论文发展脉络
Chat Paper
正在生成论文摘要